Patient perspectives on AI: a pilot study comparing large language model and physician-generated responses to routine cervical spine surgery questions
Abstract
Aim: The purpose of this study was to elucidate differences in patient perspectives on large language model (LLM) vs. physician-generated responses to frequently asked questions about anterior cervical discectomy and fusion (ACDF) surgery.
Methods: This cross-sectional study had three phases: In phase 1, we generated 10 common questions about ACDF surgery using ChatGPT-3.5, ChatGPT-4.0, and Google search. Phase 2 involved obtaining answers to these questions from two spine surgeons, ChatGPT-3.5, and Gemini. In phase 3, we recruited 5 cervical spine surgery patients and 5 age-matched controls to assess the clarity and completeness of the responses.
Results: LLM-generated responses were significantly shorter, on average, than physician-generated responses (30.0 +/- 23.5 vs. 153.7 +/- 86.7 words, P < 0.001). Study participants were more likely to rate LLM-generated responses with more positive clarity ratings (H = 6.25, P = 0.012), with no significant difference in completeness ratings (H = 0.695, P = 0.404). On an individual question basis, there were no significant differences in ratings given to LLM vs. physician-generated responses. Compared with age-matched controls, cervical spine surgery patients were more likely to rate physician-generated responses as higher in clarity (H = 6.42, P = 0.011) and completeness (H = 7.65, P = 0.006).
Conclusion: Despite a small sample size, our findings indicate that LLMs offer comparable, and occasionally preferred, information in terms of clarity and comprehensiveness of responses to common ACDF questions. It is particularly striking that ratings were similar, considering LLM-generated responses were, on average, 80% shorter than physician responses. Further studies are needed to determine how LLMs can be integrated into spine surgery education in the future.
Keywords
INTRODUCTION
Anterior cervical discectomy and fusion (ACDF) is a common surgical intervention for the management of cervical spinal pathologies, including degenerative disc disease (central and paracentral disc herniations, and cervical stenosis), traumatic injuries, infection, and tumors[1]. The procedure’s technical aspects have undergone significant evolution, enhancing surgical outcomes and patient recovery trajectories[2]. Despite the procedure’s high prevalence, the complexity of ACDF, the heterogeneous pathologies for which it is performed, and the varying surgical techniques pose challenges for patients attempting to understand the surgery’s risks, benefits, and postoperative recovery process[3].
Studies have shown that a significant proportion of patients rely on online resources to gather information about surgeries, and that facilitating access to online health information can bolster patient compliance, postoperative plan adherence, and support the patient-physician relationship. However, this reliance on digital health resources can prove problematic, as outdated, contradictory, or highly technical information can complicate the patient’s decision making[4]. In this context, Langford et al. emphasized the importance of integrating high-quality online information into medical consultations, significantly impacting patient care and the dialogue between patients and physicians[5]. Thus, patient-focused online educational resources must be precise, accessible, and importantly, accurate.
Studies have reported on the capability of large language models (LLMs), such as OpenAI’s ChatGPT and Google’s Gemini (formerly known as Bard), to parse through vast datasets and online surgical information to generate patient-specific responses that are coherent, comprehensive, and concise[6-8]. Nonetheless, the accuracy, clarity, and completeness with which LLMs navigate complex medical domains, interpret clinical nuances, and subsequently deliver patient-friendly explanations warrants validation and continuous refinement. While LLMs have the potential to enhance patient comprehension of their medical conditions and treatment options, thereby increasing transparency and trust in surgical decision making, they may also carry the risk of disseminating inaccurate or biased information that could mislead patients and adversely affect their decision making and health outcomes[9]. In this study, we evaluate the clarity and comprehensiveness of ChatGPT, Gemini, and two spine surgeons’ responses to ten frequently asked patient questions by comparing how cervical spine surgery patients and their age-matched non-surgical patient counterparts rated these answers in terms of clarity and completeness.
METHODS
This cross-sectional study was approved by the Stanford Institutional Review Board (IRB-eProtocol #73097), and informed consent was obtained from all study participants.
