Pragmatism in transforming surgical workplace with artificial intelligence
ARTIFICIAL INTELLIGENCE AS A PARADIGM SHIFT IN SURGERY
On March 10th, 2016, Google’s DeepMind developed the AlphaGo software which defeated the best human Go player with a mindboggling victory. This marked the beginning of popular trust and interest in artificial intelligence (AI)[1]. The evolving gains of machine learning and deep learning technology in recent years have led to an escalating awareness and adoption of AI in all sectors such as business, entertainment, manufacturing, and commerce. Healthcare has seen increasing numbers of startups and ventures in AI initiative recently with projected valuation at USD 45 billion by 2026[2].
AI is an overlapping domain that consists of data science (e.g., data mining and data analysis), mathematics (mainly statistics), and computer science (e.g., machine learning, deep learning, etc.). By definition AI is the ability of computer programs to execute task with human-like reasoning[3]. The four important domains which interplay in its applications include:
1. Machine learning: It is the backbone of AI technology; it is a learning algorithm built on an intelligence model based on existing data, to make predictions or decisions without being explicitly been programmed to perform the task[4,5].
2. Deep learning: The most intriguing subfield of machine learning, which creates a learning model which mimics the human neural network; it is complex, convoluted, and multilayered. Common examples of deep learning in computer applications include convolution neural network and deep reinforcement learning[4,5].
3. Computer vision: This field enables visual data interpretation for a meaningful recommendation by a computer system[6].
4. Natural language processing: A subfield of machine learning which deals with technology which enables computers to understand and process human language[7].
With the passage of time, AI has become a necessity for efficient utilization of the increasing load of digital data pool (i.e., big data) in healthcare[8-10]. One use of AI in healthcare is in the field of surgery; AI applications have the potential to reduce redundancy in surgeon’s daily workload[11]. On par with the increasing life expectancy of global population[12], AI can fulfill the increasing demands required to provide more comprehensive and complex surgical care.
ADOPTING AI INTO SURGICAL WORKPLACE
“Change is the only constant in life” is a tangible truth which applies in all aspect of human lives. AI will revamp medical practice in the near future, just as any unequivocally beneficial technology has done in prior years. AI in surgery is a booming industry, but not all industry driven technology will be translated into working clinical applications. The process of adopting this technology should ensure unequivocal benefit to both patients and surgeons.
A pragmatic multiphase effort, with achievable transformation goals for incremental adaptation and introduction of AI in surgical practice, is essential for sustainability[13]. AI technologies should be initially adapted to address simple and repetitive administrative tasks. This optimizes time for surgeons who spend an estimated 70% of their time on non-operative duties[14]. Besides increasing work efficiency for surgeons, this approach will ensure voluntary engagement and likeability for AI technologies by staffs and hence, increasing adoption.
As surgeons acclimatize to this new technology, hospital- and home-based care such as virtual based assistance and remote monitoring can be introduced subsequently. A deeper integration of AI into workflow and practice will ensure smooth and gradual engagement of surgeons and patients with this new technology. Both technology advancement (e.g., deep learning, natural language processing, networking, wearable devices, and etc.) and cultural adaption within a surgical workplace is vital in this phase[15].
Ultimately, as surgeons realize the full capability of AI in a surgical workplace, AI technology will be seen as a need and integral part of surgical practice. At this pinnacle, AI based surgical care would have been trained in numerous clinical trials to a near perfect accuracy and would have overcome gaps in ethics, interpretability, and interoperability[16].
AN EFFICIENT SURGICAL WORKPLACE WITH AI
Electronic health record (EHR) was introduced to reduce medical errors and to increase quality of care by facilitating communication. Unfortunately, surgeons have expressed distrust and unease with the system. Studies have shown that surgeons find documentation via the EHR incomplete, and takes a longer time to complete than paper-based systems[17,18]. This has led to reduced adoption of EHR in surgical practice.
