Artificial intelligence and liver transplantation: looking inside the Pandora's box
Abstract
Artificial intelligence (AI) is the discipline of computer science dedicated to processing a large amount of throughput data and is based on algorithms that can rationalize increasingly complex tasks and ultimately reproduce human intelligence. It has been speculated for clinical uses in liver transplantation (LT) for several years, but its application remains incipient worldwide. Therefore, the recent advancements of digital and robotic tools in daily medical practice make the modern environment propitious to its proper implementation. Nevertheless, it is noteworthy that this technology has significant limitations: (i) its unconditional dependence on a pre-established reliable and extensive database; (ii) the potential impact on independent medical decision-making; and (iii) a major economic and environmental burden. So, despite its seducing and flawless simplicity features, AI emerges as a new “Pandora’s box” that should be carefully understood and used under the light of ethical principles to improve clinical outcomes, promote medical and para-medical working conditions, and increase patient safety and access to medical care. The present work aims to review literature data supporting AI implementation on this basis.
Keywords
INTRODUCTION
Liver transplantation (LT) currently represents the best curative treatment for diverse liver-related diseases and is a life-saving therapy. During the last decades, major advances in surgical technique, anesthesiological management, organ preservation, and immunosuppression therapies have been achieved, resulting in a one-year adult recipient and graft survivals of 94% and 92%, respectively, and a 10-year patient survival rate for pediatric recipients as good as 90.7%[1]. Based on these excellent results, the number of LTs performed worldwide has increased, and clinical indications have been progressively widened. Hence, the current most prominent challenges for the LT community are the organ shortage and the increasing complexity of organ allocation (e.g., oncological transplantation and exception points situations)[2].
In this context, it is noteworthy that LT is based on limited resources (i.e., liver grafts) and is highly costly, requiring significant human, structural, and instrumental investments. Moreover, it is a complex multidimensional and multifactorial process, which depends on the elaboration of dynamic variables related to donors, recipients, and logistical factors that encompass clinical, psychological, social, and environmental dimensions. These variables interact simultaneously and have short- and long-term effects not only on the single patient’s life but also on the whole transplant healthcare system. Managing all these complexities has relied on progressive experience accumulation, development of specific logistics systems, integrated and multidisciplinary collaboration, intense clinical and experimental research, implementation of new technologies, and personal commitment and resilience.
Artificial intelligence (AI) is the discipline of computer science that can simultaneously observe and rapidly process a large amount of throughput data and create algorithms that can rationalize increasingly complex and non-linear relationships, thus ultimately recapitulating human intelligence[3]. In medical practice, AI may provide new opportunities for developing evidence-based medicine. Its efficiency and accuracy may save time, resources, and money, and improve patient safety and outcomes. From this perspective, AI projects in healthcare involved more investments than those in any other sector worldwide in 2016[3].
Due to LT’s inherent multidimensional and multifactorial complexity, AI may represent an ideal tool to support and enhance LT practice. However, despite its deployment in clinical practice, which may appear technically feasible, particularly for those with solid computer science knowledge, several non-technical aspects could make AI a new Pandora’s box. The present review aimed to explore the methodological, ethical, legal, and socioeconomic issues that a clinician may face when implementing AI in a complex clinical setting such as LT. A systematic and detailed analysis of all potential clinical applications of AI and ML in LT was beyond the comprehensiveness of the present review. Instead, we preferred to build a pragmatic perspective for future users, who realistically are not in the position to explore and comprehend all the complex and specific technicalities of AI but clearly understand the complexities and limits of the real-world settings in which such technologies can potentially be deployed.
BIG DATA, BIG RESPONSIBILITY
AI works with a data-driven rather than a hypothesis-driven approach; therefore, its development and function depend essentially on data. Particularly, machine learning (ML) is specifically targeted to the management of vast and diverse collections of heterogeneous data that continue to grow exponentially over time and are generated from a wide variety of sources, i.e., big data. The digital transition in clinical practice has favored the creation of longitudinal electronic health records (EHRs), which record diverse and longitudinal data on diagnosis, procedures, medications, and labs for each patient. Moreover, developing federated or swarm methods for ML provides the appropriate framework for protected data sharing since they enable the training of models beyond numerous devices in different locations with no need to centralize or share the fundamental data[4,5]. Most ML algorithms currently available are based on structured data, which are limited by coding. Therefore, data incorporation and recording in EHR require a major revision of clinical routines across health facilities, which is indeed challenging. It has been estimated that up to 90% of digital data in healthcare are unstructured[6], and great efforts will be required to achieve a systematic, nationally/internationally agreed, structured, and measurable standardization and rationalization of imaging, laboratory, pathology, and clinical data.