Study design and participants
Figure 1 shows the three phases of the study design. The goal of phase 1 was to craft ten commonly asked questions regarding ACDF surgery. To accomplish this goal, we utilized the following search engines: ChatGPT-3.5, ChatGPT-4.0, and Google. For both ChatGPT-3.5 and ChatGPT-4.0, the following prompt was submitted: “Suggest a list of the 20 most common frequently asked patient questions about anterior cervical discectomy and fusion”. The newly generated 40 questions were again submitted to ChatGPT-4.0 with the following prompt: “Consolidate themes and synthesize 10 questions from the following set of 40 questions: …”. Concurrently, a Google search for “frequently asked questions AND anterior cervical discectomy and fusion OR ACDF surgery” was submitted, and the first 50 websites meeting our website inclusion criteria [Table 1] were surveyed. After eliminating redundancies and applying exclusion criteria, we were able to generate 10 frequently asked questions about ACDF surgery [Table 2]. All searches for phase 1 occurred on November 6, 2023.
Inclusion and exclusion criteria for websites containing frequently asked questions regarding ACDF surgery
Inclusion criteria | Exclusion criteria |
Patient-focused, relevant questions and answers | Nongeneralizable physician anecdotes and physician-specific inquiries |
Evidenced-based medical websites | Proprietary surgical techniques and devices which are not widely available |
Information presented in the form of questions and answers | Research articles, non-patient-centered information |
Ten consolidated frequently asked questions administered to ChatGPT-3.5, Gemini, and doctors
Question 1 | What is ACDF surgery, and how is it performed? |
Question 2 | How long is the typical recovery and fusion period, and when can I expect to return to work and daily activities? |
Question 3 | What materials are used for fusion in ACDF surgery, and what are the complications associated with both the procedure and the materials? |
Question 4 | Why is ACDF surgery recommended, and what are its potential risks and benefits? |
Question 5 | What are the long-term outcomes, success rates, and potential long-term effects or risks associated with ACDF surgery? |
Question 6 | What restrictions or precautions should I be aware of during my recovery, including wearing a neck brace and certain activities to avoid? |
Question 7 | What should I expect post-surgery in terms of incisions, scars, pain management, and potential discomfort? |
Question 8 | How long will I need to stay in the hospital post-surgery, and will I require physical therapy or rehabilitation? |
Question 9 | How long does ACDF surgery typically take? |
Question 10 | Are there any alternative treatments to ACDF, and under what circumstances might this surgery be repeated for other disc issues? |
Phase 2 involved soliciting responses to our 10 commonly asked ACDF surgery questions from spine surgeons and LLMs. In this effort, we conducted interviews with two attending spine surgeons, including one neurosurgeon and one orthopedic surgeon. Both surgeons were given clear instructions to answer the questions as if they were answering questions from a patient. Notably, both surgeons were blinded to all aspects of our study’s design, including the questions themselves, prior to the day of the interview. Following the interview, a transcript of their answers was produced. We next asked the same questions to ChatGPT-3.5 and Gemini with the following prompt: “Speak as an expert spine surgeon who is up to date with the latest scientific research and has years of experience counseling patients with empathy and clarity. Provide a comprehensive and easily understandable answer to the following question about cervical spine fusion surgery. Limit your answer to 250 words and focus on the most important aspects to ensure clarity: …”. The word limit was determined based on the average length and range of our physician-generated responses. At the end of phase 2, we had a total of 40 answers (10 each from two spine surgeons, ChatGPT-3.5, and Gemini) to our 10 commonly asked questions [Supplementary Table 1]. All searches for phase 2 occurred on November 23, 2023.
Phase 3 involved recruiting study participants according to the inclusion and exclusion criteria shown in Table 3. In total, there were 10 participants including 5 patients who had previously had cervical spine surgery and 5 gender- and age-matched controls. All study participants were given the same questionnaire with the 10 commonly asked ACDF surgery questions and 40 answers from phase 2. Participants were asked to use a 5-point Likert scale (Strongly Disagree, Disagree, Neutral, Agree, and Strongly Agree) to rate every response on both clarity and completeness. The exact prompts in the questionnaire were “This answer is clear and easy to understand” and “This answer completely answers the question”. All participants were blinded to LLMs or physicians being involved in the generation of responses, and the responses for every question appeared in a random order for each participant. Phase 3 produced a total of 800 data points for subsequent analysis.