AI can fill this gap with natural language processing, an AI methodology which understands human language. Natural language processing in combination with machine learning model facilitates the translating and interpretating of clinical data from EHR. Additionally, voice recognition software embedded into natural language processing-machine learning models will be able to document the problems, diagnosis, and procedures of patients through a voice-based commands[19,20]. One such application is SayKara, which was launched in 2017. It has the ability to extract relevant information from conversations, an convert them into written documents[19].
AI enabled EHR has the benefit of reducing administrative fatigue among surgeons and staffs by assisting in efficient billing, claim processing, revenue management, and automated disease coding (ICD codes)[11,21,22]. AI-enabled cloud-based EHR (e.g., Amazon Comprehend Medical, Athenahealth) facilitates data sharing and accessibility of clinical data[22,23]. This cloud-AI interface could overcome the heterogeneity of current EHR systems to improve their interoperability[16].
AI IN ENHANCING PATIENT CARE
Making an accurate diagnosis is an important task for surgeons when it comes to providing optimal patient care. Furthermore, early disease recognition especially in cancers are pertinent for improved patients’ survival[24]. Diagnostic decision support system (DDSS) is an AI designed application which can augment surgeons’ diagnostic process. A DDSS computer engine matches patient-specific assessments to a computerized clinical knowledge database to provide individualised recommendations, which are then presented to the clinician to support decision making[25]. Even though DDSS in surgical practice is in its infancy, clinical trials in dermatology, neurology, and ophthalmology have shown that a DDSS and nonexpert clinician combination made clinical diagnosis at a more accurate rate compared to an expert clinician in each pertaining field[26,27]. Hence, adoption of DDSS into surgical practice is foreseeable in the near future. For a successful adoption of DDSS into surgical practice, a trustworthy and bias free system which was trained with ample clinical data will be required[15,28,29].
Accurate cancer staging is essential in determining if further adjuvant therapy is required for a patient. Adjuvant therapy, be it, chemotherapy, radiotherapy, or targeted therapy, can significantly improve survival and disease-free interval in patients[30-32]. But over-staging a patient will expose them to unnecessary toxic side effects of adjuvant therapy[33,34]. Often more so ever, surgeons face challenges in staging “borderline” cases, obfuscating the disease management. AI algorithms have a potential role in aiding accurate histopathological and clinical staging of cancers. Machine learning algorithms can assist surgeons by incorporating imaging, laboratory genomic, and personal data to select a precise treatment modality and predict response from each treatment modalities[35]. Examples of AI augmented cancer staging, which have showed favorable results, are histopathological detection of cancer cells in sentinel lymph node specimens for breast cancer[36], radiological image assessment for lymph node metastasis in rectal cancers[37,38], and clinical staging of intrahepatic cholangiocarcinoma[39].
The American College of Surgeons National Surgical Quality Improvement Program Surgical Risk Calculator, Revised Cardiac Risk Index, and the Physiology and Operative Severity Score are empirical pre-operative risk prediction models which are used in surgical practice[40-43]. These calculators, which are created using the linear regression technique, have inherent flaws of subjectivity and assumption that risk factors are additive. Hence, they commonly either under- or overestimate the risk[40-43]. AI models can refine the surgeon’s ability to risk stratify patients, as they can better evaluate variables in a nonlinear fashion. One such example is the Predictive OpTimal Trees in Emergency Surgery Risk (POTTER) calculator, an AI algorithm trained to predict postoperative mortality, morbidity, and 18 specific complications. In addition, the POTTER calculator is user-friendly and is suitable for daily bedside use[44].
Early detection and prevention of post-operative complications are of a paramount importance in surgical practice[45]. As with any craftsman, surgeons thrive on good and flawless outcomes. Unfortunately, due to heavy workload, surgeons often delegate patients’ post-operative care to junior team members. To assist them, current linear algorithm-based applications such as the Modified Early Warning System were created to detect post operative complications but they have high false positive rates[46], hence, there has been distrust by health providers. By providing a refined multilayer assessment, AI applications can assist in predicting and assessing for post operative complications. AI algorithms incorporate both clinical data and intra-operative data, to provide an accurate and patient centric prediction of post-operative complications (e.g., prolonged hospital stay and missed intraoperative injuries) for early detection[47].