LIVER TRANSPLANTATION PERSPECTIVES
LT would represent an ideal setting for deploying AI since LT consists of big data. Organ quality assessment, donor-recipient (D-R) matching, and recipient prioritization are just some clinical scenarios in which multiple, diverse, and dynamic clinical data are evaluated in the decision-making process. Besides the necessity of correctness and completeness in data collection, the most critical aspect that the process of AI deployment must manage is probably represented by data selection, operational definition/classification, and measurement, which are genuinely clinical rather than technological issues[4-7]. Moreover, classification, nomenclature, diagnostic criteria, and pathogenic theories evolve over time. A clear example in the LT setting is represented by hepatorenal syndrome (HRS), whose definition of HRS type-1 has undergone a radical revision after the concepts of acute kidney injury, acute and chronic kidney disease have been introduced[8]. Furthermore, some clinical classifications inherently present even a certain grade of subjectivity. Indeed, in the Child-Pugh classification of end-stage liver disease, two variables, ascites and hepatic encephalopathy, are qualitatively graded as absent, mild/moderate, and severe. Even histology, which currently represents the gold standard for acute rejection diagnosis, can be subjective, as shown by the continual updating of the Banff classifications over time[9].
Intuitively, supplementary complexity will not improve performance if primary variables and data are identical. In a study assessing 2,179 North American patients with acute-on-chronic liver failure, ML models showed comparable accuracy to the Model for End-Stage Liver Disease (MELD-Na) model in the prediction of patient death[10]. Therefore, the highest priority in contemporary research activity should probably target, even with the support of ML, the development/identification of new biomarkers to be implemented in clinical practice. Then, deploying AI models would be an achievement and an advance in real life.
The implementation of AI as a tool in translational medicine and omics research may confer a more straightforward applicability. Omics research aims to comprehensively identify, describe, and quantify the molecules and molecular processes that translate into the function, structure, and dynamics of cells and tissues[11]. There are diverse areas of upcoming research that can be classified as omics. For instance, the comprehensive analyses of RNA, genes, metabolites, and proteins can be defined as transcriptomics, genomics, metabolomics, and proteomics, respectively[12]. Clearly, the inherent high complexity of the omics data and models requires AI to be managed. The clinical application of such high-throughput analyses may provide new mechanistic insight and advances in translational medicine, thus potentially identifying new highly predictive and accurate biomarkers as well as key and specific interventional targets for precision medicine and individualized therapies[13,14]. Ischemia-reperfusion graft injury, immunotolerance, rejection, immunosuppression, and primary disease recurrence are probably the most prominent LT-related clinical aspects in which omics research may provide promising results with impactful clinical advances[11,14].
FRAILTY IN THE HIGHLIGHTS
Frailty has emerged as a precise biological syndrome characterized by decreasing physiological reserve and growing vulnerability to stress factors, resulting in multidimensional disturbs across the musculoskeletal, neurological, endocrine, immune system, and psychosocial spheres[15]. Assessing frailty in clinical practice demands complex efforts, including exhaustive examinations to comprehensively estimate the multidimensional aspect of frailty. Currently, the main assessment tools are based on performance and functional tests, which can exclusively be performed in a prospective manner, aside from being time-consuming and resource-consuming[14]. In this perspective, cirrhotic patients are at major risk of frailty and might show diverse clinical features related to a large range of frailty severeness[15,16]. Prevalence rates can reach up to 43%, depending on the studied population, definitions, and assessment methods[15,16]. Moreover, frailty has been singly related to the risk of specific cirrhosis complications, such as encephalopathy, ascites, variceal bleeding, and acute decompensation, and ultimately to waiting list drop-out and mortality. Despite the use of the principle of the “sickest-first” for prioritization in the current transplant decision-making process, the assessment and estimation of frailty become an issue of paramount significance that needs to be urgently tackled[15-17]. Furthermore, taking into account that the severity of liver disease at the moment of transplantation has worsened in recent years, the proportion of older adults (≥ 65 years) on the waiting lists increases, and the prevalence of liver disease associated with obesity is soaring, we can conclude that there is a growing number of LT recipients who become more and more complex from a medical point of view, and can notoriously be defined as “frail”[15,17]. In this context, frailty is inherently “big data”; an AI could efficaciously manage it technically, but AI outcomes may not currently be able to correctly address frailty from a clinical standpoint unless the above outlined methodological and ethical issues are primarily ruled out.
