Artificial intelligence in breast reconstruction
Abstract
Breast reconstruction is a critical component of breast cancer treatment. With the rapid integration of Artificial Intelligence (AI) into healthcare, its potential to revolutionize breast reconstruction has become increasingly evident. This narrative review examines the latest AI developments across the preoperative, intraoperative, and postoperative phases of breast reconstruction. In preoperative consultations, AI and augmented reality (AR)-driven simulations help both the surgeons and the patients visualize reconstruction outcomes. Imaging analysis and predictive modeling enhance the precision and efficiency of autologous procedures such as deep inferior epigastric artery perforator flap-based reconstruction. Within the operating room, AI applications such as real-time perforator mapping and AR modeling offer plastic surgeons improved control and visualization, which helps to reduce postoperative complications. Furthermore, AI models help surgeons design and deliver more personalized and value-based postoperative care, thereby improving patient satisfaction and overall cost-effectiveness. While AI applications demonstrate promising utility, challenges such as high costs, reliability, and the need for extensive clinical validation remain. Ongoing research and large-scale clinical trials are crucial to fully harness AI’s potential in improving breast reconstruction outcomes.
Keywords
INTRODUCTION
Plastic surgery is a creative field at its core that is driven forward by innovation in both the techniques and devices used in aesthetic and reconstructive procedures. Breast reconstruction is a critical aspect of breast cancer treatment with significant physical and psychological benefits for patients[1]. Artificial intelligence (AI), which encompasses various fields such as machine learning and natural language processing, can drastically transform current breast reconstruction practices. Recently, many applications of AI, such as imaging analysis and symptom monitoring, are gradually becoming tools for plastic surgeons, both inside and outside of the operating room. As various AI technologies continue to emerge in the surgical realm, it is important to assess how these budding applications can be implemented in breast reconstruction to further improve patient outcomes. Here, we present a narrative review of the latest AI developments relating to the preoperative, intraoperative, and postoperative phases of breast reconstruction.
METHODS
This narrative review synthesizes key findings from the current literature on AI applications in breast reconstruction. A comprehensive literature search was conducted on PubMed and MEDLINE on August 1, 2024. Articles published on or prior to this date were included in the query. Keywords included MeSH terms “artificial intelligence”, “mammaplasty”, “surgery, plastic”, and “augmented reality”. Given the narrative nature of the review, no formal inclusion or exclusion criteria were applied. Articles were included if they offered specific discussions and presented novel findings on the use of AI, including machine learning (ML), Large Language Models (LLMs), and convolutional neural networks (CNNs) in breast reconstruction. Priority was given to original research, clinical trials, and articles with the most up-to-date information within the field. Articles that offered substantial insights or unique perspectives on advancements, limitations, and clinical applications were emphasized. The included articles were categorized into pre-, intra-, and postoperative applications. Recurring findings, including AR, AI-assisted perforator mapping, symptom monitoring, and patient satisfaction and outcome initiatives, were grouped and discussed.
AI IN PREOPERATIVE PLANNING
The gold standard for autologous breast reconstruction is the deep inferior epigastric artery perforator (DIEP) flap. Computed tomography angiography (CTA) is essential for plastic surgeons to understand the complex vascular architecture associated with the inferior epigastric artery and to select appropriate perforators for autologous breast reconstruction with DIEP flaps. However, manual interpretation of CTA by radiologists and plastic surgeons is labor-intensive and subject to variability.
As a promising alternative, AI has been deployed to automate CTA interpretation for DIEP flap planning. A recent study published by Mavioso et al. tested the feasibility of using semi-automated software to detect perforators for DIEP reconstruction. This AI application reduced the time spent on planning each case by approximately two hours[2]. While the software’s accuracy is lower for smaller vessels (< 1.5mm), it performs equally well for larger vessels compared to manual selection. Another recently published study by Lim et al. compared four LLMs in the interpretation of CTA scans[3]. Certain models, like Bing AI (Microsoft, Redmond, Washington, 2023), demonstrated superior accuracy and readability. However, as noted by the authors, the reliability of the output by these models remained a significant challenge, as they can sometimes provide irrelevant or “hallucinated” references. This limitation can detrimentally mislead surgeons, indicating the need for further development and testing of AI in CTA analysis.
