Evolution of the treatment of esophageal cancer: artificial intelligence and the role of sentinel lymph node assessment in esophageal cancer
Abstract
Sentinel lymph node (SLN) biopsy has revolutionized the staging and prognosis of breast cancer and melanoma. Because of the complicated lymphatic network around the esophagus, the utility of SLN biopsy for esophageal cancer is less clear. The accuracy of SLN mapping in esophageal cancer depends on tumor site, disease stage, use of neoadjuvant therapy, and patient characteristics. SLN biopsy may improve staging and result in less morbidity in patients with early esophageal cancer, compared with radical lymphadenectomy and esophagectomy. A recent study that investigated hybrid tracers in sentinel node navigation surgery (SNNS) demonstrated promising results for the detection of peritumoral SLNs. However, evidence that firmly establishes the concept of the SLN for esophageal cancer is still lacking. Big data analytics and artificial intelligence have been associated with improvements in the detection and prognosis of esophageal cancer. This review considers the roles of the evolving technologies of SLN biopsy and artificial intelligence, which together have the potential to further improve prognoses and outcomes for patients with esophageal cancer. Additional investigation is necessary to establish standardized protocols and to determine the long-term effectiveness of these approaches in settings involving neoadjuvant therapy and advanced-stage disease.
Keywords
INTRODUCTION
Sentinel lymph node (SLN) assessment has evolved considerably during the last 30 years and is now associated with less morbidity and equivocal survival outcomes, compared with radical resection of lymph node basins[1,2]. SLN biopsy is widely used for staging and prognostication of breast cancer and melanoma[3-6]. Emerging technologies, such as artificial intelligence (AI) and robotic surgery, and the availability of new molecular particles for staining of lymph nodes have the potential to further advance the assessment of SLNs across cancer types.
SLN biopsy may have a role to play in the staging, prognostication, and management of gastrointestinal cancer, although the available data supporting its utility for this disease are insufficient and its use in this context remains somewhat controversial. However, recent technological advances may help to clarify the use of SLN biopsy in esophageal cancer. AI is poised to revolutionize every facet of our lives, including medical research. Pathologic evaluation of lymph nodes for the presence of metastatic cancer cells can be cumbersome, with long assessment times and high rates of false positives and false negatives. AI has been applied to assess whole-slide images to facilitate the automatic detection of lymph node metastasis and may one day be used in standard pathologic practice to achieve efficient and accurate detection of lymph node metastases[7]. In an analogous application, AI combined with robotic surgery and its various technological applications may further allow real-time assessment of the lymph nodes in cancer[8]. In this review, we discuss the current state of SLN mapping in esophageal cancer and consider the growth of AI within this context.
CURRENT CHALLENGES
The challenges posed in applying SLN mapping to esophageal cancer include the location of the tumor in relation to the length of the esophagus, the stage of the cancer, and the individual tumor and patient characteristics [Table 1][9]. In addition, technical factors, such as the type of dye(s) used, the timing of the injection, and the available resources and provider expertise, can affect the success or failure of the application of this advanced technology[9-11]. For these and other reasons, the use of SLN biopsy for esophageal and other gastrointestinal cancers is both controversial and challenging. The location and length of the esophagus spanning across three anatomically distinct regions (neck, chest, and abdomen), the multidirectional flow of esophageal lymphatics, the potential presence of skip metastases, the use of neoadjuvant chemotherapy and radiotherapy, the subsequent effect of these treatments on tissue, and the dearth of relevant data compound the challenges of using SLN biopsy in patients with esophageal cancer[12,13]. Despite these challenges, research has shown that SLN biopsy may be feasible in patients with early esophageal cancer, thus offering the benefit of limited lymphadenectomy and decreased morbidity[10,11,14]. The use of a second, complementary injectable dye for the identification of SLNs can improve the specificity of detection. Finally, the addition of robotic AI as an overlay holds tremendous potential to further improve SLN assessment. Taken together, these advances have the potential to greatly enhance our ability to accurately assess SLNs, which was once thought to be impossible, given the complexities of esophageal lymph nodes[13]. To gain better insights into the evolution of SLN mapping in esophageal cancer, it is helpful to trace the origins of the technique.