Inclusion and exclusion criteria used to select cervical spine surgery patients and age-matched controls
Inclusion criteria | Exclusion criteria |
Speaks and reads English at native level proficiency | Participants who did not fill out the study questionnaire |
Age > 18 years old | Participants who did not consent to participate in the study |
Patients must have a history of cervical spine surgery at Stanford from 2019 to 2023 | Age-matched controls cannot have a history of spine surgery |
Statistical methods
Data normality was assessed using the Shapiro-Wilk test. For non-normal data, the Kruskal-Wallis test and the Mann-Whitney U test were utilized, as appropriate, to assess differences in word counts by LLMs vs. physicians and overall ratings provided by patients and controls. Dunn-Bonferroni post-hoc test was conducted to pinpoint specific differences. Categories for the 100% stacked bar charts were set as follows: Likert ratings are converted to numbers (strongly disagree = 1, disagree = 2, neutral = 3, agree = 4, and strongly agree = 5), and then positive and negative feelings are combined to create categories (1 and 2 = disagree, 3 = neutral, 4 and 5 = agree). For our analyses, physician-generated response ratings were averaged. For a subset of the analysis, ChatGPT-3.5 and Gemini ratings were also averaged to assess patient perspectives on the two different LLMs. Independent two-sample t-test was used to compare ratings for each question. Inter-rater reliability was assessed using Fleiss’ Kappa. Kappa values of > 0.80 indicate excellent reliability; 0.61 to 0.80, substantial reliability; 0.41 to 0.60, moderate reliability; 0.21 to 0.40, fair reliability; and ≤ 0.20, poor reliability[10]. The level of statistical significance was set at P < 0.05 or a specifically listed P-value when a conservative Bonferroni correction was applied in instances of analyses for multiple comparisons. All statistical analyses were executed using R Studio (version 4.1.2) or Python (version 3.8; Python Software Foundation).
RESULTS
The Shapiro-Wilk test indicated that the data were not normally distributed (W = 0.825, P < 0.001). This finding justified the use of non-parametric statistical methods for subsequent analyses.
Word count analysis
Compared to physician-generated responses, ChatGPT-3.5 and Gemini produced markedly shorter responses to every question (LLM avg = 30.0 +/- 23.5 vs. doctors avg = 153.7 +/- 86.7 words; P < 0.01; Figure 2). Despite being asked to limit responses to 250 words, the longest responses produced by ChatGPT-3.5 and Gemini were 77 and 107 words, respectively, while the average LLM responses were 31 and 28.9 words, respectively. Responses from physicians were significantly longer, with an average of 153.7 words per question [Table 4]. Overall, LLMs produced significantly shorter responses than physician-generated responses (P < 0.001). Comparisons of individual LLM platforms also revealed shorter responses produced by ChatGPT-3.5 vs. physicians (P < 0.001) and shorter responses produced by Gemini vs. physicians (P < 0.001), but no statistical difference in word count between ChatGPT-3.5 vs. Gemini (P = 0.383).
Figure 2. Line graph showing total and average word count for answers to the 10 frequently asked questions regarding ACDF surgery generated by ChatGPT-3.5, Gemini, and doctors. ACDF: Anterior cervical discectomy and fusion.
Word counts for answers by ChatGPT-3.5, Gemini, and doctors
ChatGPT-3.5 | Gemini | LLM (average) | Doctors (average) | |
Question 1 | 77 | 107 | 92 | 119 |
Question 2 | 41 | 30 | 36 | 272 |
Question 3 | 29 | 25 | 27 | 193 |
Question 4 | 34 | 34 | 34 | 178 |
Question 5 | 30 | 25 | 28 | 153 |
Question 6 | 22 | 13 | 18 | 119 |
Question 7 | 19 | 11 | 15 | 207 |
Question 8 | 17 | 18 | 18 | 93 |
Question 9 | 11 | 5 | 8 | 56 |
Question 10 | 30 | 21 | 26 | 149 |
Average | 31 | 28.9 | 30.0 | 153.7 |
STDEV | 18.4 | 28.8 | 23.4 | 86.7 |
Aggregate ratings for LLM vs. physician-generated responses
Analysis of overall clarity ratings for LLM responses from study participants (n = 10) revealed that 75% agreed that responses were clear, while 6.5% disagreed and 18.5% were neutral. Clarity ratings for physician-generated responses showed that a statistically significantly lower 62.5% agreed that responses were clear, while 20% disagreed and 17.5% were neutral [Figure 3A]. Analysis of completeness ratings for Chatbot responses revealed that 63% agreed that responses were complete, while 18.5% disagreed and 18.5% were neutral. Completeness ratings for physician-generated responses showed that 64.5% agreed that responses were complete, while 12% disagreed and 23.5% were neutral [Figure 3B].