ORGAN ALLOCATION CHALLENGES
Medical AI models have been attracting attention primarily because of their capacity to identify highly complex non-linear patterns based on the analysis of massive amounts of complex variables. In addition, AI models are designed to continuously improve their performance and accuracy[18]. These seducing advantages have been claimed to be the ultimate solution for many medical problems, earning time and, theoretically, being cost-effective[3]. Considering the number of different variables used in the process of donor-recipient matching, organ allocation is one of the best examples of how AI models could be implemented to improve accuracy and decrease disparities. Nevertheless, we should consider that there is a significant risk of decreasing explainability and transparency while trying to increase the precision of these models[19]. Considering that the liver allocation process implicates legal and ethical features such as justice and equity, the opacity of those models may undermine human scrutability, making it hazardous to define the moral responsibility for the decisions that have been made or to identify possible bias that could perpetuate inequities[20]. In this perspective, Governmental institutions, such as the Committee of the Legal Affairs of the European Parliament, published in 2022 the “AI Liability Directive” with the perspective to address the legal risks related to the AI “black box” effect and to determine civil liability rules[21].
In transplant settings, disparities and inequity represent a huge and currently unsolved issue, with female sex and racial minorities being the most disadvantaged. Considering racial and sex differences among populations in large datasets, it is noteworthy that they are more often a consequence of socioeconomics and healthcare policies rather than genetic or biological factors[22]. Therefore, just adjusting highly sophisticated ML models for race or sex, while well-intentioned, would probably not overcome the inequity or even potentially exacerbate it. Before the worldwide adoption of the MELD score in the early 2000s, liver allocation was brimful of variability and inaccuracy, which resulted in inequities and a high rate of mortality while waiting for a liver graft[23]. The MELD score, which is calculated using simple lab tests - bilirubin, creatinine, international normalized ratio (INR), and more recently, sodium - is fairly correlated to a three-month mortality risk in patients with cirrhosis. Its implementation allowed transplant programs to improve and standardize organ allocation programs according to the principle of “the sickest first”, which significantly decreased mortality while waiting for a liver graft but not necessarily improved post-transplant survival. Despite its apparent good balance between simplicity and efficiency, the MELD score has been criticized for its limitation in avoiding inequity in specific situations and its inability to predict post-transplant mortality and avoid futile transplants.
One example of MELD’s drawbacks is the misestimation of women’s renal dysfunction. Because of a lesser muscle mass compared to men, renal function calculated by creatinine values leads to the attribution of lower scores to female candidates compared to male ones, and it negatively impacts women’s accessibility to liver transplantation[24]. In 2021, the MELD 3.0 was designed to eliminate this evident sex disparity[25]. Other corrected MELD scores have been designed with the same purpose, but allocation changes concerning liver size, driving smaller livers to shorter candidates, may also be needed to equalize access to liver transplants between men and women[26-28]. Size mismatches may lead to unfavorable outcomes and should be accurately evaluated before organ harvesting. Because of abdominal wall morphological constraints, this risk concerns more frequently women and young recipients. Graft volume has been a prominent issue in the field of liver transplant with live donors, but even if it is less frequent while considering cadaveric whole grafts, D-R matching for tiny recipients is definitely a subject of concern[29,30]. Intraoperative recognition of organ-recipient mismatches can be related to technical difficulties and early graft failure[31]. Deep learning (DP) models have recently been speculated to estimate liver graft weight automatically, including segmentation to optimize D-R matching[32]. This is a plausible clue of how AI can effectively optimize D-R pairing and improve recipients’ safety.
Another example of the limitation of current allocation systems is the “exception points” policy. It allows patients with certain conditions, such as HCC, to gain extra points and an advanced position on the waiting list. A study based on the Organ Procurement and Transplantation Network’s (OPTN) registries revealed that patients to whom MELD exception points were attributed had a lower risk of mortality on the waiting list, higher chances of being transplanted, and mostly were men[33]. In an ideal world, the policy of organ allocation would consider not only the principle of urgency but also the principles of “individual transplant benefit” and “population-based transplant benefit”[33]. In addition to the difficulties in prioritizing individuals on the waiting list considering all potential ethical issues, the challenge of matching a graft to a specific recipient based on different principles is a complex daily task experienced by liver transplant teams.