Implementation of Enhanced Recovery After Surgery for a “value-based surgery” has mounted to earlier discharges (post op day 3-5) after surgery[48,49]. Unfortunately, in the process of enhancing patients’ autonomy in their treatment, the burden of post-operative care shifts on to themselves. Wearable health devices will enable surgeons to remotely monitor patients, for an uninterrupted post-operative monitoring. These devices provide real-time data which can be analyzed by machine learning algorithms to provide remote feedback to surgeons, regarding the patients’ condition[50]. To top it off, this occurs with minimal disruption to patients’ daily lives.
AI FOR INTRAOPERATIVE AUGMENTATION
Advancement in computer vision has enable AI programs to perform automated detection of surgical phases and tracking of instruments[51]. Expansion of these have created applications which can perform automated intra-operative assessments, such as, difficulty assessment by utilising phase recognition (e.g., via surgical fingerprints)[51,52], operative performance assessment by real-time tool tracking[51,52], identification of critical events in surgical procedures (e.g., via haemorrhage)[53], and key anatomy identification (e.g., critical view of safety in cholecystectomy)[54]. During surgery, these applications can provide real-time feedbacks and guidance to ensure safe conduct of the procedure.
Autonomous robotic surgery, which envision surgical robots built with computer vision and deep learning technologies to performance surgical task without the need for surgeons’ assistance[55]. One such prototype is the Smart Tissue Autonomous Robot (STAR), which was designed to perform bowel anastomosis. This system utilizes a 3D visual tracking system and a robotic arm to place sutures for an anastomosis. This is a supervised-autonomous system, whereby it requires surgeons to place pointers at deflection points for guidance. The STAR system was reported to be able to finish the anastomosis in 60 s, which was 4 min faster than a surgeon handled robot[56]. It also delivered a more precise suture placement with equal tension on bites[56]. This “supervised autonomous surgery” lays the stepping stone for a “full-autonomous robotic surgery” which can be expected in the future.
FOR WIDE ADOPTION OF AI INTO SURGICAL WORKPLACE
Surgical education should incorporate teaching modules about fundamentals of AI. This will equip future surgeons, with the essential knowledge to understand and trust the AI technologies. Furthermore, with increasing financial interest in AI technology, more AI applications are expected to be churned out into the market. Inevitably, some of these technologies will have flaws or, may not be suitable for a surgical practice. Hence forth, it is fundamentally necessary for surgeons to master principles of AI, to be able to select appropriate and compatible AI applications, which are to be integrated into their practices.
AI technology does not conform to conventional ethics and confidentiality of current surgical practice. Refining these laws with consideration of data privacy and confidentiality with the 4 core principles of medical ethics, autonomy, justice, beneficence, and non-maleficence, is pertinent. Dawson et al.[57] discussed the following in regard to ethics framework in AI, which can be applied in surgery:
(1) Generation of net benefits to the people that must be greater than the costs that may involve accessing of personal data;
(2) Do no harm in so much as to minimize any negative impact on the civilian population;
(3) Regulatory and legal compliance of federal, state, and territory laws by corporations building and supplying AI systems;
(4) Privacy protection that safeguards personal data from breaches that can result in financial, professional, psychological, and reputational harm;
(5) Fairness to prevent inherent biases due to the use of training data sets that are inadvertently weighted against a race, gender, or socioeconomic group, which result in discrimination and opportunity losses;
(6) Transparency and ease of explaining information as to when the algorithm is used and how it arrives at a decision or prediction;
(7) Contestability where person(s) negatively impacted by algorithm-derived decisions have an efficient avenue to challenge the output;
(8) Accountability of organizations and people responsible for the creation and use of algorithms even when deployed in good faith and with no malicious intent.