Predictive analytics powered by ML models have emerged as crucial tools in preoperative risk assessment and management. In a study by O’Neill et al., a ML model was used to predict flap failure in microvascular breast reconstruction. It identified high-risk patient groups, such as those with obesity, comorbidities, and smoking habits, allowing for targeted interventions and improved outcomes[4]. With tools like this, surgeons can immediately stratify the risk of each patient and provide personalized counseling to high-risk patients on lifestyle modifications to prevent flap failure.
The Artificial Intelligence-Based Multimodal Risk Assessment Model for Surgical Site Infection (AMRAMS) exemplifies the potential of AI in risk management[5]. By incorporating demographics, preoperative lab results, and free-text preoperative notes, AMRAMS leveraged deep learning methods, including CNNs, to enhance accuracy. Compared with the National Nosocomial Infections Surveillance risk index manually scored by surgeons, AMRAMS offered significantly higher accuracy. The inclusion of semantic embeddings of preoperative notes further improves model performance, suggesting that AMRAMS could potentially replace traditional risk indices to provide highly personalized guidance for preoperative interventions.
AI-aided surgical planning is also transforming preoperative consultations. Traditional preoperative consultations often involve the use of diagrams, photographs, and verbal descriptions, which may not effectively render the potential outcomes of the surgery. In a study published by Chartier et al., BreastGAN, an AI tool driven by generative adversarial neural networks (GANs), was able to simulate breast augmentation outcomes based on preoperative images[6]. The use of another complementary technology in tandem with AI is particularly revolutionizing this space. Augmented reality (AR) overlays digital content onto the real world. When combined with AI that provides computational analysis and predictive capabilities, AR creates more immersive and comprehensive ways to tangibly visualize surgical outcomes, helping center patient expectations and improve postoperative satisfaction.
A prospective study with patients undergoing breast augmentation reported that patients were satisfied with preoperative 3D simulation using Arbrea Breast Software (Arbrea Labs, Zurich, Switzerland, 2018) and postoperative outcomes, measured with a visual satisfaction analog scale and BREAST-Q Augmentation module[7]. Arbrea, similar to BreastGAN, uses a type of generative AI called GANs in combination with AR and 3D simulation to help patients visualize surgical outcomes. Additionally, in another cohort of 40 patients undergoing breast reconstruction, virtual reality (VR) tools (3D imaging) and external sizers were shown to be the most effective among the five methods tested in choosing the implant volume[8]. Furthermore, the Crisalix portable 3D surface imagers, driven by deep learning, predicted breast volume with accuracy closely matching estimates from experienced plastic surgeons and actual intraoperative specimen weights[9].
Despite these exciting results, there are several barriers hindering the wider adoption of AI and AR in preoperative consultations. Users report that AR tools like the 3D HoloLens can be cumbersome and challenging to use[10]. In terms of breast volume prediction, discrepancies in accuracy may exist between different AI softwares, highlighting the need for additional testing and head-to-head comparison when choosing the application carefully[11]. Moreover, the cost of implementing AR technology for preoperative planning remains unclear, largely due to its scarce implementation thus far[12]. However, the cost of AR modalities that may implement AI is relatively fixed, such as the Microsoft 3D HoloLens, which retails for about $3,500; thus, their gradually decreasing cost per use may be beneficial for long-term implementation[13]. Effective utilization also requires specialized training for surgeons. Therefore, future studies should focus on addressing these limitations by exploring cost-effective solutions and developing standardized training protocols. Further, large-scale randomized controlled trials are necessary to validate the benefits of AR and establish its efficacy in different clinical settings.