Figure 3. (A) Aggregate clarity and (B) completeness ratings, expressed in percentages, from all study participants (n = 10) comparing LLM vs. physicians-generated responses. LLM: Large language model.
Overall, study participants were more likely to agree that responses generated by LLMs were clearer compared to responses generated by physicians (H = 6.25, P = 0.012). Despite the differences seen in the word count analysis, findings from study participants’ ratings do not support differences in ratings for completeness between LLM vs. physician-generated responses (H = 0.695, P = 0.404). When comparing responses to each individual question, there were no significant differences between clarity or completeness ratings for LLM vs. physician-generated responses [Figure 4A and B].
Perspectives of cervical spine patients vs. controls
Ratings from cervical spine surgery patients (n = 5) were compared to those of gender- and age-matched controls (n = 5). There was an overall trend of patients being more likely to agree with statements about clarity and completeness compared to age-matched controls. Compared to controls, cervical spine surgery patients were more likely to give higher ratings for clarity (H = 6.42, P = 0.011) and completeness (H = 7.65, P = 0.006) for the physician-generated answers. Patients also showed a trend of rating LLM responses higher on clarity (H = 3.04, P = 0.081) and completeness (H = 2.79, P = 0.09) compared to the control group, but these differences did not reach statistical significance [Figure 5A and B]. Next, we separated the two LLM platforms to see if there were differences in patients vs. controls rating ChatGPT and Gemini responses. Compared to controls, spine surgery patients gave ChatGPT responses higher clarity ratings (H = 9.06, P = 0.003), with no significant differences in clarity ratings for Gemini responses (H = 0.01, P = 0.930). There were no significant differences between patients and controls on completeness ratings for ChatGPT (H = 5.36, P = 0.206) or Gemini separately (H = 1.61, P = 0.204) [Supplementary Figure 1A and B].
Figure 5. (A) Clarity and (B) completeness ratings, expressed in percentages, from cervical spine surgery patients (n = 5) vs. age-matched controls (n = 5) comparing answers generated by LLMs and physicians. LLMs: Large language models.
Comparisons of individual questions revealed no statistically significant differences in clarity or completeness between patients and controls for responses by ChatGPT-3.5, Gemini, and physician-generated answers [Supplementary Figure 2A and B, Supplementary Table 2]. Overall, study participants exhibited poor to fair inter-rater reliability in ratings for LLM vs. physician-generated responses with regards to clarity (LLMs: k = 0.16, P < 0.001; Physicians: k = 0.24, P < 0.001) and completeness (LLMs: k = 0.23, P < 0.001; Physicians: k = 0.12, P < 0.001).
DISCUSSION
Several recent studies have demonstrated the potential of LLMs to deliver precise medical information and educate patients across various medical specialties[11-13]. This study used patients who had undergone cervical spine surgery and gender- and age-matched controls to investigate perspectives on LLMs vs. physician-generated answers to commonly asked questions regarding ACDF surgery. We found that study participants were more likely to rate LLMs than physician-generated responses with positive ratings for clarity. Despite LLM responses being much shorter than physician-generated responses, they received equal ratings on completeness. This finding is exciting as it demonstrates that LLMs can provide short, concise responses to complex medical questions that are both clear and complete, appealing to patients and controls alike.
