Clinical deployment of machine learning models in craniofacial surgery: considerations for adoption and implementation
Abstract
The volume and complexity of clinical data are growing rapidly. The potential for artificial intelligence (AI) and machine learning (ML) to significantly impact plastic and craniofacial surgery is immense. This manuscript reviews the overall landscape of AI in craniofacial surgery, highlighting the scarcity of prospective and clinically translated models. It examines the numerous clinical promises and challenges associated with AI, such as the lack of robust legislation and structured frameworks for its integration into medicine. Clinical translation considerations are discussed, including the importance of ensuring clinical utility for real-world use. Finally, this commentary brings forward how clinicians can build trust and sustainability toward model-driven clinical care.
Keywords
CLINICAL DEPLOYMENT OF MACHINE LEARNING MODELS IN CRANIOFACIAL SURGERY: CONSIDERATIONS FOR ADOPTION AND IMPLEMENTATION
The volume and complexity of clinical data are growing rapidly across all fields of medicine. In parallel, computational power is expanding and becoming more accessible, while human resources continue to remain relatively stagnant and limited in healthcare[1]. It has been predicted that artificial intelligence (AI) and machine learning (ML) will be ubiquitous in future clinical care[1]. Significant areas of interest that could revolutionize care include processing of electronic health record data, image classification, and identification of medical errors[2,3]. The promising appeal to incorporate AI into clinical practice must be contextualized, and the intrinsic limitations to the use of ML algorithms acknowledged. Clinical translation of ML tools and prospective validation articles are scarce to date and a new set of considerations for adoption and implementation have been unveiled. This article reviews the current ML landscape in craniofacial surgery and highlights promises, challenges, and considerations for successful clinical translation.
Current landscape of ML in craniofacial surgery
The role of ML and AI in craniofacial surgery has previously been thoroughly reviewed[4-9]. In a scoping review by Mak et al. (2020), the authors identified numerous craniofacial-based studies developing ML models[4]. ML is particularly relevant to craniofacial surgery as it is a specialty that: (1) relies on imaging for diagnostic purposes; (2) uses standardized and universal anatomical landmarks (soft tissue and bony); (3) benefits from three-dimensional planning and surgical navigation; and (4) has variable outcomes and benefits from risk prediction[9]. Thus far, published craniofacial surgery studies using AI have been experimental and theoretical in nature, mostly relying on retrospective datasets with very limited sample sizes and generally single-centered.
Cleft surgery
The potential benefit of integrating AI into cleft care spans numerous facets of clinical care due to the varied presentations (from cleft lip to velopharyngeal insufficiency and dentoalveolar discrepancies) and evolution over patients’ growth and development. A scoping review identified previously explored areas for implementation of AI in cleft care[10]: prediction of risk of developing cleft lip or palate, diagnosis (prenatal cleft presence), severity of morphological deformities of nose[11], speech evaluation (presence of hypernasality, assessment of intelligibility)[12], surgical planning (estimation of volumetric defect of alveolar cleft), prediction of need for orthognathic surgery, and more.
Orthognathic surgery
Orthognathic surgery lands itself well to be augmented by AI, although few studies have been published thus far. A review of possible applications demonstrated areas of interest for future studies, including[13]: complex diagnoses (superimposing numerous diagnostic tools for measurement of upper airways and management of obstructive sleep apnea), common diagnoses (lateral cephalogram review), treatment planning (taking into account the symbiotic relationship with orthodontic changes), creation of custom dental appliances, and much more. An early study (2019) within the field of orthognathic surgery proposed a proof of concept that AI (specifically convolutional neural networks) can be used in order to score facial attractiveness and apparent age in orthognathic surgery patients[14]. Assistance in diagnosis and surgical planning has also been proposed using CT scan images and comparing discrepancies in cephalometric measures between the AI-generated plan and the postoperative images[15].
Craniosynostosis and head shape difference
Head shape differences are ubiquitous, and the rarity of craniosynostosis and the clinical ramifications of later diagnosis generate huge diagnostic importance, which can be aided by AI. A review from 2023 explored the existing literature on the topic, highlighting that most studies thus far have used two-dimensional photographs for model building in which image orientation had a large impact on outcome[16]. A team at the Hospital for Sick Children, Toronto, is also developing a model compatible with three-dimensional photographic analysis for diagnostic purposes and is validating it into a mobile capture for widespread use and patient empowerment[17].