Challenges, technological advances, and key studies regarding the use of SLN assessment in esophageal cancer
Category | Challenges and technological advances in esophageal cancer |
Challenges | ● Tumor location ● Stage of cancer ● Dye particle size ● Timing of dye administration ● Individual tumor heterogeneity ● Patient characteristics |
Technological advances | ● Use of multiple complementary injectable dyes ● Smaller particle size ● Use of AI and machine learning overlay |
Key studies | ● Caldonazzi et al. (2023). Value of artificial intelligence in evaluating lymph node metastases[7] ● Kitagawa et al. (2000). Esophageal Cancer Practice Guidelines 2017 edited by the Japan Esophageal Society: part 1[9] ● Kato et al. (2003). Sentinel lymph nodes with technetium-99m colloidal rhenium sulfide in patients with esophageal carcinoma[10] ● Hagens et al. (2020). Distribution of lymph node metastases in esophageal carcinoma patients undergoing upfront surgery: a systematic review[13] ● Frederiks et al. (2023). Feasibility and safety of tailored lymphadenectomy using sentinel node-navigated surgery in patients with high-risk T1 esophageal adenocarcinoma[42] |
SLN MAPPING
In their early study of SLNs in gastrointestinal cancer, Kitagawa et al. highlighted the importance and challenges of using lymphatic mapping to facilitate staging and prognostication in gastrointestinal cancers[9,15]. This investigation laid the foundation for further exploration of this technology for gastrointestinal cancers. In 2003, Kato et al. investigated lymphatic mapping with technetium-99m colloidal rhenium sulfide in patients with esophageal squamous cell carcinoma scheduled to undergo radical esophagectomy (n = 25)[10]. Technetium-99m colloidal rhenium sulfide was injected into the submucosa at four sites around the periphery of the primary tumor the day before surgery. This was followed by lymphoscintigraphy and mapping and harvesting of presumed SLNs. Esophagectomy, including regional lymph node dissection, was performed 17 h after injection of technetium-99m. After surgery, the resected lymph nodes were evaluated by cytokeratin staining. The authors concluded that technetium-99m colloid could be used to identify the lymphatic basin and that this was a feasible approach for patients with squamous cell carcinoma of the esophagus. The addition of cytokeratin immunochemistry increased the accuracy of SLN identification.
In a similar study from Yasuda et al., 23 patients with esophageal cancer underwent standard 3-field esophagectomy, and 21 patients with gastric cancer underwent standard D2 lymph node dissection; all patients had no obvious signs of lymph node metastases on preoperative staging and workup[11]. Patients received technetium-99m injection the day before surgery, and SLNs were identified with the aid of an intraoperative gamma probe. Lymph node metastasis was identified in 18 of the 44 patients, of whom 15 had metastatic foci in at least one “hot” node.
The above studies demonstrated that detection of SLNs is feasible in early esophageal cancer[9-11]. Despite these early advances in SLN biopsy for gastrointestinal cancers, the role of SLN mapping and biopsy in esophageal cancer is still considered controversial and poses unique challenges related to the factors stated above. While these and other studies have demonstrated the feasibility of the approach, they have not demonstrated “proof of concept” of the SLN. Importantly, it remains to be established that the SLN is truly reflective of the entire burden of disease and the entire bed of lymph nodes to which esophageal cancer may metastasize.
Esophageal cancer is an aggressive cancer and ranks as the seventh most common cancer and the sixth most deadly cancer worldwide[16]. Lymph node metastasis is an important predictor of long-term survival, even for patients with early-stage esophageal cancer (e.g., T1b), in whom rates of lymph node metastasis are as high as 37%[17-19]. However, predicting the spread of esophageal cancer within the lymphatic network is a daunting task, as evidence has shown that spread across the cervical, thoracic, and abdominal domains is complex, with widespread and multidirectional flow[13,20]. For example, the metastatic lymph node may be found remotely from the primary tumor site, and this is particularly true with increasing depth of tumor invasion and with the additional complexity of skip metastases[12,21,22].
Hagens et al. examined transthoracic esophagectomy using either a 2-field or a 3-field lymphadenectomy[13]. The prevalence and mapping of metastatic lymph nodes in 14 studies (8,952 total patients) were sorted by primary tumor location and histologic subtype [Figure 1]. This review showed that both adenocarcinoma and squamous cell subtypes metastasize to cervical, thoracic, and abdominal lymph nodes, irrespective of the location of the primary tumor. The authors observed that squamous cell cancer of the esophagus was more often described in the literature, compared with esophageal adenocarcinoma, owing in part to the higher prevalence of squamous cell esophageal cancer.