CONCLUSION
Surgeons should be optimistic about the integration of AI in surgical workplace. It is time that surgeons embrace the reality regarding AI’s role in shaping future of surgery. This acceptance reaffirms surgeons’ autonomy in practice and establishes surgeons as industrial leaders who will drive the AI in surgery revolution forward.
DECLARATIONS
Authors’ contributionsThe author contributed solely to the article.
Availability of data and materialsNot applicable.
Financial support and sponsorshipNone.
Conflicts of interestThe author declared that there are no conflicts of interest.
Ethical approval and consent to participateNot applicable.
Consent for publicationNot applicable.
Copyright© The Author(s) 2022.
REFERENCES
2. Talby D. The current state of the healthcare AI revolution. Available from: https://www.forbes.com/sites/forbestechcouncil/2021/04/28/the-current-state-of-the-healthcare-ai-revolution/?sh=272adbf2980d [Last accessed on 21 Dec 2021].
3. Kersting K. Machine learning and artificial intelligence: two fellow travelers on the quest for intelligent behavior in machines. Front Big Data 2018;1:6.
4. Janiesch C, Zschech P, Heinrich K. Machine learning and deep learning. Electron Markets 2021;31:685-95.
5. van Ginneken B. Fifty years of computer analysis in chest imaging: rule-based, machine learning, deep learning. Radiol Phys Technol 2017;10:23-32.
6. Esteva A, Chou K, Yeung S, et al. Deep learning-enabled medical computer vision. NPJ Digit Med 2021;4:5.
8. Mathias B, Lipori G, Moldawer LL, Efron PA. Integrating "big data" into surgical practice. Surgery 2016;159:371-4.
9. Hashimoto DA, Rosman G, Rus D, Meireles OR. Artificial intelligence in surgery: promises and perils. Ann Surg 2018;268:70-6.
10. Nagano H. Big data, information and communication technology, artificial intelligence, internet of things: how important are they for gastroenterological surgery? Ann Gastroenterol Surg 2018;2:166.
11. Davenport T, Kalakota R. The potential for artificial intelligence in healthcare. Future Healthc J 2019;6:94-8.
12. Roser M, Ortiz-Ospina E, Ritchie H. Life expectancy. Available from: https://ourworldindata.org/life-expectancy [Last accessed on 21 Dec 2021].
13. Hadley TD, Pettit RW, Malik T, Khoei AA, Salihu HM. Artificial intelligence in global health - a framework and strategy for adoption and sustainability. Int J MCH AIDS 2020;9:121-7.
14. Holzer E, Tschan F, Kottwitz MU, Beldi G, Businger AP, Semmer NK. The workday of hospital surgeons: what they do, what makes them satisfied, and the role of core tasks and administrative tasks; a diary study. BMC Surg 2019;19:112.
15. Liberati EG, Ruggiero F, Galuppo L, et al. What hinders the uptake of computerized decision support systems in hospitals? Implement Sci 2017;12:113.
16. Varghese J. Artificial intelligence in medicine: chances and challenges for wide clinical adoption. Visc Med 2020;36:443-9.
17. Sanders DS, Read-Brown S, Tu DC, et al. Impact of an electronic health record operating room management system in ophthalmology on documentation time, surgical volume, and staffing. JAMA Ophthalmol 2014;132:586-92.
18. Frazee R, Harmon L, Papaconstantinou HT. Surgeons' perspective of a newly initiated electronic medical record. Proc (Bayl Univ Med Cent) 2016;29:21-3.
20. Davenport TH, Hongsermeier TM, Mc Cord KA. Using AI to improve electronic health records. Available from: https://hbr.org/2018/12/using-ai-to-improve-electronic-health-records [Last accessed on 21 Dec 2021].
21. Presti MV. Taking medical coding to the next level with Artificial Intelligence. Available from: https://www.ibm.com/blogs/watson-health/medical-coding-with-ai/ [Last accessed on 21 Dec 2021].
23. Lin WC, Chen JS, Chiang MF, Hribar MR. Applications of artificial intelligence to electronic health record data in ophthalmology. Transl Vis Sci Technol 2020;9:13.