Craniofacial trauma
The detection of facial trauma on imaging has been an area of interest for the introduction of AI[18]. Overall performance of models being developed, such as the DeepCT, has been excellent with high reported sensitivity (89%) and specificity (95%) while using two-dimensional models with CT scan images[19]. Detection of facial fractures using three-dimensional images is also being explored within the biotechnology literature[20]. Various models have been used, including CNNs and deep learning systems using a one-stage detection called you only look once (YOLO)[18,21,22].
Facial reanimation
Thus far, AI represents a great promise of revolutionizing outcomes assessment for facial reanimation. Traditionally, researchers and surgeons have used static images of smile commissure excursion using various tools to provide an outcome assessment[23]. With the use of ML technology, facial expression tracking and, therefore, dynamic facial assessment are becoming possible. Video analysis of cross-facial nerve graft (CFNG) and free gracilis muscle patients took place in a proof of concept study by Boonipat et al.[24]. This methodology enabled the authors to analyze symmetry, excursion, and overall facial movements via the review of more than 500 facial landmarks. Another aspect of facial reanimation surgery that has remained difficult to capture with existing outcome measures includes smile spontaneity. Dusseldorp et al. compared controls with patients having undergone CFNG, masseter nerve coaptation, and dual innervation to assess the feasibility of using such a tool to review spontaneity[25]. Although promising innovative outcomes analyses are being developed, the surgical reconstruction of facial palsy focuses on the lower face and smile, whereas AI technology considers the face as a whole.
Clinical promises
To many clinicians, AI represents a new technological advancement that can revolutionize their practice, yet also a poorly understood and intimidating one. Such paradoxical perceptions of AI can be explained by the power that is inherent to it and the often lack of transparency behind its use on our electronic devices, social media, and more. Transparency, safety, and methodological rigor are central to evidence-based medicine and can be enabled with established reporting standards tools. A new initiative, the MINimum Information for Medical AI Reporting (MINIMAR), proposes such reporting standards[2]. MINIMAR highlights four fundamental areas of transparency and reporting: (1) study population and setting; (2) patient demographic characteristics; (3) model architecture; and (4) model evaluation. This concept is further explored by research done by Sendak et al. with the “Model Facts” label[26]. To maximize the effective and positive implementation of an AI model, adherence to such standards of reporting is highly recommended.
Some of the highly anticipated promises of AI are centered around the optimization of patient-centered care and outcomes. The hope is that detailed and precise ML algorithms can help enhance a clinician’s diagnostic, management, and prognostic capabilities, as well as monitor and decrease medical errors. AI may also allow patients to have a sense of improved ownership of their data and empower them with the ability to interpret their health information[1]. Such optimization of care would have a system-level impact and could allow for more efficient workflow, better resource allocation, and improved clinical outcomes, to name a few[1].
Clinical challenges
Some of the most feared consequences of AI are the potential for patient harm and the increased accountability for clinicians. At baseline, introducing new technology into the medical field requires close monitoring and auditing. In the case of AI, a flawed algorithm can lead to the dissemination of iatrogenic harm, medical errors, and malpractice[1]. Incorrect model outputs leading to adverse patient outcomes also increase the potential liability for physicians. This can deter healthcare professionals from utilizing AI as it is currently not essential to the delivery of care. Beyond an inaccurate algorithm also exists a black box one, or one for which the operations and output cannot be explained and is at the center of much controversy[27,28]. In the current climate, careful model validation, prospective auditing, and algorithm enhancements are therefore necessary, as well as human support and oversight upon deployment of any AI models[1]. Transparency regarding model development and data sources used, algorithms and overall methodological disclosure according to the MINIMAR reporting guidelines can mitigate some of these challenges[2].
The Gender Shades project further underscores the importance of reporting guidelines to ensure rigorous bias assessments are completed[29]. The study highlights significant racial and gender bias in commercial facial recognition technologies. Their research revealed that AI systems from companies like IBM, Microsoft, and Face++ were less accurate at identifying gender in darker-skinned individuals, particularly women, with error rates of up to 34.7% for darker-skinned females, compared to less than 1% for lighter-skinned males. This disparity is linked to the underrepresentation of diverse phenotypes in the datasets used to train these models, which overwhelmingly consist of lighter-skinned individuals.
