Is artificial intelligence the new kid on the block? Sustainable applications in cardiology
Abstract
Artificial intelligence (AI) is changing our clinical practice. This is particularly true in cardiology where the clinician is often required to handle a large amount of clinical, biological, and imaging data during decision making. In this context, AI can address the need for fast and accurate tools while reducing the burden on clinicians and improving the efficiency of healthcare systems. With this inevitable shift towards more automated and efficient systems, patients may benefit from a more accurate diagnosis and more tailored treatment. A multitude of clinical applications have already been made available and implemented in several fields of cardiology. The aim of this narrative review is to provide an overall picture of the most recent evidence in the literature about AI implementations, highlighting their potential impact on clinical practice.
Keywords
INTRODUCTION
The advent of artificial intelligence (AI) has already dramatically changed almost all aspects of our daily lives. Medicine and cardiovascular health are also concerned, with significant resources spent in the last decades to boost research on the development and implementation of novel AI tools in this field. The goal is to assist cardiologists in their practice, reducing the pressure deriving from the large amount of data and tasks they are required to handle, overall improving their efficiency. Artificial intelligence will benefit the patients first by providing more personalized and effective care. The scenario of the available applications of AI and machine learning (ML) tools is constantly changing [Figure 1]. This narrative review provides an overall picture of current and potential applications of AI in the cardiovascular (CV) field.
Figure 1. Implementation of artificial intelligence in clinical practice. Starting from clinical hypothesis and access to large clinical datasets, AI-based models can assist in diagnosis and provide prognostic assessment with several clinical applications. This, in turn, has an impact on clinical decisions and could modify clinical outcomes with overall improved patient care. AI constantly learns from data, with continuous optimization and improvement of analytic models.
CARDIOVASCULAR RISK ASSESSMENT
Cardiovascular prevention is based on the recognition and control of several factors associated with a disease or clinical condition defined as risk factors, including age, sex, smoking, body mass index (BMI), hypertension, and diabetes mellitus (DM)[1].
In addition, CV prevention should integrate non-traditional risk markers, CV imaging data, and in the future, genomics, proteomics, lipidomics, and polygenic risk score (PRS). Multiple blood-borne biomarkers, such as microRNAs (miRNAs), have emerged as powerful regulators of cellular processes and, due to their biological characteristics, can be easily measured by non-invasive testing. Numerous studies have established their role as new diagnostic and/or prognostic markers for the prevention and stratification of CV risk[2]. Similarly, multi-omics data providing information about molecular pathways involved in several diseases by measuring the expression of proteins or genes are promising candidates for predicting CV risk[3].
Artificial intelligence can be used to develop models able to combine and process a large amount of existing clinical data with imaging and multi-omics data to make automated predictions of scores in the CV risk assessment, leading to the identification of new diagnostic and prognostic markers[4]. For instance, a machine learning (ML) model was used to classify endocrine hypertension subtypes using multidimensional omics analysis of plasma and urine samples. This MOmics approach, compared to the analysis of mono-omics data, enables more sensitive and specific discrimination among various forms of endocrine hypertension[5]. Moreover, using an AI model generated a new coronary artery disease (CAD) risk score able to identify patients with CAD on the basis of the identification of some sphingolipid species. Due to their different biosynthetic pathways, plasma levels of sphingolipids can, in fact, independently predict CV risk. This model, based on the combination of AI-derived sphingolipid risk scores and LDL-C values, provided a more accurate risk assessment than the singular biomarkers[6].
In another study, ML models implemented the circulating profile of two miRNAs (miR-186-5p and
ELECTROCARDIOGRAPHY AND ARRHYTHMOLOGY
An electrocardiogram (ECG) is a widely available non-invasive tool used for the diagnosis of several heart diseases including arrhythmias, atrioventricular conduction disease, and acute coronary syndrome[8]. It has been estimated that a huge amount of information embedded in ECG tracings is not used by the traditional interpretation systems, while its widespread adoption in medicine - estimated at over 300 million exams every year-globally determines a significant workload for physicians[9]. To improve the workflow, a computer-aided interpretation of ECG data was introduced 50 years ago. However, commercial algorithms for ECG interpretation continue to show important rates of misdiagnosis[10-12]. In the last years, substantial improvements have been driven largely by a specific class of AI models known as deep neural networks (DNNs). The performance of DNNs tends to improve as the amount of training data increases, making widespread digitization of ECG data a great advantage[13].