The Transplant Benefit System (TBS), developed in the UK, was one of the first propositions of a more developed model that considers not only donor but also recipient characteristics. Although not designed with AI models, this system is based on 28 variables, including 7 concerning the donor and 21 about the recipient - aiming to achieve a more accurate prediction of D-R matching outcomes[33]. A multicentric study assessing data from 11 Spanish transplant centers used 57 variables: 26 concerning the recipient, 19 regarding the donor, six regarding the harvesting procedure, and six regarding the transplant procedure. Artificial neural networks (ANN) models were used to predict the probability of graft survival with a sensitivity of 90.79% but showed suboptimal ability to predict graft loss[34]. This methodology was explored and validated in a second study performed at the King’s College Hospital during the same period, obtaining accurate predictions for 3 and 12 months, which was almost 15% higher than the best reached in the Spanish study. The discrepancy between these two studies highlights the lack of consistency when using specific AI models across different populations and environments[35]. There are many other models based on patients’ characteristics, donor risk features, and combined donor-recipient-based systems developed on traditional biostatistics. Most of them have been deeply reviewed, but unfortunately, hitherto, none of them could be considered suitable to give a perfect response to D-R pairing.
TRANSPLANT ONCOLOGY
Transplant oncology is not a completely new concept but has garnered increasing interest in recent decades. It is fundamentally based on the principles of radical oncological surgery and transplant medicine as it enables the removal of the entire tumor burden in addition to the underlying liver disease, avoiding recurrence. The first improvement toward a mature concept of oncological transplantation came with Mazzaferro et al., who defined for the first time a subgroup of candidates who could benefit from LT for HCC, achieving 5-year survival > 70%, what is called “Milan Criteria”[36]. In times of organ shortage, the increasing number of indications for LT tends to accentuate the “liver gap”, which is known as the discrepancy between patients on the waiting list and the annual liver transplants performed[37]. Despite all efforts of the transplant community to optimize the pool of available organs (e.g., living donors, split grafts, machine perfusions, and extended criteria grafts), the need for high-performance and reliable selection tools has become of paramount importance in order to avoid futile transplants[38]. For instance, liver transplantation for HCC has progressively increased and currently represents one-third of indications worldwide, with a recurrence rate that can reach up to 35% in patients beyond the Milan Criteria[39]. Factors related to recurrence can be classically divided into morphological features (based on radiological assessment), biomarkers (e.g., α-fetoprotein), and pathological features (micro-invasion and differentiation). Seeking more comprehensive models that could be able to analyze these different data, some teams proposed models based on omics technology and AI. Qu et al. developed a model of deep pathomics score (DPS) for estimating HCC recurrence following liver transplantation[40]. Despite the significant limitations of this model, which is based on training data from a single institution with predominantly HBV patients, the DPS model seemed to facilitate the identification of specific aspects of HCC and called attention to the importance of immune cells and tumor microenvironment. Another Asiatic team, using patients with treated hepatitis B and a machine learning model, developed a system based and validated on a large cohort of Korean and Caucasian patients that outperformed other risk scores[41]. It is called the Prediction of Liver Cancer using Artificial Intelligence-driven model for Network - hepatitis B (PLAN B model), which is available online as a web-based system (https://planbhcc.com). Multicentric and multinational consortiums seem to be a coherent and viable formula to develop and validate complex models that are highly dependent on costly investments and large-scale datasets. The RELAPSE model[42] is the result of the association of the US Multicenter HCC Transplant Consortium (UMHTC) and the European Hepatocellular Cancer Liver Transplant (EurHeCaLT) Study group to build and validate a model destinated to predict HCC recurrence and the outcomes based on more than 6,000 patients from 20 American and 6 European centers. The authors of this work advocate the use of their model to manage post-transplant follow-up, including modification of immunosuppression and ultimately defining the necessity or not of implementing adjuvant therapies in recipients with HCC according to the assessed recurrence risk.