25. Sutton RT, Pincock D, Baumgart DC, Sadowski DC, Fedorak RN, Kroeker KI. An overview of clinical decision support systems: benefits, risks, and strategies for success. NPJ Digit Med 2020;3:17.
26. Bau CT, Chen RC, Huang CY. Construction of a clinical decision support system for undergoing surgery based on domain ontology and rules reasoning. Telemed J E Health 2014;20:460-72.
27. Chang AC. . Chapter 7 - clinician cognition and artificial intelligence in medicine. Intelligence-based medicine. Academic Press; 2020.
28. Jones C, Thornton J, Wyatt JC. Enhancing trust in clinical decision support systems: a framework for developers. BMJ Health Care Inform 2021;28:e100247.
29. Khairat S, Marc D, Crosby W, Al Sanousi A. Reasons for physicians not adopting clinical decision support systems: critical analysis. JMIR Med Inform 2018;6:e24.
30. Kawamura H, Morishima T, Sato A, Honda M, Miyashiro I. Effect of adjuvant chemotherapy on survival benefit in stage III colon cancer patients stratified by age: a Japanese real-world cohort study. BMC Cancer 2020;20:19.
31. Boyne DJ, Cuthbert CA, O'Sullivan DE, et al. Association between adjuvant chemotherapy duration and survival among patients with stage II and III colon cancer: a systematic review and meta-analysis. JAMA Netw Open 2019;2:e194154.
32. Brierley J, Gospodarowicz M, O'Sullivan B. The principles of cancer staging. Ecancermedicalscience 2016;10:ed61.
33. Azim HA Jr, de Azambuja E, Colozza M, Bines J, Piccart MJ. Long-term toxic effects of adjuvant chemotherapy in breast cancer. Ann Oncol 2011;22:1939-47.
34. Galata C, Merx K, Mai S, et al. Impact of adjuvant chemotherapy on patients with ypT0-2 ypN0 rectal cancer after neoadjuvant chemoradiation: a cohort study from a tertiary referral hospital. World J Surg Oncol 2018;16:156.
35. Bera K, Katz I, Madabhushi A. Reimagining t staging through artificial intelligence and machine learning image processing approaches in digital pathology. JCO Clin Cancer Inform 2020;4:1039-50.
36. Dihge L, Ohlsson M, Edén P, Bendahl PO, Rydén L. Artificial neural network models to predict nodal status in clinically node-negative breast cancer. BMC Cancer 2019;19:610.
37. Bedrikovetski S, Dudi-Venkata NN, Kroon HM, et al. Artificial intelligence for pre-operative lymph node staging in colorectal cancer: a systematic review and meta-analysis. BMC Cancer 2021;21:1058.
38. Zheng Q, Yang L, Zeng B, et al. Artificial intelligence performance in detecting tumor metastasis from medical radiology imaging: a systematic review and meta-analysis. EClinicalMedicine 2021;31:100669.
39. Kunstman JW. Artificial intelligence in cancer staging: limitless potential or passing fad? Ann Surg Oncol 2020;27:978-9.
40. Raymond BL, Wanderer JP, Hawkins AT, et al. Use of the american college of surgeons national surgical quality improvement program surgical risk calculator during preoperative risk discussion: the patient perspective. Anesth Analg 2019;128:643-50.
42. Ramesh VJ, Rao GS, Guha A, Thennarasu K. Evaluation of POSSUM and P-POSSUM scoring systems for predicting the mortality in elective neurosurgical patients. Br J Neurosurg 2008;22:275-8.
43. Biccard BM, Rodseth RN. Utility of clinical risk predictors for preoperative cardiovascular risk prediction. Br J Anaesth 2011;107:133-43.
44. Bertsimas D, Dunn J, Velmahos GC, Kaafarani HMA. Surgical risk is not linear: derivation and validation of a novel, user-friendly, and machine-learning-based predictive optimal trees in emergency surgery risk (POTTER) calculator. Ann Surg 2018;268:574-83.