Artificial intelligence algorithms have also been proved to be able to recognize different types of arrhythmias with performances comparable to those of expert cardiologists. In addition, AI models have been developed to detect structural heart disease, perform risk stratification, and execute ECG signal processing, improving its quality and diagnostic accuracy[14-19]; see Table 1.
Main achievements with AI in the field of ECG
Study | Year | Sensor | Population/dataset | Principal findings |
Smith et al.[14] | 2019 | 12-Lead ECG | 500 samples | A DNN algorithm improved the interpretation of AF |
Liu et al.[15] | 2023 | 12-Lead ECG | 952 patients | An AI model outperformed ECG criteria for detecting LVH |
Attia et al.[16] | 2019 | 12-Lead ECG | 97,829 patients | An AI-enabled ECG has been proposed as a screening tool in asymptomatic individuals to identify ALVD |
Attia et al.[17] | 2019 | 12-Lead ECG | 649,931 samples from 180,922 patients | An AI-enabled ECG acquired during normal sinus rhythm allows identification of patients with AF |
Raghunath et al.[18] | 2020 | 12-Lead ECG | 1,169,662 samples from 253,397 patients | A DL approach provided prognostic information to the interpretation of ECG |
Poungponsri et al.[19] | 2013 | 2-Lead ECG | MIT-BIH arrhythmia database MIT-BIH noise stress test database | An adaptive filtering approach based on discrete wavelet transform and ANN can successfully remove various noise and artifacts in ECG signals |
Jadhav et al.[20] | 2010 | 12-Lead ECG | 452 patients | An ANN based system performed cardiac arrhythmia classification using ECG signal data |
Acharya et al.,[21] | 2017 | 1-Lead ECG | 109,449 samples | A CNN identified and classified the different types of ECG heartbeats |
Haseena et al.[22] | 2011 | 2-Lead ECG | MIT-BIH arrhythmia database | An ANN based system performed cardiac arrhythmia classification using ECG signal data |
Sayantan et al.[23] | 2018 | 2-Lead ECG | MIT-BIH arrhythmia database MIT-BIH supra-ventricular arrhythmia database | A CNN identified and classified the different types of ECG heartbeats |
Tateno et al.[24] | 2001 | 2-Lead ECG | MIT-BIH AF database MIT-BIH arrhythmia database | An AI model allowed automatic detection of AF |
Dash et al.[25] | 2009 | 2-Lead ECG | MIT-BIH AF database MIT-BIH arrhythmia database | An AI model allowed automatic detection of AF |
Huang et al.[26] | 2011 | 3-Lead ECG 2-Lead ECG | A dataset of 24-h Holter ECG recordings (n = 433) MIT-BIH AF database MIT-BIH NSR database | An AI model allowed automatic detection of AF |
Babaeizadeh et al.[27] | 2009 | 2-Lead ECG | A dataset of 24-h Holter ECG recordings (n = 633) MIT-BIH AF database | An AI model allowed automatic detection of AF |
Jiang et al.[28] | 2012 | 3-Lead ECG 2-Lead ECG | ECG Department of the Sir Run Run Shaw Hospital and the First Affiliated Hospital, School of Medicine, Zhejiang University, Chin database (n = 45 24-Holter ECG recordings) MIT-BIH AF database | An AI model allowed automatic detection of AF |
Xia et al.[29] | 2018 | 2-Lead ECG | MIT-BIH AF database | An AI model allowed automatic detection of AF |
Acharya et al.[30] | 2018 | 2-Lead ECG 1-Lead ECG | MIT-BIH arrhythmia database MIT-BIH malignant ventricular arrhythmia database Creighton University ventricular tachyarrhythmia database | An AI model identified shockable ECG signals |
Sabut et al.[31] | 2021 | ECG sensors | CUDB and VFDB databases of the PhysioNet repository | DNN permitted identification VA episodes |
Chang et al.[32] | 2021 | 12-Lead ECG | 60,537 samples from 35,981 patients | An AI model allowed detection both rhythm classes and acute STEMI |
Zheng et al.[33] | 2022 | 12-Lead ECG | 18,612 samples from 545 patients | An ML model precisely predicted the correct origins of idiopathic ventricular arrhythmia |