Figure 1. Prevalence of lymph node metastases per tumor location in patients with squamous cell carcinoma. (A) Upper thoracic esophageal tumor; (B) Middle thoracic esophageal tumor; (C) Distal thoracic esophageal tumor. Data presented as percentage of patients with lymph node metastases in this region. Reproduced with permission[13].
THE RELEVANCE OF AI IN THE MANAGEMENT OF ESOPHAGEAL CANCER
Recently, AI and big data analytics have been applied to SLN biopsy. In one application of these technologies, deep machine learning algorithms have been used to aid physician decision making[7,23,24]. For example, these technologies can aid in the prediction of lymph node metastasis and response to treatment and can help detect pathologic abnormalities in resected surgical specimens[25]. The application of such technologies can further decrease required human efforts and may decrease human burden and errors. For example, the sensitivity and specificity of SLN biopsy as currently performed are suboptimal, and the procedure is costly and susceptible to errors. Furthermore, the role that micrometastases play in the course of disease and their prognostic impact remain to be determined.
Liu et al. investigated a deep learning-based AI algorithm called LYmph Node Assistant for the analysis of tissues and the recognition of metastases on whole-slide images. The authors observed an AUC of 99.3% and a sensitivity of 91.9%[26]. Oliver et al. developed and tested multiple machine learning algorithms for the identification of patients with head and neck melanoma with a very low risk of occult lymph node metastasis, with the goal of avoiding the unnecessary and expensive use of SLN biopsy based on NCCN treatment guidelines. Machine learning-directed SLN biopsy demonstrated superior performance (AUC, 0.734 vs. 0.542) and higher specificity (25.8% vs. 11.3%; P < 0.001), compared with SLN biopsy based on NCCN recommendations. However, the false-negative rate of the machine learning-based approach (1.2%) and the approach based on NCCN guidelines (2.7%) were not significantly different (P = 0.26)[27].
Numerous efforts have been made to apply AI technology to enhance the quality of treatment by increasing diagnostic accuracy, reducing interobserver variations, and reducing the burden of human workload in the management of esophageal cancer[7,23]. At present, the use of AI in the management of esophageal cancer has focused on two aspects: (1) diagnosis (in particular, the differentiation between Barrett’s esophagus and esophageal adenocarcinoma) and detection at early stages of esophageal cancer and (2) prediction of outcomes and response to therapy. AI models have been trained with various modalities - including white light imaging, magnifying endoscopy, narrow-band imaging, chromoendoscopy, and intracapillary loop to improve upon standard modalities of endoscopic tissue identification[7,23,24]. A method that uses AI to analyze gene expression profiles, reported by Kan et al., achieved a predictive accuracy of 86% for lymph node metastasis in squamous cell carcinoma[28]. Furthermore, AI approaches based on demographic, clinicopathologic, laboratory, radiologic, and genetic variables can aid in the prediction of survival and response to neoadjuvant chemoradiotherapy and chemotherapy. Such tools can promote the development of optimal treatment strategies tailored to individual patients[29].
THE PRINCIPLE OF SENTINEL NODE NAVIGATION SURGERY
Although multimodal treatment has become a crucial component in the management of esophageal cancer, surgical resection (in particular, esophagectomy) remains the cornerstone of curative care for both early and locally advanced disease[9,30,31]. However, esophagectomy combined with radical lymphadenectomy, whether 2 or 3 fields, is associated with significant morbidity and mortality and poor quality of life, compared with nonradical lymphadenectomy. Recent evidence suggests that lymphadenectomy in appropriately selected patients can result in fewer postoperative complications[32]. At present, endoscopic resection is well established as the treatment of choice for intramucosal carcinomas [T1a lesions (lesions confined to the muscularis mucosae or lamina propria)], which are associated with a low incidence of lymph node metastasis (1%-2%)[33]. However, the role of endoscopic resection as a curative treatment for tumors invading the submucosal layer (T1b lesions) remains controversial, owing to the heterogeneity of data on the risk of lymph node metastasis in these tumors (reported range of 0% to 37%)[17-19,34]. As endoscopic resection of esophageal cancer does not allow for lymph node dissection, the ability to accurately assess the risk of lymph node metastasis is crucial for selecting the optimal therapeutic intervention for a particular patient[35]. On the basis of the available evidence, accurately assessing the risk of lymph node metastases for patients with T1b disease depends on several factors, including the depth of invasion, histologic differentiation, lateral and deep margin status, and the presence of lymphovascular invasion[36,37]. Lesions harboring high-risk features - such as submucosal invasion > 500 μm, positive margin status, lymphovascular invasion, and poor differentiation - carry a high risk of lymph node metastases[17,37].