EXPLAINABILITY AND TRANSPARENCY
AI’s accuracy encompasses so many complex processes that make the pathways by which the result was reached unintelligible to human minds. Therefore, while expert informaticians may discern the architecture of the AI system that processes the data, it is inexorably impossible to explain why and how a defined outcome was reached[43]. On the other hand, having a system that is as accurate as possible could be the solution to eliminating discrepancies and promoting equity and justice. In this context, some researchers claim that accuracy should be a more important value than explainability based on the argumentation that losing explainability could be acceptable when the benefits are superior to the costs[18]. Conversely, others advocate that although accuracy is an essential value in the context of outcome-oriented justice, explainability is a sine qua non condition for procedural fairness, such as scarce medical resources and organ allocation[44]. Moreover, explainability and transparency are essential to protect society from possible biases that might recurrently lead to favoring or harming particular groups or minorities, which can reproduce systemic inequalities and reinforce discriminatory trends. This debate raises several bioethical and philosophical points that become crucial in the process of designing and implementing AI decision-making models.
Analyzing clinical human decisions, we can define them as a sequence of objective and subjective reasoning. Objective reasoning is built based on scientific resources, as well as cumulative observation and knowledge. Our subjective reasoning also impacts on our decisions. It can be in accordance with our intuition, understanding, and in part, on the subconscious. Thus, in various degrees, pairing a donor with a recipient in the real world can also be impacted by uncontrollable factors[45]. Considering these mechanisms of the clinical decision-making process, one should claim that, similarly to AI algorithms, many human decisions lack transparency and explainability. So, can the medical community, society, and legal institutions accept the lack of AI transparency and explainability in the name of accuracy?
Obviously, there is a real need for more precise and comprehensive systems for organ allocation, not only in liver transplantation but also in other medical fields. AI systems seem to be a helpful tool for reaching goals such as accuracy, efficiency, and time savings. Nevertheless, because of its intrinsic drawbacks, the implementation of AI systems in liver transplantation, which depends immensely on public human resources, must be accompanied by a prior deep discussion with medical and societal representatives to address debatable points and survey its impact on medical practice and population health.
SUSTAINABILITY
Big data are associated with massive energy expenses and carbon imbalance, and their long-term sustainability must be critically debated. Data centers are intensive energy-consuming machinery that encompasses all the facilities necessary for information technology, including physical spaces, computers, data storage devices, and specialized human resources[45]. According to recent estimates, data centers have the fastest growing carbon imbalance index over the “Information and Communication Technology” sector due to their high rates of CO2 emissions and consumption of non-renewable energy, besides the massive waste production. Healthcare currently contributes 4.9% to the world’s carbon emissions, which is more than aviation (1.9%-2.4%) or shipping (1.7%)[46]. LT is considered one of the most resource-consuming procedures in current medical practice, and its impact is multidimensional, ranging from transports and logistics for organ allocation to waste disposal of the enormous amount of waste produced by surgery and anesthesia[47]. Therefore, the implementation of AI in LT clinical practice should probably take a broader perspective and strive to focus on its overall sustainability rather than just targeting an aprioristic, uncritical creation of massive, unsustainable ML models without any pragmatic clinical benefit just for the sake of the technology.
DIGITAL TRANSFORMATION
In the context of Digital Transformation in medicine, it is crucial to build digital models that can not only enhance accuracy but also tackle societal challenges and promote universal values that can positively impact our society and individuals. Therefore, it is paramount for the different representatives, such as education promoters, private and public organizations, clinical practitioners, and users, to work together to make comprehensive and responsible human-focused decisions. While there is clear evidence of the critical call for responsible design and implementation of AI in medicine, there is still a lack of research in the LT field on the basis of Responsible AI, Explainable AI, Human-centered AI, and Inclusive AI. Likewise, big data must be critically assessed in terms of environmental sustainability; the overall implementation of AI in clinical practice will require a debate on its socioeconomic sustainability. Currently, private investors and private data analytics companies are the leading actors in AI development, while the ongoing major global economic crisis has been putting significant pressure on publicly-funded health systems. LT is already a highly costly procedure, and the actual achievement that the transplant community should strive for with the implementation of new technologies should be not only the improvement of outcomes and safety but also the enhancement of equity and accessibility[21,44,48].