Raghunath et al.[34] | 2021 | 12-Lead ECG | 1.6 million samples from 431,000 patients | A DL model predicted new-onset AF from the 12-lead ECG in patients with no previous history of AF |
Hsu et al.[35] | 2022 | 12-Lead ECG | 2,206 participants | An ML model predicted LAE in young adults using ECG and biological features |
Zhao et al.[36] | 2022 | 12-Lead ECG | 1,863 patients | A CNN model detected LVH by 12-lead ECG with higher sensitivity than current ECG diagnostic criteria |
Grogan et al.[37] | 2021 | 12-Lead ECG | 2,541 patients | AI-enabled ECG effectively detected cardiac amyloidosis and may promote early diagnosis |
Kwon et al.[38] | 2019 | 12-Lead ECG | 55,163 samples from 22,765 patients | A DL model accurately identified HF using ECG features |
Dey et al.[40] | 2021 | 12-Lead ECG | PTB database | A DL model detected MI |
Jahmunah et al.[41] | 2021 | 1-Lead ECG | Fantasia and St. Petersburg databases | A CNN model allowed automatic categorization of normal, CAD, MI and CHF classes using ECG signals |
Yadav et al.[42] | 2021 | 12-Lead ECG | PTB diagnostic database | A CNN model detected MI |
Hasbullah et al.[43] | 2023 | 12-Lead ECG | PTB-XL Database | A CNN model differentiated MI from the healthy class and the other CVD class |
Xiong et al.[44] | 2021 | 12-Lead ECG | PTB database | An AI model accurately localized MI |
Ibrahim et al.[45] | 2020 | 12-Lead ECG | ECG-ViEW II database | An AI model predicted AMI from 12-Lead ECG |
Gustafsson et al.[46] | 2022 | 12-Lead ECG | 492,226 samples from 214,250 patients | A DL model discriminated between control, STEMI, and NSTEMI on the presenting ECG of a real-world sample (all-comers to the emergency department) |
Fiorina et al.[47] | 2022 | 3-Lead ECG | 1,000 samples | A DL approach classified a broad range of distinct arrhythmias from ambulatory ECGs with high diagnostic performance |
Initially, researchers focused on the creation of models able to distinguish normal patterns from abnormal ones; in one of the earliest studies in this field, a neural network (NN) with static backpropagation algorithms was used to classify ECG signals into normal and abnormal subgroups, with an accuracy of 86.7%[20]. Progressively, the performance and output complexity of these AI models has significantly increased, enabling not only the detection of abnormal ECGs but also further sub-classification of arrhythmias in different categories[21-23]. A subject of particular interest in this field was atrial fibrillation (AF) detection. Many algorithms have been proposed for this scope; some of these are based only on RR interval detection[24-26], while others need detection of both RR intervals and P wave[27,28]. Therefore, they rely on the accuracy of peak detection, with poor or reduced performance in case of missing or erroneously detected peaks. An alternative method[29] converted the one-dimensional ECG signal into two-dimensional form by short-time Fourier and stationary wavelet transform, respectively, with no need for peak detection and outperforming the majority of the existing algorithms.
Even in the case of life-threatening arrhythmias, AI models have shown excellent performance[30]. An innovative method based on hybrid time-frequency features and deep learning (DL) has been proposed for the detection of VA episodes; compared with the previous systems, it was superior in detecting shockable VTAs, with a sensitivity, specificity, and accuracy of 99%[31].
As already pointed out, the accuracy of AI models is, in some areas, comparable, if not superior, to those of experienced physicians. For instance, an AI model showed an accuracy for the classification of 12 heart rhythms superior to that of internists, emergency physicians, and even cardiologists[32]. In addition to classifying arrhythmias, AI models can further characterize them. For instance, a ML algorithm was able to precisely predict the origin of idiopathic VA with an accuracy of 98%[33].