These findings are particularly relevant for photo-based AI applications in craniofacial surgery. As these technologies become integrated into surgical planning and diagnostics, biases could disproportionately affect individuals with darker skin tones, potentially leading to misdiagnoses or improper treatment recommendations. It underscores the necessity of using diverse and balanced datasets in the development of AI models in craniofacial surgery as well as conducting detailed subgroup bias assessments on gender, age, race, ethnicity, and in some instances, skin tone (for image/photography-based applications). Of note, transparency to patients on how a model is expected to work specifically for them, based on these subgroup bias assessments, is important to facilitate genuine informed consent. It is expected that variations in performance will occur, and with transparency in understanding the limitations of a model, risk can be mitigated to ensure care delivery remains equitable even when a model may not perform equally across various populations.
While large data pools are required to create reliable and generalizable ML models, the acquisition of such information may lead to concerns regarding health data ownership, privacy, and security[30,31]. Possible malicious uses of AI technology have been reviewed and could include: breaches of data security and privacy, hacking of algorithms with the intent to harm, manipulation of data, and much more[32]. Governance bodies should seek to define best practices, to mitigate security and safety threats, and to have action plans in case a malicious event occurs[32]. Guidance navigating expectations and consequences of the use of ML models in healthcare should be encouraged at all levels (patient, physician, institutions, and governing bodies). Excessive or absolute dependence on experimental models should be avoided until robust foundations and infrastructures are in place to mitigate risks associated with AI.
Clinical translation: real-world introduction
Watson et al. conducted semi-structured interviews with American Academic Medical Centers regarding their use of predictive modeling and ML techniques within clinical care[33]. The team identified specific barriers to the adoption and implementation of such models that encompassed several themes: culture and personnel, clinical utility, financing, technology, and data. Overall, multidisciplinary development teams were found to be essential to ensure the integration of ML tools into the clinical workflow. A well-defined clinical utility with clinically relevant parameters and actionable alerts ensured the usefulness of the ML tools. Securing funding was seen as a significant challenge to overcome to support all phases of ML model development and deployment. Partnerships with vendors could be considered to help overcome challenges associated with translation and the long-term sustainability of model deployment[33].
The generalizability of ML models to the real-world clinical realm can be limited despite rigorous internal and external validation studies. It has been shown that the real-world introduction of ML models sometimes leads to lower accuracy and higher false positives[34]. This discrepancy between experimentation and reality can be partially due to the datasets used. Research datasets have been shown to be constrained by stringent inclusion and exclusion criteria[35,36]. Clinical deployment, therefore, requires close model and output monitoring, followed by adjustments. Another aspect of validation can be the challenges in data sharing. In that regard, “federated learning” could enable the use of large multi-institution datasets by decentralizing data analysis and sharing computational models rather than data[37].
A disconnect between developers and users may sometimes occur. The technical expert team developing craniofacial surgery ML models may not be versed in the clinical needs and settings in which the technology will be deployed. An in-depth understanding of the clinical environments is key for both the development and translation of the ML tools to the bedside. Are there support team members available to perform data entry? Is the information obtained novel or more accurate than the one recorded from conventional clinical assessment? Can the outputs be easily interpreted? Are the output results clinically relevant and helpful in guiding the management of patients?[35]. The clinical utility of ML models needs to be properly estimated and clinical needs should therefore guide model development and tool creation.