AI APPLICATIONS INSIDE THE OPERATING ROOM
AI is revolutionizing the use of intraoperative imaging and visualization, particularly in autologous breast reconstruction, by generating AR models based on the data collected through advanced imaging technologies. These advanced imaging technologies include Near-Infrared Laser-Assisted Indocyanine Green (ICG) imaging, which has emerged as a reliable assessment tool of direct perforator perfusion zones. From raw ICG data, the SPY-Q Analysis Toolkit (Novadaq Technologies, Inc., Toronto, Canada, 2009) can calculate quantifiable parameters like relative and absolute perfusion values, so surgeons can objectively identify the dominant perforators and their perfusion zones. Sacks et al. demonstrated success in conventional free anterolateral thigh flap dissection with the aid of laser-assisted ICG, with only 1 flap loss in 15 due to venous congestion[14]. Ongoing efforts have shown early success in developing high-fidelity AI models using ICG fluorescence angiography to assist in flap trimming decisions intraoperatively. Singaravelu et al. developed an ensemble subspace k-nearest neighbor model that was able to predict the optimal excision area with 99.3% accuracy[15].
Building upon SPY-Q-guided manual identification, the integration of 3D-printed vascular modeling (3DVM) enables the visualization of more spatial information with depth, orientation, and direct tactile feedback in DIEP flap breast reconstructions[16]. Following these advancements in 3DVM, Meier et al. introduced a novel approach using projected AR for perforator mapping in DIEP flap breast reconstruction with the aim of increasing interactive visualization of vascular anatomy[17]. The study utilized a self-aligning projection device equipped with a thermal camera, which projected a color-coded thermal map directly onto the patient’s abdomen. This system employed dynamic infrared thermography (DIRT) to identify perforators based on their heat signatures, offering a quick and non-invasive method for perforator mapping. While the technology is still nascent, early results are promising, with the majority of
Recent advancements in AI have led to the development of a novel approach using DIRT coupled with CNNs for automated blood vessel detection in DIEP flap breast reconstruction[2]. These deep learning models can extract complex features from medical images, capturing both local and global context to enhance the accuracy of perforator identification and flap perfusion assessment[18].
The study by De La Hoz et al. utilized a DIRT protocol to capture images of the abdominal area after cooling[19], generating a dataset that was used to train and test the CNN. The CNN was designed to directly segment these thermograms to identify perforators with high confidence. Saxena et al. further advanced the field by exploring deep learning for vessel segmentation in DIEP flap planning[20]. Their approach used rectus abdominis muscle segmentation from computed tomography (CT) scans to select the appropriate region of interest for targeted vessel segmentation and diameter measurement. However, the model’s reliance on synthetic datasets requires further validation.
A separate study by Seth et al. segmented CTA data using AI-enabled software, Materialise Mimics (Materialise NV, Belgium, 2017)[21]. Materialise Mimics can provide automated segmentation of anatomical structures on CTA using proprietary AI that involves image thresholding, ML, deep learning, and graph learning algorithms applied to computer vision. This study created a virtual overlay of 3D models containing color-coded representations of patient anatomy, subsequently manually aligned with the patient using fixed anatomical landmarks[22]. This allowed for the visualization of DIEP’s extra- and intramuscular routes directly on the patient's abdomen, effectively remedying the limitations with perforator depth and its respective course changes mentioned by DeFazio et al.[16]. While the sample size was limited to 5 cases, preoperative perforator route identification was successful for all patients, as verified by Doppler. Map alignment time may prove to be prohibitive to large-scale implementation, but other studies using coordinate systems centered around the umbilicus have shown high accuracy without the same tedious alignment process[23].