A significant advantage of AI models is that they can also automatically extract information that usually sits below the level of human detection. In Attia et al.’s study[17], an AI-enabled ECG acquired during normal sinus rhythm allowed the identification of individuals with paroxysmal AF with an accuracy of 83%. In another study, a neural network (NN) was trained on 1.6 million ECGs to predict new-onset AF within
Deep learning ECG-based algorithms could also have a central role in the early diagnosis of heart failure (HF). Researchers have developed and validated a DL algorithm, using data from over 50,000 ECGs, that was superior in detecting HF with reduced ejection fraction (HFrEF) compared to logistic regression[38]. Moreover, AI algorithms can identify patients with asymptomatic left ventricular dysfunction (ALVD)[16], a condition associated with reduced quality of life (QoL) and longevity and present in about 3%-6% of the general population[39].
Beyond diagnosis, several prognostic applications have also been described; a DNN was, in fact, capable of predicting 1-year all-cause mortality from ECG voltage-time traces[18] .
Furthermore, studies in which AI systems showed the capacity to detect and classify[40-43], localize[44] or even predict myocardial infarction[45] have also been published. An AI model has shown excellent results in classifying between control, ST elevation myocardial infarction, and non-ST elevation myocardial infarction in a real-world sample of all-comers to an emergency department[46].
Artificial intelligence can also be finally used to improve technical aspects. Researchers proposed an adaptive filtering technique based on wavelet transform and artificial NN that can successfully remove different noise and artifacts in ECG signals[19].
Holter ECG analysis can also benefit from a broader use of AI models. A DNN model proved to be non-inferior and faster compared to the conventional Holter ECG analysis performed by electrophysiologists in the detection of major rhythm abnormalities[47]. Single-lead ECG wearables and smartwatches are nowadays increasingly used[48,49]. These systems perform an automated analysis, potentially relieving physicians from heavy workloads. However, the large number of wearable ECG recorders developed for consumer use are dreaded by a relevant degree of inconclusive tracings, potentially undermining their usefulness[50].
Artificial intelligence tools have also been applied to data extraction from cardiac implantable electronic devices (CIEDs), demonstrating the ability to predict clinically relevant atrial high-rate episodes (AHRE) and electrical storms[51,52]. Moving to current clinical applications, an AI tool (AccuRhythm™ AI, Medtronic, Dublin, Ireland) has recently received the Food and Drug Administration (FDA) market clearance for use with an insertable cardiac monitor (ICM). The two most common sources of ICM false alerts are AF and Pause detection. Validation data demonstrate that the sensitivity of AF algorithm to true ICM detections was 99% at the alert level. The relative specificity of the AF algorithm was 74% at the alert level. The AI retained 99.9% of the total true AF duration detected by the ICM with a positive predictive value of 98,6%. For pause algorithm, sensitivity and specificity were 99.7% and 98.3%, respectively; as a result, false alerts were reduced by 97.4% while maintaining 100% of true alerts[53,54].
Compared to standard detection algorithms using fixed rules for cardiac electrical signal analyses with reduced capacity to adapt to specific patient patterns, AI seems promising in reducing the burden of data review, performing efficient and accurate data triage with overall reduced false alert detection. In addition, the identification and use of patient-specific rate and rhythm models seem to be an advantage in the prediction of future clinical events. Probably, in the next years, a growing amount of data will be needed to develop and validate new AI models. This could be a limit for further advancements. In fact, collecting data to create new databases is a costly task both economically and temporally. The use of realistic synthetic ECGs may overcome this issue by enabling the generation and augmentation of training data. In addition, many studies found models to synthetize ECG signals by using mathematical modeling[55-59]; moreover, new methods using DL techniques have been recently described[60]. Compared to traditional methods, these models can easily generate a particular type of abnormal heartbeat and may also be easier for operators to use as they do not have to spend a great deal of time searching for suitable parameters for generation.
CARDIOVASCULAR IMAGING
Similar to what is already described for ECG data, the number of diagnostic images performed has constantly increased over the last years[61]. Hence, there is a concomitant growing need for tools capable of improving workflow efficiency and accuracy in the imaging diagnostic pathway. Artificial intelligence has the potential to address this need, shortening time spent in image analysis and concurrently reducing human errors at different levels. Table 2 summarizes the main achievements of AI in cardiovascular imaging.