CONCLUSION
In conclusion, AI has a huge potential to enhance medical practice by processing massive amounts of complex throughput data. Its use in the field of LT is still incipient, but a plethora of speculations have emerged regarding its potential applications both pre- and post-transplantation. Nevertheless, there are some fundamental points concerning ethical, socioeconomic, legal, and even philosophical features that should be discussed and clarified to help institutions and individuals conduct a responsible digital transformation. The upcoming new era is still surrounded by much uncertainty, but the only aspect that is bound is that we are prone to live a milestone transformation that can change our society in a significant manner. A systematic and detailed analysis of all potential clinical applications of AI and ML in LT was beyond the scope of the present study, and rather than providing solutions, we actually meant to call attention and pose questions that may be neglected when technology is unconditionally considered the solution for clinical problems. So then, the actual scientific community and healthcare providers, as well as users, must be aware and ready to handle the present challenges that encompass education, planning, and regulation of the whole process in order to build a human-focused balance and avoid the digital dictatorship.
Key points
● Scientific questions remain the starting point and researchers should refrain from jumping into analyzing complex big data while neglecting the critical first step of putting data into context.
● The deployment of AI in clinical practice requires as a methodological prerequisite a systematic, nationally/internationally agreed, structured, and measurable standardization and rationalization of operational definitions, classification, measurement, and reporting of data (imaging, laboratory, pathology, and clinical data).
● AI promoters should balance the risks of losing explainability and transparency with the potential benefit of improving accuracy and efficiency on the basis of equity and justice.
● It is paramount for the different representatives such as private and public organizations, as well as field staff, to work together in order to make human-focused decisions from a problem-solving perspective rather than adapting human activity to technology.
● Economic, geographic, and epidemiological features must be taken into account to avoid resource wastefulness.
DECLARATIONS
Authors’ contributions
Designed the project, performed the literature review, and wrote the paper: Sepulveda A, Pravisani R
Availability of data and materials
Not applicable.
Financial support and sponsorship
None.
Conflicts of interest
Both authors declared that there are no conflicts of interest.
Ethical approval and consent to participate
Not applicable.
Consent for publication
Not applicable.
Copyright
© The Author(s) 2024.
REFERENCES
2. Gorji L, Brown ZJ, Limkemann A, Schenk AD, Pawlik TM. Liver transplant as a treatment of primary and secondary liver neoplasms. JAMA Surg 2024;159:211-8.
3. Sucher R, Sucher E. Artificial intelligence is poised to revolutionize human liver allocation and decrease medical costs associated with liver transplantation. Hepatobiliary Surg Nutr 2020;9:679-81.
4. Bhat M, Rabindranath M, Chara BS, Simonetto DA. Artificial intelligence, machine learning, and deep learning in liver transplantation. J Hepatol 2023;78:1216-33.
5. Balsano C, Burra P, Duvoux C, Alisi A, Piscaglia F, Gerussi A. Special Interest Group (SIG) Artificial Intelligence and Liver Disease; Italian Association for the Study of Liver (AISF). Artificial Intelligence and liver: opportunities and barriers. Dig Liver Dis 2023;55:1455-61.
6. Ge J, Kim WR, Lai JC, Kwong AJ. “Beyond MELD” - Emerging strategies and technologies for improving mortality prediction, organ allocation and outcomes in liver transplantation. J Hepatol 2022;76:1318-29.
7. Briceño J. Artificial intelligence and organ transplantation: challenges and expectations. Curr Opin Organ Transplant 2020;25:393-8.
8. Kahn J, Wagner D, Homfeld N, Müller H, Kniepeiss D, Schemmer P. Both sarcopenia and frailty determine suitability of patients for liver transplantation - a systematic review and meta-analysis of the literature. Clin Transplant 2018;32:e13226.
9. Angeli P, Garcia-Tsao G, Nadim MK, Parikh CR. News in pathophysiology, definition and classification of hepatorenal syndrome: a step beyond the International Club of Ascites (ICA) consensus document. J Hepatol 2019;71:811-22.
10. Hu C, Anjur V, Saboo K, et al. Low predictability of readmissions and death using machine learning in cirrhosis. Am J Gastroenterol 2021;116:336-46.
11. Liu Z, Xu J, Que S, et al. Recent progress and future direction for the application of multiomics data in clinical liver transplantation. J Clin Transl Hepatol 2022;10:363-73.
12. Martorell-Marugán J, Tabik S, Benhammou Y, et al. Deep learning in omics data analysis and precision medicine. In: Husi H, editor. Computational biology [Internet]. Brisbane (AU): Codon Publications; 2019. Chapter 3.