Fostering clinical trust
Beyond weighing the recognized benefits and risks of the introduction of ML and AI in their practices, surgeons may experience a distrust toward AI systems and their outputs. This skepticism may come from a lack of transparency or understanding of the processes. The explainability factor is important for users in the context of clinical decision support systems[38,39]. Explainable AI (XAI) is an emerging field bridging humans with machines by enabling a better understanding of how a model’s decisions and predictions were made[40,41]. For clinicians to trust AI and ML models, such bidirectional dialogue and reasoning is crucial. Using XAI involves significant trade-offs, such as the cost of its incorporation. Costs mostly come from the computation required to create a dialogue and learning capabilities between the model and clinician. Another identified trade-off lies between performance and interpretability. It appears that the models offering the best performance metrics are also often the least explainable[42]. As medicine strives for the best clinical performance and outcomes, deployment of explainable yet less performant models may be questionable. Ultimately, surgeons will have to justify clinical decisions with models that they trust and can understand in order to provide optimal machine-augmented care. Explainability can help answer some ethical, societal, and regulatory apprehensions and requirements if paired with rigorous model validation techniques and bias assessments[38]. To sustainably translate ML models into clinical practice, XAI appears to be a fundamental investment that requires further attention and development. However, it is important to note that model explainability is not a substitute for model evaluation following evidence-based medicine best practices and robust statistical validation.
Sustainability after clinical translation
The long-term sustainability of ML in practice requires financial support for data quality and access, governance, security, continuous model validation, and operational deployment. The implementation of AI models in clinical practice may also require the creation of new roles to facilitate the adoption and maintenance of AI algorithms, such as data scientists and machine learning operations (MLOps) engineers. For example, MLOps engineers will help create systems that continuously monitor models to ensure a decline in model performance does not occur without clinical and operational teams being aware. This is critical as models can decline in performance based on data drift and other changes in the clinical real-world environment. To support this, business models and associated governance structures should be created[43]. They may vary in size and range from innovation clusters, combining local expertise in AI, translational research, digital health, statistics, and more, to centers of excellence in large organizations[44].
Final thoughts
As we enter an age of increased intersections between society, data, and technology, we will notice the rapid proliferation of ML models migrating from development labs into real-world surgical settings. We recommend craniofacial surgeons be open and enthusiastic about upcoming ML models and tools but also aware of their numerous limitations. Clinical deployment of such models is arduous yet promising for the advancement of surgical care at the patient, team and system levels. Successful and safe integration of these models into practice requires input from surgeons. Although clinical expertise cannot be replaced at this time, it can be augmented by ML models, and surgeons should not be afraid of being innovators or early adopters if they are equipped with knowledge and awareness.
The greatest enemy of knowledge is not ignorance, it is the illusion of knowledge - Stephen Hawking
DECLARATIONS
Authors’ contributions
Made substantial contributions to the conception, literature search, writing and review of the study: Roy M, Reid RR, Senkaiahliyan S, Doria AS, Phillips JH, Brudno M, Singh D
Availability of data and materials
Not applicable.
Financial support and sponsorship
None.
Conflicts of interest
Singh D is the co-founder and CEO of a Canadian healthcare technology start-up company called Hero AI, while the other authors have declared that they have no conflicts of interest.
Ethical approval and consent to participate
Not applicable.
Consent for publication
Not applicable.
Copyright
© The Author(s) 2024.
REFERENCES
1. Topol EJ. High-performance medicine: the convergence of human and artificial intelligence. Nat Med 2019;25:44-56.
2. Hernandez-Boussard T, Bozkurt S, Ioannidis JPA, Shah NH. MINIMAR (MINimum Information for Medical AI Reporting): developing reporting standards for artificial intelligence in health care. J Am Med Inform Assoc 2020;27:2011-5.
3. Schiff GD, Bates DW. Can electronic clinical documentation help prevent diagnostic errors? N Engl J Med 2010;362:1066-9.
4. Mak ML, Al-Shaqsi SZ, Phillips J. Prevalence of machine learning in craniofacial surgery. J Craniofac Surg 2020;31:898-903.
5. Jarvis T, Thornburg D, Rebecca AM, Teven CM. Artificial intelligence in plastic surgery: current applications, future directions, and ethical implications. Plast Reconstr Surg Glob Open 2020;8:e3200.
6. Kanevsky J, Corban J, Gaster R, Kanevsky A, Lin S, Gilardino M. Big data and machine learning in plastic surgery: a new frontier in surgical innovation. Plast Reconstr Surg 2016;137:890e-7e.
7. Zhu VZ, Tuggle CT, Au AF. Promise and limitations of big data research in plastic surgery. Ann Plast Surg 2016;76:453-8.