The economic considerations of implementing 3D modeling as an intraoperative aid vary significantly between physical and virtual approaches. Ghasroddashti et al. reported that the cost of printing each 3DVM differed significantly, ranging from $6.50 to $829.72[24]. While plastic and reconstructive surgery lacks formal cost-benefit analyses specific to the field, insights can be drawn from other surgical specialties. Ravi et al. and Ballard et al. have demonstrated that within the field of general surgery, orthopedic, and maxillofacial surgery, the reduced operative times do generally outweigh the model production costs[25,26]. In contrast, virtual 3D modeling approaches, particularly those utilizing DIRT technology, show promising cost advantages. The growing adoption of DIRT can be attributed to its operational simplicity and potential for further cost reduction through integration with smartphone-based cameras, though comprehensive cost analyses for virtual modeling implementations remain limited[27].
AI TOOLS TO ENHANCE POSTOPERATIVE OUTCOMES
Symptom monitoring and postoperative recovery
AI is rapidly revolutionizing breast reconstruction procedures by transitioning postoperative care from traditional in-person flap checks and clinical visits to portable and ambient tools, providing closer monitoring, quicker responses, and improved surgical outcomes. One such application is the use of smartphone-based tools for managing and monitoring microvascular flaps[28]. Supervised ML was able to predict vascular compromise in free flaps with a high accuracy of 98.4% using images taken on a smartphone and clinical variables[29]. In the study by Hsu et al., a deep learning model integrated smartphone application was used for free flap monitoring, which demonstrated high accuracy (95.3%), sensitivity (95.2%), and specificity (95.3%) in predicting venous congestion in free flaps, with an area under the curve being 0.99[30]. More importantly, in several cases, the smartphone application was able to detect an increase in congestion probability on average two hours earlier than human observers. Another study by Kim et al. similarly harnessed the power of CNNs and the convenience of smartphone cameras for free flap monitoring[31]. Their best model detected venous insufficiency and arterial insufficiency with sensitivity of 97.5% and 92.8%, respectively. Pilot implementation of a smartphone application equipped with the same AI algorithms on 10 patients demonstrated great utility in the clinical setting, with prompt feedback in less than one second. In autologous breast reconstruction, the time at which potential flap compromise is detected may determine what surgical or medical interventions are possible, and the likelihood of those treatments working to salvage a flap from progressing to failure. The current practice of detecting free flap failure primarily involves frequent clinical examination of the flap combined with Doppler ultrasound to monitor blood flow. This process requires specially trained medical professionals and needs to be done in the medical setting, which is laborious and resource-intensive. Digital monitoring tools, especially when equipped with predictive ML algorithms to identify early risk factors, could provide cost-effective, real-time, and non-invasive monitoring. These emerging applications of AI will not only allow for prompt intervention by plastic surgeons to improve flap survival, but also enhance patient outcomes and optimize resource allocation within the healthcare system.
While smartphone-based tools and ML show promising clinical utility, comprehensive cost analysis specific to those technologies when used in postoperative flap monitoring is limited. Lee et al. noted a significant cost reduction due to a drastic decrease in the staffing burden required for frequent flap checks, especially within the first 24 h post-operation[32].
Predictive analytics can also be utilized to create personalized postoperative care plans and enhance patient-specific, value-based care and recovery[33]. ML models based on preoperative and intraoperative information can help plastic surgeons quickly stratify risk factors of flap failure in microvascular breast reconstruction and guide postoperative treatment planning[34]. For example, ML models have been developed to predict
Other AI applications that are revolutionizing postoperative care include remote monitoring and telemedicine. Wearable AI devices have been applied in aesthetic postoperative settings to monitor patient compliance with positioning after discharge[42]. This could be used to detect clinical derangements and alert surgeons in real time, enabling prompt response and better outcomes, while allowing patients to recover from the comfort of their own homes.
Patient satisfaction and outcomes initiatives
Patient satisfaction with breast reconstruction following mastectomy is highly variable and subject to influence from various factors involved in their cancer treatment process. ML models developed from an international cohort of patients diagnosed with breast cancer who underwent mastectomy and reconstruction demonstrated the ability to accurately predict individual patient-reported outcome measures (PROMs), as evaluated by BREAST-Q, prior to the initiation of the reconstruction process[43]. By integrating these AI models into treatment planning for each patient, plastic surgeons may better guide the patients in making treatment plans that maximize patient satisfaction.