As recent data have suggested that T1b esophageal cancers have a low rate of lymph node metastasis, the use of SLN mapping in such patients to decrease morbidity has gained interest[38]. In this context, a novel surgical technique called sentinel node navigation surgery (SNNS) has been developed[39,40]. The goals of this approach are to reduce patient morbidity and mortality and improve quality of life by potentially avoiding the need for esophagectomy and thereby sparing the esophagus. SLN mapping in breast cancer and melanoma has been shown to avoid unnecessary lymphadenectomy and associated morbidity[3-5]. As discussed above, the use of SLN mapping in esophageal cancer is an evolving and dynamic field. SNNS is used to identify and evaluate the first lymph node draining the primary tumor[39], thus enabling better clinical staging by achieving a more accurate assessment of the risk of lymph node metastasis. The rationale behind this approach is that confirmation that SLNs are free of cancer cells could enable the omission of radical lymphadenectomy and its attendant morbidity. However, as stated above, acceptance of this approach requires demonstration that SLNs in esophageal cancer accurately reflect the burden of disease and that the related rates of false negatives and false positives are low.
THE EVOLUTION OF SNNS
Künzli et al. performed a pilot study to establish the feasibility and safety of SNSS in patients with early esophageal adenocarcinoma[41]. The day before surgery, patients received a submucosal endoscopic injection of a combined technetium-99m nanocolloid tracer (2 mL) at four quadrants around the endoscopic resection scar or esophageal tumor. Planar lymphoscintigraphy was performed 15 min and 2 h after injection of the radioactive tracer, followed by single-photon emission computed tomography/computed tomography to anatomically locate the SLNs. During esophagectomy, the SLNs were detected using an endoscopic gamma probe. After the SLNs were removed, the esophagus and peritumoral lymph nodes were resected by minimally invasive esophagectomy. The median number of retrieved SLNs during SNNS was three, and there was a high concordance between preoperative imaging and intraoperative detection. However, one positive non-SLN was not detected by the intraoperative tracer because of the “shine-through effect,” which was considered a limitation of the radioactive tracer method[41]. Consequently, Overwater
Frederiks et al. investigated the utility and safety of radical endoscopic resection followed by SNNS using a hybrid technetium-99m nanocolloid and indocyanine green tracer in patients with high-risk T1b esophageal carcinoma, with the goal of identifying patients who can safely forgo esophagectomy. In their cohort of 10 patients, the median number of SLNs identified was three (range, 1 to 6). There was a strong concordance observed between preoperative imaging and intraoperative findings. Two patients (20%) had metastasis or micrometastasis detected in a resected SLN. On the basis of findings from SNSS, esophagectomy was not indicated for any of the patients. This work demonstrates a new paradigm for an esophagus-sparing approach and the potential for SNNS to guide decision making for patients with early high-risk T1 esophageal cancers. However, further research is necessary to determine the long-term efficacy and consequences of this treatment approach[42].
NEOADJUVANT CHEMOTHERAPY AND RADIATION TREATMENT
The issue of chemoradiotherapy in SNNS remains challenging as the accuracy of SLN mapping in patients undergoing neoadjuvant therapies remains a matter of debate. Uenosono et al. found that only 45% of patients who underwent neoadjuvant CRT for esophageal cancer had an SLN identified[43]. Thus, they concluded that only early-stage, T1, N0 esophageal cancers are appropriate for SNNS. In contrast, Thompson et al. found no significant differences in the identification of SLNs between patients who received neoadjuvant therapy and those who did not[44]. In a systemic review that included 23 studies, Nagajara et al. further assessed the viability and precision of radioguided SLN mapping for esophageal cancer[14]. In cases of squamous cell carcinoma, the detection rate was 0.89, sensitivity was 0.91, and accuracy was 0.84. The authors concluded that identifying and retrieving an SLN before neoadjuvant therapy in esophageal cancer is feasible. However, they emphasized the importance of optimizing radiocolloid type, refining techniques, and developing methods for efficiently determining SLN status intraoperatively. Furthermore, many of the studies in their analysis had a small sample size, and the results must therefore be interpreted with caution.