Main achievements with AI in the field of cardiovascular imaging
Study | Year | Sensor | Population/dataset | Principal findings |
Kusunose et al.[63] | 2020 | Echocardiography | 300 patients | A DNN algorithm detects regional wall motion abnormalities and differentiate among coronary infarction territories |
Madani et al.[64] | 2018 | Echocardiography | 267 samples | A CNN algorithm recognizes similarities among related views and classifies relevant image features |
Zhang et al.[65] | 2018 | Echocardiography | 14,035 samples | A CNN algorithm accurately identifies views, enables the segmentation of individual cardiac chambers and detects HCM, CA and PAH |
Tabassian et al.[66] | 2017 | MRI | 60 patients | A ML-MRI model captures the spatio-temporal LV characteristics and exploits them for MI diagnosis based on the LV deformation traces |
Sengupta et al.[67] | 2016 | Speckle tracking echocardiography | 94 patients from 103 | A ML-STE model distinguish CP from RCM |
Heitzingeret al.[68] | 2023 | Echocardiography and laboratory data | 3,359 moderate sTR samples 1,509 severe sTR samples | A ML model provides risk-stratification in patients with moderate and severe sTR |
Lei et al.[69] | 2019 | Echocardiography Clinical data ECG | 117 from 184 patients | A ML model can regularize ECG QRS duration (QRSd) through echocardiography and enhance the correlation between QRSd and CRT response |
Chen et al.[71] | 2017 | CNN-cardiac MRI | 3,975 samples | A neural network (U-Net) trained with Cardiac MRI images from a single scanner has the potential to produce competitive segmentation results on multi-scanner data across domains |
He et al.[72] | 2020 | Cardiac MRI | 100 patients | A CNN algorithm performed more rapidly CMR LV segmentation and improves diagnostic efficiency |
Bhuva et al.[73] | 2019 | Cardiac MRI | 110 patients | A ML model is faster with similar precision to the most precise human techniques to detect changes in EF |
Augusto et al.[74] | 2001 | Cardiac MRI | 60 patients | A ML algorithm is superior to experts in MWT measurement in patients with HCM |
Fahmy et al.[76] | 2018 | Cardiac MRI | 1,041 samples from 7,775 | DCN automatically quantifies left ventricle mass and scar volume in LGE sequences in patients with HCM |
Fahmy et al.[77] | 2019 | Cardiac MRI | 149 processing layers with a total of approximately 9 million kernels | FCN allows fast and automatic analysis of myocardial native T1 mapping images and myocardial segmentation |
Neisius et al.[78] | 2019 | Cardiac MRI | 332 patients | An AI model discriminates between hypertensive heart disease and HCM patients and provides incremental value over global native T1 mapping through the analysis of native T1 images |
Wang et al.[79] | 2020 | Cardiac MRI | 102 patients | An AI model discriminates between MYH7- and MYBPC3 genes -associated HCM patients through the analysis of native T1 images |
Xue et al.[80] | 2022 | Cardiac MRI | 160 patients | An AI model measured MAPSE and GL‐Sh with strong associations with adverse outcomes |
Coenen et al.[81] | 2018 | CCTA | 525 vessels 351 patients | An AI model improves the performance of CCTA-FFR by correctly reclassifying hemodynamically nonsignificant stenosis and performs equally well as CFD-based CT-FFR in CAD |
Kang et al.[82] | 2015 | CCTA | 126 vessels 42 patients | An AI model allowed detection of obstructive and nonobstructive lesions from CTA |
Liao et al.[83] | 2022 | CT MRI PET | 142 saples 6,066 samples 131 samples | An AI model allows more accurate and high-quality image reconstruction |
Motwani et al.[84] | 2017 | CCTA | 10,030 samples from COronary CT angiography evaluatioN For clinical outcomes: an inteRnational multicenter registry | An ML model predicts 5-year all-cause mortality better than existing clinical or CCTA metrics alone |
van Rosendael et al.[85] | 2018 | CCTA | 8,844 patients | A ML model improves the integration of CCTA derived plaque information to improve risk stratification |
Li et al.[86] | 2022 | PET | 22 XCAT phantoms were generated (10 for training, 1 for validation and 11 for testing) | A CNN algorithm reduces motion artifacts while utilizing all gated PET data |
Nakajima et al.[87] | 2017 | SPECT | 1,001 samples | An ANN model is accurate in various clinical settings, including patients with previous myocardial infarction and coronary revascularization and helps to diagnose CAD |
Arsanjani et al.[88] | 2013 | SPECT | 1,181 samples | A ML algorithm improves diagnostic performance of SPECT in CAD patients |
Betancur et al.[89] | 2018 | SPECT | 1,638 patients | A CNN model improves automatic interpretation of SPECT in CAD patients |