AI tools such as chatbots and virtual assistants can significantly enhance the patient experience with consultation and education by promptly addressing patients’ queries. For real-time patient question resolution, a survey from a single institution demonstrated that 96% of patients were satisfied or somewhat satisfied with their interaction with the patient-facing AI chatbots[44]. Regarding general simplification of patient instructions and educational materials, Chat Generative Pretrained Transformer 3.5 (OpenAI, San Francisco, California, 2022) could effectively simplify these resources down to a broadly acceptable reading level[45]. This is especially relevant for patients considering implant-based reconstruction, who often face lengthy and evolving educational materials about implant safety and risks, as the Food and Drug Administration (FDA) continues to update its recommendations and warnings surrounding breast implants[46]. For example, AI-driven tools can help break down lengthy patient materials regarding the safety profile and risks associated with these implants into more digestible formats, which will help patients make informed decisions.
Moreover, patients undergoing breast reconstruction, especially those from racial minority groups, report significant dissatisfaction with the information provided to them regarding preoperative decision making and postoperative recovery[47]. They often perceive significant gaps in their knowledge and understanding of expected outcomes, leading to increased anxiety and fear during the surgical process. Patients are increasingly turning to online resources for advice and insights prior to a potential surgical journey, often because of the stress and frustration of navigating the complex or inaccessible materials provided by their surgical teams. Yet, even as the available number of existing resources targeted toward breast reconstruction continues to grow, most are not written at a broadly accessible level[48]. This calls for the need to improve this process, which will not only elevate immediate patient experience, but may also lead to overall increased patient satisfaction with their breast reconstruction. AI chatbots in their current state have shown the ability to perform such tasks effectively and could be easily integrated to bridge this gap with almost immediate effect[49]. Notably, a concern in validating AI software is that it is limited by the biases of the datasets on which it is built, including those disproportionately affecting populations that are poorly represented even in large clinical trials. With even high-level evidence succumbing to this weakness[50,51], it is important that clinically facing tools are continuously refined to ensure high-quality care.
CONCLUSION
The integration of AI in breast reconstruction holds great promise for improving surgical outcomes and patient satisfaction. AI-driven image analysis, predictive modeling, and personalized planning tools enhance the precision and efficiency of surgical planning. Additionally, VR and AR technologies provide innovative ways to educate and engage patients, helping them make informed decisions about their care. As AI technologies continue to evolve, their applications in breast reconstruction are likely to expand, offering even greater benefits to patients and surgeons alike. Overcoming current limitations, including costs, accuracy, and reliability, and further evaluating these applications through prospective, randomized, and large-scale clinical trials will be necessary to leverage AI’s full potential to improve surgical care and patient outcomes in breast reconstruction.
DECLARATIONS
Authors’ contributions
Conceptualization, drafting and editing of the manuscript, technical support, administrative support: Shen Y, Malek AJ
Drafting and editing of the manuscript, technical support: Gao R
Conceptualization, editing of the manuscript, administrative support: Broyles JM
Availability of data and materials
Not applicable.
Financial support and sponsorship
None.
Conflicts of interest
Broyles JM serves on the scientific advisory board for Healshape LLC, receives consulting support from The Agency for Healthcare Research and Quality, and serves on the Medical Device Advisory Board for the Food and Drug Administration. The other authors declared that there are no conflicts of interest.
Ethical approval and consent to participate
Not applicable.
Consent for publication
Not applicable.
Copyright
© The Author(s) 2025.
REFERENCES
1. Roy N, Downes MH, Ibelli T, et al. The psychological impacts of post-mastectomy breast reconstruction: a systematic review. Ann Breast Surg. 2024;8:19-19.
2. Mavioso C, Araújo RJ, Oliveira HP, et al. Automatic detection of perforators for microsurgical reconstruction. Breast. 2020;50:19-24.