FUTURE DIRECTIONS OF SLN IN ESOPHAGEAL CANCER
Future research into the application of SLN biopsy in esophageal cancer should strive to establish a standardized protocol for its use that includes elements such as dosage, timing, duration, and methodology for detection. Further research is needed to determine the long-term effects on outcomes and survival resulting from the omission of esophagectomy with radical lymphadenectomy in patients with T1b esophageal cancer with high-risk features. Although the studies discussed here had small sample sizes, they were among the first to investigate a standardized approach that features SLN biopsy in the context of early esophageal cancer. It remains to be seen whether SLN can be applied to more advanced-stage disease beyond T1 esophageal cancer, and to patients who have received neoadjuvant chemoradiation.
CONCLUSIONS
In summary, the use of SLN biopsy in esophageal cancer continues to evolve. Although its use is not yet in the mainstream, it clearly holds promise to decrease morbidity while achieving better prognostication and outcomes for patients with esophageal cancer. It is also clear that AI tools have a role to play in SLN assessment and can improve efficiency, accuracy, and outcomes. Surgeons must exercise prudent judgment, achieve mastery, and use these tools with discretion until their efficacy and reliability are fully established.
DECLARATIONS
Author contributions
Performed a literature review, wrote and revised the manuscript: Qureshi AP
Helped revise the manuscript: Chobarporn T
Made substantial contributions to the conception and design of the study and edited the manuscript: Molena D
Availability of data and materials
Not applicable.
Financial support and sponsorship
Molena D’s work is supported, in part, by the National Institutes of Health/National Cancer Institute (P30 CA008748 to Memorial Sloan Kettering Cancer Center).
Conflicts of interest
Molena D serves on a steering committee for AstraZeneca; as a consultant for Johnson & Johnson, Bristol-Myers Squibb, AstraZeneca, and Boston Scientific; and has been an invited speaker for Merck and Genentech. The other authors declare that there are no conflicts of interest.
Ethical approval and consent to participate
Not applicable.
Consent for publication
Not applicable.
Copyright
© The Author(s) 2024.
REFERENCES
1. Morrow M, Van Zee KJ, Patil S, et al. Axillary dissection and nodal irradiation can be avoided for most node-positive Z0011-eligible breast cancers: a prospective validation study of 793 patients. Ann Surg 2017;266:457-62.
2. Giuliano AE. The evolution of sentinel node biopsy for breast cancer: personal experience. Breast J 2020;26:17-21.
3. Morton DL, Thompson JF, Cochran AJ, et al; MSLT Group. Final trial report of sentinel-node biopsy versus nodal observation in melanoma. N Engl J Med 2014;370:599-609.
4. Veronesi U, Paganelli G, Viale G, et al. Sentinel-lymph-node biopsy as a staging procedure in breast cancer: update of a randomised controlled study. Lancet Oncol 2006;7:983-90.
5. Krag DN, Anderson SJ, Julian TB, et al. Sentinel-lymph-node resection compared with conventional axillary-lymph-node dissection in clinically node-negative patients with breast cancer: overall survival findings from the NSABP B-32 randomised phase 3 trial. Lancet Oncol 2010;11:927-33.
6. Lyman GH, Giuliano AE, Somerfield MR, et al; American Society of Clinical Oncology. American Society of Clinical Oncology guideline recommendations for sentinel lymph node biopsy in early-stage breast cancer. J Clin Oncol 2005;23:7703-20.
7. Caldonazzi N, Rizzo PC, Eccher A, et al. Value of artificial intelligence in evaluating lymph node metastases. Cancers 2023;15:2491.
8. Peek G, Ross SB. The future implementation of artificial intelligence technology in esophageal surgery. Art Int Surg 2023;3:249-54.
9. Kitagawa Y, Uno T, Oyama T, et al. Esophageal Cancer Practice Guidelines 2017 edited by the Japan Esophageal Society: part 1. Esophagus 2019;16:1-24.
10. Kato H, Miyazaki T, Nakajima M, et al. Sentinel lymph nodes with technetium-99m colloidal rhenium sulfide in patients with esophageal carcinoma. Cancer 2003;98:932-9.
11. Yasuda S, Shimada H, Chino O, et al. Sentinel lymph node detection with Tc-99m tin colloids in patients with esophagogastric cancer. Jpn J Clin Oncol 2003;33:68-72.
12. Prenzel KL, Bollschweiler E, Schröder W, et al. Prognostic relevance of skip metastases in esophageal cancer. Ann Thorac Surg 2010;90:1662-7.