We also found that, when compared to age-matched controls, patients were more likely to rate physician-generated responses as clear and complete. This could potentially be explained by the patients having recently undergone spine surgery and spine surgery education (from the surgeon and surgical team), so that they are more familiar with medical terminology regarding ACDF surgery. This is further supported by patients also showing a trend of giving higher clarity and completeness ratings to LLM responses, potentially reflecting their familiarity with the subject matter. The familiarity with spine surgery likely introduces a bias for these patients, leading to a preference for responses that align with their prior knowledge. While this effect is evident in our study, it could potentially be generalized to other medical contexts, particularly where patients have prior experience or familiarity with a specific procedure. However, more research with larger sample sizes is needed to confirm this effect across different medical questions and procedures. For patients without prior surgery experience, LLMs could offer a more neutral perspective, potentially leveling the playing field between LLM and physician-generated responses. To better meet the needs of such patients, LLMs could be tailored with explanations that build foundational understanding and make complex medical information more accessible to those with less familiarity. Practically, this can be accomplished with more specific LLM prompting based on one’s prior understanding (or lack thereof) of the medical intervention. The lack of significant differences in individual question responses in our study is important because it validates that our findings are not skewed by any particular question, ultimately reinforcing the reliability of our findings.
As LLMs become more advanced, including faster and better at responding to complex medical questions clearly and completely, it may become prudent for physicians to employ LLMs as tools to improve practice efficiency and patient education. A recent study by Jahanshahi et al. assessed AI and machine learning techniques to process online messages between doctors and patients and to generate multiple automatic responses[14]. Their machine learning model “BERT” was able to achieve an accuracy rate of 85.41% when suggesting the top 3 doctor responses. Worldwide, other studies have employed LLMs in telemedicine to reduce barriers to healthcare access and receive quick consultations in the setting of a pandemic[15-17]. Collectively, these studies suggest that LLMs show great potential for quickly addressing medical questions from patients. Building upon this research, our study found that both spine patients and non-spine patient controls were satisfied with the clarity and completeness of LLM, as compared to physician-generated responses, and that LLMs outperformed physicians in some respects including brevity and clarity.
Our study is limited by its small sample size and poor to fair inter-rater reliability. The uniformly low to fair interrater reliability across all questions is likely due to differences in participants’ background knowledge and potential ambiguities in our questions. Our initial intent was to capture the participant’s gut reaction and initial response to the educational material, which is why we did not provide in-depth training. It is likely (and has been shown here) that these “gut reactions” or impulse responses are less reliable than ones that are given with systematic criteria. To improve reliability in future studies, we could provide rater training to ensure raters are aligned in their understanding of evaluation criteria. This study is also limited in that we used the free, more easily accessible ChatGPT-3.5, instead of paying for the newest version ChatGPT-4.0 which is - at the time of writing - OpenAI’s most advanced system featuring the most safe and useful responses[18]. It is important to consider the differences between these models since advancements in models’ abilities can significantly enhance their performance. Specifically, ChatGPT-4.0 boasts significant improvements in understanding and generating human-like text, likely resulting in higher accuracy and a deeper comprehension of complex topics. If ChatGPT-4.0 had been used in our study, the responses might have been clearer and more closely aligned with expert-level answers, potentially influencing our assessment of AI’s utility in this study[19]. We expect that as the models continue to be refined, the capabilities of LLMs in this space will only improve.
We nevertheless feel that our study is significant in that it is the first of its kind to specifically evaluate LLM vs. physician-generated responses regarding ACDF surgery and the first to look for differences between patient and non-patient populations. Future studies examining patient perspectives on LLM vs. physician-generated responses should explore multiple other dimensions associated with patient satisfaction, including empathy and perceived trustworthiness of the response. Prior research has shown that physicians are more likely to rate LLM-produced responses as higher in empathy compared to physician-generated responses[20]. Another study revealed that ChatGPT-4.0 shows the capacity for empathy when used to answer USMLE Step 2 Clinical Skills questions which are known to forecast performance in key residency domains, such as patient care, teamwork, professionalism, and communication[21,22]. These studies both beg the question of whether the empathy, imparted by artificial intelligence, is felt by patients scouring through LLMs for answers to their healthcare queries. The impact of significantly shorter responses associated with LLMs vs. physicians is also an avenue worth exploring as a measure of patient satisfaction in future studies.