45. Thompson JS, Baxter BT, Allison JG, Johnson FE, Lee KK, Park WY. Temporal patterns of postoperative complications. Arch Surg 2003;138:596-602; discussion 602-3.
46. Stenhouse C, Coates S, Tivey M, Allsop P, Parker T. Prospective evaluation of a modified Early Warning Score to aid earlier detection of patients developing critical illness on a general surgical ward. Br J Anaesth 2000;84:663P.
47. Bian Y, Xiang Y, Tong B, Feng B, Weng X. Artificial intelligence-assisted system in postoperative follow-up of orthopedic patients: exploratory quantitative and qualitative study. J Med Internet Res 2020;22:e16896.
48. Melnyk M, Casey RG, Black P, Koupparis AJ. Enhanced recovery after surgery (ERAS) protocols: time to change practice? Can Urol Assoc J 2011;5:342-8.
49. Ljungqvist O, Scott M, Fearon KC. Enhanced recovery after surgery: a review. JAMA Surg 2017;152:292-8.
50. Amin T, Mobbs RJ, Mostafa N, Sy LW, Choy WJ. Wearable devices for patient monitoring in the early postoperative period: a literature review. Mhealth 2021;7:50.
52. Chadebecq F, Vasconcelos F, Mazomenos E, Stoyanov D. Computer vision in the surgical operating room. Visc Med 2020;36:456-62.
53. Garcia-Martinez A, Vicente-Samper JM, Sabater-Navarro JM. Automatic detection of surgical haemorrhage using computer vision. Artif Intell Med 2017;78:55-60.
54. Mascagni P, Vardazaryan A, Alapatt D, et al. Artificial intelligence for surgical safety: automatic assessment of the critical view of safety in laparoscopic cholecystectomy using deep learning. Ann Surg 2020; doi: 10.1097/SLA.0000000000004351.
55. Attanasio A, Scaglioni B, De Momi E, Fiorini P, Valdastri P. Autonomy in surgical robotics. Annu Rev Control Robot Auton Syst 2021;4:651-79.
56. Leonard S, Wu KL, Kim Y, Krieger A, Kim PC. Smart tissue anastomosis robot (STAR): a vision-guided robotics system for laparoscopic suturing. IEEE Trans Biomed Eng 2014;61:1305-17.
57. Dawson D, Schleiger E, Horton J, et al. Artificial intelligence: Australia’s ethics framework - a discussion paper. Available from: https://apo.org.au/node/229596 [Last accessed on 21 Dec 2021].
Cite This Article
Export citation file: BibTeX | EndNote | RIS
OAE Style
Tamalvanan V. Pragmatism in transforming surgical workplace with artificial intelligence. Art Int Surg 2022;2:1-7. http://dx.doi.org/10.20517/ais.2021.09
AMA Style
Tamalvanan V. Pragmatism in transforming surgical workplace with artificial intelligence. Artificial Intelligence Surgery. 2022; 2(1): 1-7. http://dx.doi.org/10.20517/ais.2021.09
Chicago/Turabian Style
Vethunan Tamalvanan. 2022. "Pragmatism in transforming surgical workplace with artificial intelligence" Artificial Intelligence Surgery. 2, no.1: 1-7. http://dx.doi.org/10.20517/ais.2021.09
ACS Style
Tamalvanan, V. Pragmatism in transforming surgical workplace with artificial intelligence. Art. Int. Surg. 2022, 2, 1-7. http://dx.doi.org/10.20517/ais.2021.09
About This Article
Copyright
Data & Comments
Data
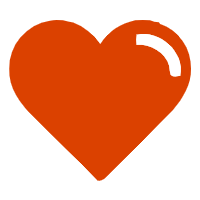
Comments
Comments must be written in English. Spam, offensive content, impersonation, and private information will not be permitted. If any comment is reported and identified as inappropriate content by OAE staff, the comment will be removed without notice. If you have any queries or need any help, please contact us at support@oaepublish.com.