8. Kim YJ, Kelley BP, Nasser JS, Chung KC. Implementing precision medicine and artificial intelligence in plastic surgery: concepts and future prospects. Plast Reconstr Surg Glob Open 2019;7:e2113.
9. Murphy DC, Saleh DB. Artificial intelligence in plastic surgery: what is it? Where are we now? What is on the horizon? Ann R Coll Surg Engl 2020;102:577-80.
10. Dhillon H, Chaudhari PK, Dhingra K, et al. Current applications of artificial intelligence in cleft care: a scoping review. Front Med 2021;8:676490.
11. Wu J, Tse R, Shapiro LG. Learning to rank the severity of unrepaired cleft lip nasal deformity on 3D mesh data. Proc IAPR Int Conf Pattern Recogn 2014;2014:460-4.
12. Maier A, Hönig F, Bocklet T, et al. Automatic detection of articulation disorders in children with cleft lip and palate. J Acoust Soc Am 2009;126:2589-602.
13. Bouletreau P, Makaremi M, Ibrahim B, Louvrier A, Sigaux N. Artificial intelligence: applications in orthognathic surgery. J Stomatol Oral Maxillofac Surg 2019;120:347-54.
14. Patcas R, Bernini DAJ, Volokitin A, Agustsson E, Rothe R, Timofte R. Applying artificial intelligence to assess the impact of orthognathic treatment on facial attractiveness and estimated age. Int J Oral Maxillofac Surg 2019;48:77-83.
15. Du W, Bi W, Liu Y, Zhu Z, Tai Y, Luo E. Machine learning-based decision support system for orthognathic diagnosis and treatment planning. BMC Oral Health 2024;24:286.
16. Qamar A, Bangi SF, Barve R. Artificial intelligence applications in diagnosing and managing non-syndromic craniosynostosis: a comprehensive review. Cureus 2023;15:e45318.
17. Mashouri P, Skreta M, Phillips J, et al.
18. Pham TD, Holmes SB, Coulthard P. A review on artificial intelligence for the diagnosis of fractures in facial trauma imaging. Front Artif Intell 2023;6:1278529.
19. Wang HC, Wang SC, Yan JL, Ko LW. Artificial intelligence model trained with sparse data to detect facial and cranial bone fractures from head CT. J Digit Imaging 2023;36:1408-18.
20. Moon G, Lee D, Kim WJ, Kim Y, Sung KY, Choi HS. Very fast, high-resolution aggregation 3D detection CAM to quickly and accurately find facial fracture areas. Comput Methods Programs Biomed 2024;256:108379.
21. Moon G, Kim S, Kim W, Kim Y, Jeong Y, Choi H. Computer aided facial bone fracture diagnosis (CA-FBFD) system based on object detection model. IEEE Access 2022;10:79061-70.
22. Wang X, Xu Z, Tong Y, et al. Detection and classification of mandibular fracture on CT scan using deep convolutional neural network. Clin Oral Investig 2022;26:4593-601.
23. Roy M, Corkum JP, Shah PS, et al. Effectiveness and safety of the use of gracilis muscle for dynamic smile restoration in facial paralysis: a systematic review and meta-analysis. J Plast Reconstr Aesthet Surg 2019;72:1254-64.
24. Boonipat T, Asaad M, Lin J, Glass GE, Mardini S, Stotland M. Using artificial intelligence to measure facial expression following facial reanimation surgery. Plast Reconstr Surg 2020;146:1147-50.
25. Dusseldorp JR, Guarin DL, van Veen MM, Miller M, Jowett N, Hadlock TA. Automated spontaneity assessment after smile reanimation: a machine learning approach. Plast Reconstr Surg 2022;149:1393-402.
26. Sendak MP, Gao M, Brajer N, Balu S. Presenting machine learning model information to clinical end users with model facts labels. NPJ Digit Med 2020;3:41.
28. Knight W. The dark secret at the heart of AI. Available from: https://www.technologyreview.com/2017/04/11/5113/the-dark-secret-at-the-heart-of-ai/. [Last accessed on 5 Dec 2024].