Betancur et al.[90] | 2018 | SPECT | 2,619 patients | A ML model predicts 3-year risk of MACE |
Arsanjani et al.[91] | 2015 | SPECT | 1,085 patients | A ML model is comparable or better than experienced readers in prediction of the early revascularization after SPECT |
Sabatino et al.[98] | 2020 | Echocardiography | 50 patients | Non-invasive MW indices identifies the ischaemic risk area during transient acute coronary occlusion |
Sabatino et al.[99] | 2021 | Echocardiography | 80 patients | Non-invasive estimation of MW indices is able to predict critical coronary artery stenosis before invasive angiography |
Raghavendra et al.[100] | 2022 | Echocardiography | 54 patients | A ML algorithm highlighted cardiac structural alteration in hypertensive patients |
Vidya et al.[101] | 2015 | Echocardiography | 800 echocardiography image frames | An AI model predicted myocardial infarction |
Glessgen et al.[102] | 2023 | CCTA | 281 patients | A DL model is able to diagnose significant coronary artery stenosis in patients with acute chest pain |
Knott et al.[103] | 2020 | Cardiac MRI | 1049 patients | An AI model calculates myocardial blood flow and myocardial perfusion reserve provides a strong, independent predictor of adverse cardiovascular outcomes |
Qiao et al.[111] | 2020 | CCTA | 197 patients | ML-based FFR-CT algorithm had superior prognostic value for severe anatomic stenosis |
Min et al.[115] | 2020 | OCT-TCFA | 602 coronary lesions from 602 patients | A DL algorithm accurately detects an OCT-TCFA with high reproducibility |
Cho et al.[116] | 2021 | IVUS | 598 coronary arteries from 598 patients | IVUS-based deep learning algorithm performed fast and accurate assessment of the extent of calcified and attenuated plaques in whole vessels |
Cook et al.[117] | 2019 | iwFR | 1,008 images | AI-based iFR pressure-wire pull back data is noninferior to expert human interpretation in determining both the hemodynamic appropriateness for PCI and the optimal physiological strategy for PCI |
Miyoshi et al.[118] | 2020 | Coronary angioscopy | 107 images from 47 patients 864 images, selected from 142 MEDLINE-indexed articles | A DCNN algorithm is able in both predictive and generative modelling that can help develop the diagnostic support system for coronary angioscopy |
Shah et al.[137] | 2015 | Echocardiography Clinical data and ECG | 397 patients | An AI model improves the classification of HFpEF |
Hedman et al.[141] | 2020 | Echocardiography Clinical and laboratory data | 32 echocardiograms from 320 HFpEF patients | A ML algorithm identifies distinct HFpEF phenogroups |
Schrub et al.[142] | 2020 | Echocardiography Clinical and laboratory data | 538 patients from the multicentre KaRen study | An AI model recognizes subgroups with different physiopathologies in HFpEF |
Cikes et al.[149] | 2018 | Echocardiography Clinical data | 1,106 HF patients from the MADIT-CRT trial | A ML algorithm identifies patients with beneficial response to cardiac resynchronization therapy |
Diller et al.[157] | 2020 | Cardiac MRI | 303 pediatric patients | A PG-GAN model generates accurate synthetic equivalents to MRI frames from patients with tetralogy of fallot |
Diller et al.[161] | 2020 | Cardiac MRI | 372 patients | A ML model estimates prognosis in adults with repaired tetralogy of fallot |
Diller et al.[163] | 2019 | Echocardiography | 267 patients | A DL algorithm is able of denoising and artefact removal in patients with CHD |
Ishikita et al.[166] | 2023 | Cardiac MRI Clinical data | 804 patients | A ML model predicts 5-year MACE in adults with repaired tetralogy of Fallot |
With its inception in the early fifties, AI is now implemented in routine echocardiography practice[62]. Deep learning algorithms can classify echocardiographic views with the same accuracy as board-certified echocardiographers and are able to detect regional wall motion abnormalities, with better outcomes than the analysis performed by residents[63,64]. Quantification of the major echocardiographic parameters is often time-consuming for the operator. Researchers demonstrated that NN algorithms can provide a fully automated myocardial segmentation with an estimation of volumes, mass, and longitudinal strain[65]. Beyond processing and analysis, AI can be helpful in disease classification; a recent study demonstrated that AI has an accuracy of nearly 90% in identifying myocardial infarction, by interpreting the analysis of strain rate curves and myocardial deformation[66]. Similar excellent diagnostic accuracy was demonstrated for an