3. Lim B, Cevik J, Seth I, et al. Evaluating artificial intelligence's role in teaching the reporting and interpretation of computed tomographic angiography for preoperative planning of the deep inferior epigastric artery perforator flap. JPRAS Open. 2024;40:273-85.
4. O'Neill AC, Yang D, Roy M, Sebastiampillai S, Hofer SOP, Xu W. Development and evaluation of a machine learning prediction model for flap failure in microvascular breast reconstruction. Ann Surg Oncol. 2020;27:3466-75.
5. Chen W, Lu Z, You L, Zhou L, Xu J, Chen K. Artificial intelligence-based multimodal risk assessment model for surgical site infection (AMRAMS): development and validation study. JMIR Med Inform. 2020;8:e18186.
6. Chartier C, Watt A, Lin O, Chandawarkar A, Lee J, Hall-Findlay E. BreastGAN: artificial intelligence-enabled breast augmentation simulation. Aesthet Surg J Open Forum. 2022;4:ojab052.
7. La Padula S, Pensato R, D'Andrea F, et al. Assessment of patient satisfaction using a new augmented reality simulation software for breast augmentation: a prospective study. J Clin Med. 2022;11:3464.
8. Hammond DC, Kim K, Bageris MH, Chaudhry A. Use of three-dimensional imaging to assess the effectiveness of volume as a critical variable in breast implant selection. Plast Reconstr Surg. 2022;149:70-9.
9. Kwong JW, Tijerina JD, Choi S, et al. Assessing the accuracy of a 3-dimensional surface imaging system in breast volume estimation. Ann Plast Surg. 2020;84:S311-7.
10. Amini S, Kersten-Oertel M. Augmented reality mastectomy surgical planning prototype using the HoloLens template for healthcare technology letters. Healthc Technol Lett. 2019;6:261-5.
11. Stern CS, Plotsker EL, Rubenstein R, et al. Three-dimensional surface analysis for preoperative prediction of breast volume: a validation study. Plast Reconstr Surg. 2023;152:1153-62.
12. Vles MD, Terng NCO, Zijlstra K, Mureau MAM, Corten EML. Virtual and augmented reality for preoperative planning in plastic surgical procedures: a systematic review. J Plast Reconstr Aesthet Surg. 2020;73:1951-9.
13. Shafarenko MS, Catapano J, Hofer SOP, Murphy BD. The role of augmented reality in the next phase of surgical education. Plast Reconstr Surg Glob Open. 2022;10:e4656.
14. Sacks JM, Nguyen AT, Broyles JM, Yu P, Valerio IL, Baumann DP. Near-infrared laser-assisted indocyanine green imaging for optimizing the design of the anterolateral thigh flap. Eplasty. 2012;12:e30.
15. Singaravelu A, Dalli J, Potter S, Cahill RA. Artificial intelligence for optimum tissue excision with indocyanine green fluorescence angiography for flap reconstructions: proof of concept. JPRAS Open. 2024;41:389-93.
16. DeFazio MV, Arribas EM, Ahmad FI, et al. Application of three-dimensional printed vascular modeling as a perioperative guide to perforator mapping and pedicle dissection during abdominal flap harvest for breast reconstruction. J Reconstr Microsurg. 2020;36:325-38.
17. Meier EL, Ulrich DJO, Hummelink S. Projected augmented reality in DIEP flap breast reconstruction: projecting perforators on the skin using dynamic infrared thermography. J Plast Reconstr Aesthet Surg. 2024;94:83-90.
18. Pinto-Coelho L. How artificial intelligence is shaping medical imaging technology: a survey of innovations and applications. Bioengineering. 2023;10:1435.
19. De La Hoz EC, Verstockt J, Verspeek S, et al. Automated thermographic detection of blood vessels for DIEP flap reconstructive surgery. Int J Comput Assist Radiol Surg. 2024;19:1733-41.