29. Buolamwini J, Gebru T. Gender shades: intersectional accuracy disparities in commercial gender classification. In: Proceedings of the 1st Conference on Fairness, Accountability and Transparency. PMLR; 2018. pp. 77-91. Available from: https://proceedings.mlr.press/v81/buolamwini18a.html?mod=article_inline&ref=akusion-ci-shi-dai-bizinesumedeia. [Last accessed on 5 Dec 2024]
30. Kish LJ, Topol EJ. Unpatients-why patients should own their medical data. Nat Biotechnol 2015;33:921-4.
31. Murdoch B. Privacy and artificial intelligence: challenges for protecting health information in a new era. BMC Med Ethics 2021;22:122.
32. Brundage M, Avin S, Clark J, et al. The malicious use of artificial intelligence: forecasting, prevention, and mitigation. ArXiv. [Preprint.] Dec 1, 2024 [accessed 2024 Dec 5]. Available from: https://doi.org/10.48550/arXiv.1802.07228.
33. Watson J, Hutyra CA, Clancy SM, et al. Overcoming barriers to the adoption and implementation of predictive modeling and machine learning in clinical care: what can we learn from US academic medical centers? JAMIA Open 2020;3:167-72.
34. Salehinejad H, Kitamura J, Ditkofsky N, et al. A real-world demonstration of machine learning generalizability in the detection of intracranial hemorrhage on head computerized tomography. Sci Rep 2021;11:17051.
35. Mechelli A, Vieira S. From models to tools: clinical translation of machine learning studies in psychosis. NPJ Schizophr 2020;6:4.
36. Patel R, Oduola S, Callard F, et al. What proportion of patients with psychosis is willing to take part in research? A mental health electronic case register analysis. BMJ Open 2017;7:e013113.
38. Antoniadi AM, Du Y, Guendouz Y, et al. Current challenges and future opportunities for XAI in machine learning-based clinical decision support systems: a systematic review. Appl Sci 2021;11:5088.
39. Bussone A, Stumpf S, O’Sullivan D. The role of explanations on trust and reliance in clinical decision support systems. In: 2015 International Conference on Healthcare Informatics; 2015 Oct 21-23; Dallas, USA. IEEE; 2015. pp. 160-9.
40. Amann J, Blasimme A, Vayena E, Frey D, Madai VI; Precise4Q consortium. Explainability for artificial intelligence in healthcare: a multidisciplinary perspective. BMC Med Inform Decis Mak 2020;20:310.
41. Barredo Arrieta A, Díaz-Rodríguez N, Del Ser J, et al. Explainable artificial intelligence (XAI): concepts, taxonomies, opportunities and challenges toward responsible AI. Inform Fusion 2020;58:82-115.
42. Linardatos P, Papastefanopoulos V, Kotsiantis S. Explainable AI: a review of machine learning interpretability methods. Entropy 2020;23:18.
43. Accuracy. Artificial Intelligence Episode 1 - overview of leading artificial intelligence clusters around the globe. Available from: https://www.accuracy.com/perspectives/overview-leading-artificial-intelligence-clusters-around-globe. [Last accessed on 5 Dec 2024].
44. McKinsey and Company. Transforming healthcare with AI: the impact on the workforce and organizations. Available from: https://www.mckinsey.com/industries/healthcare-systems-and-services/our-insights/transforming-healthcare-with-ai. [Last accessed on 5 Dec 2024].
Cite This Article
How to Cite
Download Citation
Export Citation File:
Type of Import
Tips on Downloading Citation
Citation Manager File Format
Type of Import
Direct Import: When the Direct Import option is selected (the default state), a dialogue box will give you the option to Save or Open the downloaded citation data. Choosing Open will either launch your citation manager or give you a choice of applications with which to use the metadata. The Save option saves the file locally for later use.
Indirect Import: When the Indirect Import option is selected, the metadata is displayed and may be copied and pasted as needed.
About This Article
Special Issue
Copyright
Data & Comments
Data
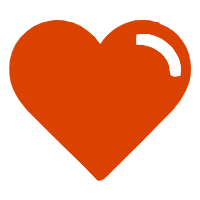
Comments
Comments must be written in English. Spam, offensive content, impersonation, and private information will not be permitted. If any comment is reported and identified as inappropriate content by OAE staff, the comment will be removed without notice. If you have any queries or need any help, please contact us at [email protected].