associative memory classifier able to distinguish between restrictive cardiomyopathy and constrictive pericarditis by analyzing differences in the speckle tracking patterns[67]. These tools can also be applied to further stratify patients to obtain meaningful prognostic information. A machine learning derived approach applied was able to reclassify patients with moderate and severe tricuspid regurgitation on the basis of identified adverse features yielding important prognostic information[68]. The additional information provided can be integrated across different modalities and aid clinical management. For instance, a ML model was able to predict response to cardiac resynchronization by combining echocardiographic, clinical, and electrocardiographic features[69]. AI models are, therefore, increasingly used in echocardiography, from image acquisition to analysis and development of novel risk stratification tools. Similar evidence is available in the literature about the growing role of AI in cardiac magnetic resonance (CMR)[62,70]. The correct planning and acquisition of CMR sequences usually requires highly trained staff, having a significant impact on the quality of the images acquired and their diagnostic value. Vendors are constantly working on the implementation of AI-based automatic acquisition tools to assist in planning and optimizing the quality of the data acquired[70]. In addition, as for other imaging modalities, several studies have demonstrated the feasibility and good accuracy of AI-based segmentation models in CMR[71,72]. This significantly reduces the time spent in post-processing, providing a precise and automated estimation of ejection fraction, mass, and volumes in a few seconds[73]. More importantly, ML models proved to be more consistent and reproducible compared to human experts in measuring key parameters such as maximum wall thickness, with potential diagnostic and management implications[74]. AI models also perform well in tissue characterization[75]; a deep fully CNN can quantify with good accuracy native T1 mapping values in patients with hypertrophic cardiomyopathy (HCM) and the presence of late gadolinium enhancement in patients with myocardial infarction[76,77]. A novel technique called texture analysis based on the capability of ML algorithms to analyze spatial heterogeneity of adjacent pixels has been demonstrated to be helpful in challenging clinical contexts such as differentiation between patients with HCM and hypertensive heart disease, but also between patients with different genetic mutations[78,79]. Despite these models still requiring supervision, they could potentially represent useful screening tools to be used on a large scale. The strong clinical interest in AI analysis is also driven by the potential prognostic implication of imaging markers that can be automatically measured. In a cohort of 1,572 patients, automated in-line assessment of global longitudinal shortening and mitral annular plane systolic excursion (MAPSE) had a strong association with adverse outcomes[80].
Excellent diagnostic accuracy has also been demonstrated in cardiac computed tomography (CCT), where AI can aid in the segmentation and detection of coronary artery plaques[81,82]. Additionally, in this field, the application of AI-derived algorithms is helpful in reducing the overall radiation doses used to acquire diagnostic images and therefore patient exposure[83]. Several studies have also demonstrated that ML-derived CCT algorithms have a superior prognostic value compared to conventional clinical scores[84,85]. Obtaining good image quality in the shortest time possible is key to all imaging modalities. Patient breathing and movement during positron emission tomography (PET) data acquisition affect image quality. A deep neural network has been successfully applied to reduce motion artifacts, outperforming the iterative registration-based method[86]. In nuclear imaging, several AI-based algorithms have been demonstrated to be able to identify stress-rest defects and increase the overall accuracy of the modality by implementing imaging data with clinical features[87-91]. Despite evidence of undeniable usefulness, there are still some concerns about the wider use of AI in CV imaging due to the lack of standardized methods and the ethical issues related to potential threats to patient privacy and confidentiality. However, the unique advantage of AI in providing fast and reliable segmentation and analysis of the major imaging parameters is meant to increase its implementation among different imaging modalities soon.