21. AI-enabled segmentation | materialise mimics. Available from: https://www.materialise.com/en/healthcare/mimics-innovation-suite/ai-enabled-segmentation. [Last accessed on 30 Dec 2024].
22. Seth I, Lindhardt J, Jakobsen A, et al. Improving visualization of intramuscular perforator course: augmented reality headsets for DIEP flap breast reconstruction. Plast Reconstr Surg Glob Open. 2023;11:e5282.
23. Necker FN, Cholok DJ, Fischer MJ, et al. HoloDIEP-faster and more accurate intraoperative DIEA perforator mapping using a novel mixed reality tool. J Reconstr Microsurg. 2024; doi: 10.1055/s-0044-1788548.
24. Ghasroddashti A, Guyn C, Martou G, Edmunds RW. Utility of 3D-printed vascular modeling in microsurgical breast reconstruction: a systematic review. J Plast Reconstr Aesthet Surg. 2024;96:95-104.
25. Ballard DH, Mills P, Duszak R Jr, Weisman JA, Rybicki FJ, Woodard PK. Medical 3D printing cost-savings in orthopedic and maxillofacial surgery: cost analysis of operating room time saved with 3D printed anatomic models and surgical guides. Acad Radiol. 2020;27:1103-13.
26. Ravi P, Burch MB, Farahani S, et al. University of Cincinnati 3D Printing Clinical Service Participants. Utility and costs during the initial year of 3D printing in an academic hospital. J Am Coll Radiol. 2023;20:193-204.
27. Cifuentes IJ, Dagnino BL, Salisbury MC, Perez ME, Ortega C, Maldonado D. Augmented reality and dynamic infrared thermography for perforator mapping in the anterolateral thigh. Arch Plast Surg. 2018;45:284-8.
28. Hudson T, Hogue E, Mullner D, Herrera F, Scomacao I. The utility of smartphone-based thermal imaging in the management and monitoring of microvascular flap procedures: a systematic review and meta-analysis. Ann Plast Surg. 2023;90:S420-5.
29. Huang RW, Tsai TY, Hsieh YH, et al. Reliability of postoperative free flap monitoring with a novel prediction model based on supervised machine learning. Plast Reconstr Surg. 2023;152:943e-52e.
30. Hsu SY, Chen LW, Huang RW, et al. Quantization of extraoral free flap monitoring for venous congestion with deep learning integrated iOS applications on smartphones: a diagnostic study. Int J Surg. 2023;109:1584-93.
31. Kim J, Lee SM, Kim DE, et al. Development of an automated free flap monitoring system based on artificial intelligence. JAMA Netw Open. 2024;7:e2424299.
32. Lee C, Chen C, Wang H, Chen L, Perng C. Utilizing mask RCNN for monitoring postoperative free flap: circulatory compromise detection based on visible-light and infrared images. IEEE Access. 2022;10:109510-25.
33. Ramkumar PN, Haeberle HS, Bloomfield MR, et al. Artificial intelligence and arthroplasty at a single institution: real-world applications of machine learning to big data, value-based care, mobile health, and remote patient monitoring. J Arthroplasty. 2019;34:2204-9.
34. Shi YC, Li J, Li SJ, et al. Flap failure prediction in microvascular tissue reconstruction using machine learning algorithms. World J Clin Cases. 2022;10:3729-38.
35. Wang SY, Barrette LX, Ng JJ, et al. Predicting reoperation and readmission for head and neck free flap patients using machine learning. Head Neck. 2024;46:1999-2009.
36. Hassan AM, Biaggi-Ondina A, Asaad M, et al. Artificial intelligence modeling to predict periprosthetic infection and explantation following implant-based reconstruction. Plast Reconstr Surg. 2023;152:929-38.
37. Braun SE, Sinik LM, Meyer AM, Larson KE, Butterworth JA. Predicting complications in breast reconstruction: development and prospective validation of a machine learning model. Ann Plast Surg. 2023;91:282-6.