13. D’Adamo GL, Widdop JT, Giles EM. The future is now? Clinical and translational aspects of “Omics” technologies. Immunol Cell Biol 2021;99:168-76.
14. Ba R, Geffard E, Douillard V, et al. Surfing the big data wave: omics data challenges in transplantation. Transplantation 2022;106:e114-25.
15. Lai JC, Sonnenday CJ, Tapper EB, et al. Frailty in liver transplantation: an expert opinion statement from the American society of transplantation liver and intestinal community of practice. Am J Transplant 2019;19:1896-906.
16. Tandon P, Montano-Loza AJ, Lai JC, Dasarathy S, Merli M. Sarcopenia and frailty in decompensated cirrhosis. J Hepatol 2021;75:S147-62.
17. Maurice JB, Nwaogu A, Gouda M, Shaw O, Sanchez-Fueyo A, Zen Y. Acute Antibody-mediated rejection in liver transplantation: impact and applicability of the Banff working group on liver allograft pathology 2016 criteria. Hum Pathol 2022;127:67-77.
18. Veerankutty FH, Jayan G, Yadav MK, et al. Artificial Intelligence in hepatology, liver surgery and transplantation: emerging applications and frontiers of research. World J Hepatol 2021;13:1977-90.
19. Rueda J, Rodríguez JD, Jounou IP, Hortal-Carmona J, Ausín T, Rodríguez-Arias D. “Just” accuracy? Procedural fairness demands explainability in AI-based medical resource allocations. AI Soc 2024;39:1411-22.
20. Tsamados A, Aggarwal N, Cowls J, et al. The ethics of algorithms: key problems and solutions. AI Soc 2022;37:215-30.
21. European Parliament. Artificial intelligence liability directive. Available from: https://www.europarl.europa.eu/RegData/etudes/BRIE/2023/739342/EPRS_BRI(2023)739342_EN.pdf. [Last accessed on 30 Jul 2024].
22. Vyas DA, Eisenstein LG, Jones DS. Hidden in plain sight - reconsidering the use of race correction in clinical algorithms. N Engl J Med 2020;383:874-82.
23. Merion RM, Sharma P, Mathur AK, Schaubel DE. Evidence-based development of liver allocation: a review. Transpl Int 2011;24:965-72.
24. Uche-Anya E, Anyane-Yeboa A, Berzin TM, Ghassemi M, May FP. Artificial intelligence in gastroenterology and hepatology: how to advance clinical practice while ensuring health equity. Gut 2022;71:1909-15.
25. Kim WR, Mannalithara A, Heimbach JK, et al. MELD 3.0: the model for end-stage liver disease updated for the modern era. Gastroenterology 2021;161:1887-95.e4.
26. Asrani SK, Jennings LW, Kim WR, et al. MELD-GRAIL-Na: glomerular filtration rate and mortality on liver-transplant waiting list. Hepatology 2020;71:1766-74.
27. Nagai S, Nallabasannagari AR, Moonka D, et al. Use of neural network models to predict liver transplantation waitlist mortality. Liver Transpl 2022;28:1133-43.
28. Wood NL, VanDerwerken D, Segev DL, Gentry SE. Correcting the sex disparity in MELD-Na. Am J Transplant 2021;21:3296-304.
29. Park R, Lee S, Sung Y, et al. Accuracy and efficiency of right-lobe graft weight estimation using deep-learning-assisted ct volumetry for living-donor liver transplantation. Diagnostics 2022;12:590.
30. Yang X, Park S, Lee S, et al. Estimation of right lobe graft weight for living donor liver transplantation using deep learning-based fully automatic computed tomographic volumetry. Sci Rep 2023;13:17746.
31. Reyes J, Perkins J, Kling C, Montenovo M. Size mismatch in deceased donor liver transplantation and its impact on graft survival. Clin Transplant 2019;33:e13662.
32. Pettit RW, Marlatt BB, Corr SJ, Havelka J, Rana A. nnU-net deep learning method for segmenting parenchyma and determining liver volume from computed tomography images. Ann Surg Open 2022;3:e155.
33. Mathur AK, Schaubel DE, Gong Q, Guidinger MK, Merion RM. Sex-based disparities in liver transplant rates in the United States. Am J Transplant 2011;11:1435-43.