While our study provides valuable insights, it also raises several important research questions that warrant further exploration. Future studies could investigate how LLMs perform across various medical specialties and how they manage more complex or sensitive patient inquiries. Understanding the impact of LLM-generated responses on patient decision making is another exciting area for future research. There is also great potential in determining how a combined model that integrates LLMs with physician oversight changes the surgical decision-making process, potentially in terms of increasing or decreasing the number of patients who opt for surgical intervention, and/or improving their comfort level and understanding of the risks/benefits.
One final exciting application of AI in surgery is its significant role in enhancing the surgical consent process in spinal surgery. Recent studies have demonstrated that AI can effectively simplify complex medical information, improving readability and comprehension for patients. For example, Ali et al. showed that ChatGPT-4.0 could generate procedure-specific consent forms at an average 6th-grade reading level, significantly enhancing patient understanding without sacrificing important medical details[23]. This AI-human expert collaborative approach not only improves patient education but also addresses medico-legal concerns by ensuring that consent forms meet both medical and legal standards. Given the litigious nature of spinal surgery, it is critical for future work to address the medico-legal implications of incorporating AI into this field. LLMs can still produce errors or “hallucinations”, making it essential to implement strict validation processes to ensure that only the most accurate information is conveyed to patients[24]. This is particularly important as AI-generated content becomes more integrated into surgical decision making, where the stakes are highest.
Notably, ChatGPT provided the following statement after answering the last question: “Always consult with your spine surgeon for personalized advice and to address specific concerns regarding your ACDF surgery”. As LLMs evolve and enhance their precision, clarity, and comprehensiveness, it is important for physicians and medical researchers to evaluate and study the best way to incorporate these tools into the routine care of our patients moving forward.
DECLARATIONS
Authors’ contributions
Made substantial contributions to the conception and design of the study: Yoseph ET, Lang S, Zygourakis CC
Performed data analysis and interpretation: Yoseph ET, Gonzalez-Suarez AD
Supported data acquisition: Yoseph ET, Zygourakis CC, Hu SS, Desai A
Wrote the manuscript: Yoseph ET, Gonzalez-Suarez AD, Zygourakis CC
Critically revised and approved final manuscript: Yoseph ET, Gonzalez-Suarez AD, Lang S, Desai A, Hu SS, Zygourakis CC
Availability of data and materials
Data are available in Supplementary Tables.
Financial support and sponsorship
None.
Conflicts of interest
Zygourakis CC is a consultant for Stryker and Amgen; Desai A is a consultant for Stryker and Carlsmed. While the other authors have declared that they have no conflicts of interest.
Ethical approval and consent to participate
Informed consent was gathered from each participant and this study was approved by the Stanford University Institutional Review Board (IRB-eProtocol #73097).
Consent for publication
Not Applicable.
Copyright
© The Author(s) 2024.
Supplementary Materials
REFERENCES
2. Gould H, Sohail OA, Haines CM. Anterior cervical discectomy and fusion: techniques, complications, and future directives. Semin Spine Surg 2020;32:100772.
3. Gaudin D, Krafcik BM, Mansour TR, Alnemari A. Considerations in spinal fusion surgery for chronic lumbar pain: psychosocial factors, rating scales, and perioperative patient education - a review of the literature. World Neurosurg 2017;98:21-7.
4. Cline RJ, Haynes KM. Consumer health information seeking on the Internet: the state of the art. Health Educ Res 2001;16:671-92.
5. Langford AT, Roberts T, Gupta J, Orellana KT, Loeb S. Impact of the Internet on patient-physician communication. Eur Urol Focus 2020;6:440-4.
6. Thirunavukarasu AJ, Ting DSJ, Elangovan K, Gutierrez L, Tan TF, Ting DSW. Large language models in medicine. Nat Med 2023;29:1930-40.
7. Hung YC, Chaker SC, Sigel M, Saad M, Slater ED. Comparison of patient education materials generated by chat generative pre-trained transformer versus experts: an innovative way to increase readability of patient education materials. Ann Plast Surg 2023;91:409-12.
8. Lang SP, Yoseph ET, Gonzalez-Suarez AD, et al. Analyzing large language models’ responses to common lumbar spine fusion surgery questions: a comparison between ChatGPT and Bard. Neurospine 2024;21:633-41.