38. Plastic surgery statistics. American society of plastic surgeons. Available from: https://www.plasticsurgery.org/plastic-surgery-statistics. [Last accessed on 30 Dec 2024].
39. Masoomi H, Hanson SE, Clemens MW, Mericli AF. Autologous breast reconstruction trends in the United States: using the nationwide inpatient sample database. Ann Plast Surg. 2021;87:242-7.
40. Fracol M, Teven CM, Selimos B, et al. Pushing the DIEP envelope with ERAS: 24 hour discharge is safe in appropriately selected patients. Plast Reconstr Surg Glob Open. 2023;11:e5070.
41. Sauro KM, Smith C, Ibadin S, et al. Enhanced recovery after surgery guidelines and hospital length of stay, readmission, complications, and mortality: a meta-analysis of randomized clinical trials. JAMA Netw Open. 2024;7:e2417310.
42. Vecchio D, Stein MJ, Dayan E, Marte J, Theodorou S. Nanotechnology and artificial intelligence: an emerging paradigm for postoperative patient care. Aesthet Surg J. 2023;43:748-57.
43. Pfob A, Mehrara BJ, Nelson JA, Wilkins EG, Pusic AL, Sidey-Gibbons C. Machine learning to predict individual patient-reported outcomes at 2-year follow-up for women undergoing cancer-related mastectomy and breast reconstruction (INSPiRED-001). Breast. 2021;60:111-22.
44. Davis L. Northwell releases AI-driven pregnancy chatbot | Northwell Health. January 11, 2023. Available from: https://www.northwell.edu/news/the-latest/northwell-releases-ai-driven-pregnancy-chatbot. [Last accessed on 30 Dec 2024].
45. Vallurupalli M, Shah ND, Vyas RM. Optimizing readability of patient-facing hand surgery education materials using Chat Generative Pretrained Transformer (ChatGPT) 3.5. J Hand Surg Am. 2024;49:986-91.
46. FDA news release. FDA strengthens safety requirements and updates study results for breast implants. FDA. October 28, 2021. Available from: https://www.fda.gov/news-events/press-announcements/fda-strengthens-safety-requirements-and-updates-study-results-breast-implants. [Last accessed on 30 Dec 2024].
47. Cohen WA, Ballard TN, Hamill JB, et al. Understanding and optimizing the patient experience in breast reconstruction. Ann Plast Surg. 2016;77:237-41.
48. Vargas CR, Koolen PGL, Chuang DJ, Ganor O, Lee BT. Online patient resources for breast reconstruction: an analysis of readability. Plast Reconstr Surg. 2014;134:406-13.
49. Farid Y, Fernando Botero Gutierrez L, Ortiz S, et al. Artificial intelligence in plastic surgery: insights from plastic surgeons, education integration, ChatGPT's survey predictions, and the path forward. Plast Reconstr Surg Glob Open. 2024;12:e5515.
50. Jüni P, Altman DG, Egger M. Systematic reviews in health care: assessing the quality of controlled clinical trials. BMJ. 2001;323:42-6.
Cite This Article

How to Cite
Download Citation
Export Citation File:
Type of Import
Tips on Downloading Citation
Citation Manager File Format
Type of Import
Direct Import: When the Direct Import option is selected (the default state), a dialogue box will give you the option to Save or Open the downloaded citation data. Choosing Open will either launch your citation manager or give you a choice of applications with which to use the metadata. The Save option saves the file locally for later use.
Indirect Import: When the Indirect Import option is selected, the metadata is displayed and may be copied and pasted as needed.
About This Article
Special Issue
Copyright
Data & Comments
Data
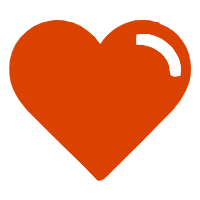
Comments
Comments must be written in English. Spam, offensive content, impersonation, and private information will not be permitted. If any comment is reported and identified as inappropriate content by OAE staff, the comment will be removed without notice. If you have any queries or need any help, please contact us at [email protected].