34. Briceño J, Calleja R, Hervás C. Artificial intelligence and liver transplantation: Looking for the best donor-recipient pairing. Hepatobiliary Pancreat Dis Int 2022;21:347-53.
35. Ayllón MD, Ciria R, Cruz-Ramírez M, et al. Validation of artificial neural networks as a methodology for donor-recipient matching for liver transplantation. Liver Transpl 2018;24:192-203.
36. Mazzaferro V, Regalia E, Doci R, et al. Liver transplantation for the treatment of small hepatocellular carcinomas in patients with cirrhosis. N Engl J Med 1996;334:693-9.
37. Soubrane O, Scatton O. The Development of Transplant Oncology May Worsen the Liver Gap and Needs New Technical Options in Liver Transplantation. Ann Surg 2024;279:226-7.
38. Mehta N, Bhangui P, Yao FY, et al. Liver transplantation for hepatocellular carcinoma. Working group report from the ILTS transplant oncology consensus conference. Transplantation 2020;104:1136-42.
39. Tabrizian P, Holzner ML, Mehta N, et al. Ten-year outcomes of liver transplant and downstaging for hepatocellular carcinoma. JAMA Surg 2022;157:779-88.
40. Qu WF, Tian MX, Lu HW, et al. Development of a deep pathomics score for predicting hepatocellular carcinoma recurrence after liver transplantation. Hepatol Int 2023;17:927-41.
41. Kim HY, Lampertico P, Nam JY, et al. An artificial intelligence model to predict hepatocellular carcinoma risk in Korean and Caucasian patients with chronic hepatitis B. J Hepatol 2022;76:311-8.
42. Tran BV, Moris D, Markovic D, et al. Development and validation of a REcurrent Liver cAncer Prediction ScorE (RELAPSE) following liver transplantation in patients with hepatocellular carcinoma: analysis of the US Multicenter HCC Transplant Consortium. Liver Transpl 2023;29:683-97.
43. London AJ. Artificial intelligence and black-box medical decisions: accuracy versus explainability. Hastings Cent Rep 2019;49:15-21.
44. Starke G, De Clercq E, Elger BS. Towards a pragmatist dealing with algorithmic bias in medical machine learning. Med Health Care Philos 2021;24:341-9.
45. Lucivero F. Big data, big waste? A reflection on the environmental sustainability of big data initiatives. Sci Eng Ethics 2020;26:1009-30.
46. National Institute for Health and Care Research Global Health Research Unit on Global Surgery. Reducing the environmental impact of surgery on a global scale: systematic review and co-prioritization with healthcare workers in 132 countries. Br J Surg 2023;110:804-17.
47. Filipponi F. Liver transplantation: is it a sustainable practice? Dig Liver Dis Suppl 2011;5:6-9.
Cite This Article
Export citation file: BibTeX | EndNote | RIS
OAE Style
Sepulveda A, Pravisani R. Artificial intelligence and liver transplantation: looking inside the Pandora's box. Art Int Surg 2024;4:170-9. http://dx.doi.org/10.20517/ais.2024.11
AMA Style
Sepulveda A, Pravisani R. Artificial intelligence and liver transplantation: looking inside the Pandora's box. Artificial Intelligence Surgery. 2024; 4(3):170-9. http://dx.doi.org/10.20517/ais.2024.11
Chicago/Turabian Style
Sepulveda, Ailton, and Riccardo Pravisani. 2024. "Artificial intelligence and liver transplantation: looking inside the Pandora's box" Artificial Intelligence Surgery. 4, no.3: 170-9. http://dx.doi.org/10.20517/ais.2024.11
ACS Style
Sepulveda, A.; Pravisani R. Artificial intelligence and liver transplantation: looking inside the Pandora's box. Art. Int. Surg. 2024, 4, 170-9. http://dx.doi.org/10.20517/ais.2024.11
About This Article
Special Issue
Copyright
Data & Comments
Data
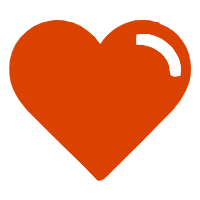
Comments
Comments must be written in English. Spam, offensive content, impersonation, and private information will not be permitted. If any comment is reported and identified as inappropriate content by OAE staff, the comment will be removed without notice. If you have any queries or need any help, please contact us at support@oaepublish.com.