13. Hagens ERC, van Berge Henegouwen MI, Gisbertz SS. Distribution of lymph node metastases in esophageal carcinoma patients undergoing upfront surgery: a systematic review. Cancers 2020;12:1592.
14. Nagaraja V, Eslick GD, Cox MR. Sentinel lymph node in oesophageal cancer-a systematic review and meta-analysis. J Gastrointest Oncol 2014;5:127-41.
15. Kitagawa Y, Fujii H, Mukai M, et al. The role of the sentinel lymph node in gastrointestinal cancer. Surg Clin North Am 2000;80:1799-809.
16. Sung H, Ferlay J, Siegel RL, et al. Global Cancer Statistics 2020: GLOBOCAN estimates of incidence and mortality worldwide for 36 cancers in 185 countries. CA Cancer J Clin 2021;71:209-49.
17. Manner H, Wetzka J, May A, et al. Early-stage adenocarcinoma of the esophagus with mid to deep submucosal invasion (pT1b
18. Gockel I, Sgourakis G, Lyros O, et al. Risk of lymph node metastasis in submucosal esophageal cancer: a review of surgically resected patients. Expert Rev Gastroenterol Hepatol 2011;5:371-84.
19. Newton AD, Predina JD, Xia L, et al. Surgical management of early-stage esophageal adenocarcinoma based on lymph node metastasis risk. Ann Surg Oncol 2018;25:318-25.
20. Yang Y, Li Y, Qin J, et al. Mapping of lymph node metastasis from thoracic esophageal cancer: a retrospective study. Ann Surg Oncol 2022;29:5681-8.
21. Hosch SB, Stoecklein NH, Pichlmeier U, et al. Esophageal cancer: the mode of lymphatic tumor cell spread and its prognostic significance. J Clin Oncol 2001;19:1970-5.
22. Feith M, Stein HJ, Siewert JR. Pattern of lymphatic spread of Barrett’s cancer. World J Surg 2003;27:1052-7.
23. Guidozzi N, Menon N, Chidambaram S, Markar SR. The role of artificial intelligence in the endoscopic diagnosis of esophageal cancer: a systematic review and meta-analysis. Dis Esophagus 2023;36:doad048.
24. Gómez B, Milla Collado L, Rodríguez M. Artificial intelligence in esophageal cancer diagnosis and treatment: where are we now? - a narrative review. Ann Transl Med 2023;11:353.
25. Cykowska A, Marano L, D’Ignazio A, et al. New technologies in breast cancer sentinel lymph node biopsy; from the current gold standard to artificial intelligence. Surg Oncol 2020;34:324-35.
26. Liu Y, Kohlberger T, Norouzi M, et al. Artificial intelligence-based breast cancer nodal metastasis detection: insights into the black box for pathologists. Arch Pathol Lab Med 2019;143:859-68.
27. Oliver JR, Karadaghy OA, Fassas SN, Arambula Z, Bur AM. Machine learning directed sentinel lymph node biopsy in cutaneous head and neck melanoma. Head Neck 2022;44:975-88.
28. Kan T, Shimada Y, Sato F, et al. Prediction of lymph node metastasis with use of artificial neural networks based on gene expression profiles in esophageal squamous cell carcinoma. Ann Surg Oncol 2004;11:1070-8.
29. Zhang YH, Guo LJ, Yuan XL, Hu B. Artificial intelligence-assisted esophageal cancer management: now and future. World J Gastroenterol 2020;26:5256-71.
30. Obermannová R, Alsina M, Cervantes A, et al; ESMO Guidelines Committee. Oesophageal cancer: ESMO Clinical Practice Guideline for diagnosis, treatment and follow-up. Ann Oncol 2022;33:992-1004.
31. Ajani JA, D’Amico TA, Bentrem DJ, et al. Esophageal and esophagogastric junction cancers, version 2.2023, NCCN clinical practice guidelines in oncology. J Natl Compr Canc Netw 2023;21:393-422.
32. van der Werf LR, Busweiler LAD, van Sandick JW, van Berge Henegouwen MI, Wijnhoven BPL; Dutch Upper GI Cancer Audit (DUCA) group. Reporting national outcomes after esophagectomy and gastrectomy according to the Esophageal Complications Consensus Group (ECCG). Ann Surg 2020;271:1095-101.