9. Blease C, Bernstein MH, Gaab J, et al. Computerization and the future of primary care: a survey of general practitioners in the UK. PLoS One 2018;13:e0207418.
10. Landis JR, Koch GG. The measurement of observer agreement for categorical data. Biometrics 1977;33:159-74.
11. Goodman RS, Patrinely JR, Stone CA Jr, et al. Accuracy and reliability of chatbot responses to physician questions. JAMA Netw Open 2023;6:e2336483.
12. Subramanian T, Shahi P, Araghi K, et al. Using artificial intelligence to answer common patient-focused questions in minimally invasive spine surgery. J Bone Joint Surg Am 2023;105:1649-53.
13. Mika AP, Martin JR, Engstrom SM, Polkowski GG, Wilson JM. Assessing ChatGPT responses to common patient questions regarding total hip arthroplasty. J Bone Joint Surg Am 2023;105:1519-26.
14. Jahanshahi H, Kazmi S, Cevik M. Auto response generation in online medical chat services. J Healthc Inform Res 2022;6:344-74.
15. Aggarwal A, Tam CC, Wu D, Li X, Qiao S. Artificial intelligence-based chatbots for promoting health behavioral changes: systematic review. J Med Internet Res 2023;25:e40789.
16. Bharti U, Bajaj D, Batra H, Lalit S, Lalit S, Gangwani A. Medbot: conversational artificial intelligence powered chatbot for delivering tele-health after COVID-19. In: 2020 5th International Conference on Communication and Electronics Systems (ICCES); 2020 Jun 10-12; Coimbatore, India. IEEE; 2020. pp. 870-5.
17. Laranjo L, Dunn AG, Tong HL, et al. Conversational agents in healthcare: a systematic review. J Am Med Inform Assoc 2018;25:1248-58.
18. OpenAI. GPT-4. Available from: https://openai.com/gpt-4. [Last accessed on 27 Sep 2024].
19. OpenAI. GPT-4 Research. Available from: https://openai.com/index/gpt-4-research/. [Last accessed on 27 Sep 2024].
20. Brin D, Sorin V, Vaid A, et al. Comparing ChatGPT and GPT-4 performance in USMLE soft skill assessments. Sci Rep 2023;13:16492.
21. Sharma A, Schauer DP, Kelleher M, Kinnear B, Sall D, Warm E. USMLE step 2 CK: best predictor of multimodal performance in an internal medicine residency. J Grad Med Educ 2019;11:412-9.
22. Ayers JW, Poliak A, Dredze M, et al. Comparing physician and artificial intelligence chatbot responses to patient questions posted to a public social media forum. JAMA Intern Med 2023;183:589-96.
23. Ali R, Connolly ID, Tang OY, et al. Bridging the literacy gap for surgical consents: an AI-human expert collaborative approach. NPJ Digit Med 2024;7:63.
Cite This Article

How to Cite
Yoseph, E. T.; Gonzalez-Suarez A. D.; Lang S.; Desai A.; Hu S. S.; Zygourakis C. C. Patient perspectives on AI: a pilot study comparing large language model and physician-generated responses to routine cervical spine surgery questions. Art. Int. Surg. 2024, 4, 267-77. http://dx.doi.org/10.20517/ais.2024.38
Download Citation
Export Citation File:
Type of Import
Tips on Downloading Citation
Citation Manager File Format
Type of Import
Direct Import: When the Direct Import option is selected (the default state), a dialogue box will give you the option to Save or Open the downloaded citation data. Choosing Open will either launch your citation manager or give you a choice of applications with which to use the metadata. The Save option saves the file locally for later use.
Indirect Import: When the Indirect Import option is selected, the metadata is displayed and may be copied and pasted as needed.
About This Article
Special Issue
Copyright
Data & Comments
Data
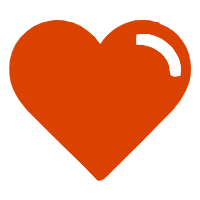
Comments
Comments must be written in English. Spam, offensive content, impersonation, and private information will not be permitted. If any comment is reported and identified as inappropriate content by OAE staff, the comment will be removed without notice. If you have any queries or need any help, please contact us at support@oaepublish.com.