33. Dunbar KB, Spechler SJ. The risk of lymph-node metastases in patients with high-grade dysplasia or intramucosal carcinoma in Barrett’s esophagus: a systematic review. Am J Gastroenterol 2012;107:850-62.
34. Nieuwenhuis EA, van Munster SN, Meijer SL, et al; Dutch Barrett Expert Centers. Analysis of metastases rates during follow-up after endoscopic resection of early “high-risk” esophageal adenocarcinoma. Gastrointest Endosc 2022;96:237-47.e3.
35. Kamarajah SK, Phillips AW, Hanna GB, Low DE, Markar SR. Is local endoscopic resection a viable therapeutic option for early clinical stage T1a and T1b esophageal adenocarcinoma? Ann Surg 2022;275:700-5.
36. Altorki NK, Lee PC, Liss Y, et al. Multifocal neoplasia and nodal metastases in T1 esophageal carcinoma: implications for endoscopic treatment. Ann Surg 2008;247:434-9.
37. Künzli HT, Belghazi K, Pouw RE, et al. Endoscopic management and follow-up of patients with a submucosal esophageal adenocarcinoma. United European Gastroenterol J 2018;6:669-77.
38. Schölvinck D, Künzli H, Meijer S, et al. Management of patients with T1b esophageal adenocarcinoma: a retrospective cohort study on patient management and risk of metastatic disease. Surg Endosc 2016;30:4102-13.
39. Takeuchi H, Kitagawa Y. Sentinel node navigation surgery in esophageal cancer. Ann Gastroenterol Surg 2019;3:7-13.
40. Overwater A, Weusten BLAM, Ruurda JP, et al. Feasibility of sentinel node navigated surgery in high-risk T1b esophageal adenocarcinoma patients using a hybrid tracer of technetium-99 m and indocyanine green. Surg Endosc 2022;36:2671-9.
41. Künzli HT, van Berge Henegouwen MI, Gisbertz SS, et al. Pilot-study on the feasibility of sentinel node navigation surgery in combination with thoracolaparoscopic lymphadenectomy without esophagectomy in early esophageal adenocarcinoma patients. Dis Esophagus 2017;30:1-8.
42. Frederiks CN, Overwater A, Bergman JJGHM, et al. Feasibility and safety of tailored lymphadenectomy using sentinel node-navigated surgery in patients with high-risk T1 esophageal adenocarcinoma. Ann Surg Oncol 2023;30:4002-11.
43. Uenosono Y, Arigami T, Yanagita S, et al. Sentinel node navigation surgery is acceptable for clinical T1 and N0 esophageal cancer. Ann Surg Oncol 2011;18:2003-9.
Cite This Article
Export citation file: BibTeX | EndNote | RIS
OAE Style
Qureshi AP, Chobarporn T, Molena D. Evolution of the treatment of esophageal cancer: artificial intelligence and the role of sentinel lymph node assessment in esophageal cancer. Art Int Surg 2024;4:68-76. http://dx.doi.org/10.20517/ais.2023.37
AMA Style
Qureshi AP, Chobarporn T, Molena D. Evolution of the treatment of esophageal cancer: artificial intelligence and the role of sentinel lymph node assessment in esophageal cancer. Artificial Intelligence Surgery. 2024; 4(2): 68-76. http://dx.doi.org/10.20517/ais.2023.37
Chicago/Turabian Style
Alia P. Qureshi, Thitiporn Chobarporn, Daniela Molena. 2024. "Evolution of the treatment of esophageal cancer: artificial intelligence and the role of sentinel lymph node assessment in esophageal cancer" Artificial Intelligence Surgery. 4, no.2: 68-76. http://dx.doi.org/10.20517/ais.2023.37
ACS Style
Qureshi, AP.; Chobarporn T.; Molena D. Evolution of the treatment of esophageal cancer: artificial intelligence and the role of sentinel lymph node assessment in esophageal cancer. Art. Int. Surg. 2024, 4, 68-76. http://dx.doi.org/10.20517/ais.2023.37
About This Article
Special Issue
Copyright
Data & Comments
Data
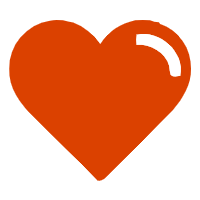
Comments
Comments must be written in English. Spam, offensive content, impersonation, and private information will not be permitted. If any comment is reported and identified as inappropriate content by OAE staff, the comment will be removed without notice. If you have any queries or need any help, please contact us at support@oaepublish.com.