CORONARY ARTERY DISEASE
Coronary artery disease (CAD) is associated with a high risk of morbidity and mortality[92]. In this context, AI could be used throughout the diagnostic definition of CAD, from the assessment of pre-test risk to the application in imaging techniques used in clinical practice for CAD evaluation[93].
In the emergency department, DNNs have proven their effectiveness in recognizing patterns related to myocardial infarction[94-96]. These methods aim to promote medical assistance even in areas with limited healthcare resources by lowering operator-dependent variability. A recent ML algorithm through three clinical variables (age, sex, and prior percutaneous coronary intervention) in addition to levels of hs-cTnI, KIM-1, and adiponectin showed exceptional accuracy in predicting CAD, especially in patients with an undetermined value of hs-cTNI[97]. The role of AI in CV imaging has already been discussed. However, there is an increasing interest in novel and early imaging biomarkers able to predict the presence of CAD and myocardial ischemia[98,99]. In the context of stress echocardiography, ML and DL methods were used to identify regional wall motion abnormalities, showing a great level of reliability[100,101]. In this context, researchers developed a DNN system to calculate an appropriate score to estimate the likelihood of CAD[89]. In addition, a large retrospective study demonstrated that DML has a high negative predictive value in evaluating coronary CT images for significant CAD in patients presenting with acute chest pain[102]. All patients with ACS and stenoses by invasive coronary angiography were identified by the DLM. A step forward in terms of complexity and clinical usefulness came more recently from a study by Knott and collaborators: they showed how AI can elaborate complex imaging data in a fully automatic way, providing, at the same time, a synthetic output with clinical and prognostic relevance[103]. More recent studies showed how AI can also improve the efficiency of non-invasive functional assessment of CAD. Fractional flow reserve (FFR) and a family of pressure-based resting indices allow the functional assessment of the coronary circulation[104-106], being more accurate than the sole coronary angiography but is invasive and costly. Non-invasive methods for CAD detection represent a valid alternative nowadays, with coronary computed tomography angiography (CCTA) as the primary approach[107]. However, while CCTA has high sensitivity, it can produce false positives and does not assess physiological function. Non-invasive computed tomography-derived FFR (CT-FFR) was therefore introduced as a way to assess ischemia without additional procedures[108]. In particular, CT-FFR has been proposed as an alternative diagnostic tool in patients with stable angina, with equivalent clinical outcomes, QoL, and lower costs compared to usual care, with a reduced rate of invasive unnecessary coronary angiography[109]. In this context, artificial intelligence algorithms and deep neural networks have been developed, improving diagnostic accuracy and outcome prediction through deep learning-CT-FFR[110]. CT-FFR has shown promise with good accuracy, and new software and ML algorithms have improved its diagnostic capabilities. Very recently, a supervised learning approach was adopted to improve the performance of a multiphysics model based on computational flow dynamics, simulating blood flow in vessels[81]. This approach was tested in a perspective study with an improved software using DL named Coronary Scope, with excellent results[111]. According to the FORECAST trial, the use of CT-FFR with off-site computational fluid dynamics could reduce invasive procedures without significant differences in MACE, QoL, and angina symptoms[112]. On the other hand, the use of computational flow dynamics could have some limitations, such as the complexity of calculations. Therefore, using a deep learning algorithm in this context could reduce transfer times and prevent the need to send sensitive clinical data. In the recent TARGET randomized trial, on-site machine learning-based CT-FFR, in comparison to standard care, decreased the rate of invasive coronary angiographies in patients with stable coronary artery disease in the absence of coronary obstruction or need for intervention in the following 90 days. However, although there was an increase in revascularizations, no improvement in symptoms or QoL was documented[113]. Furthermore, fully automated (without human input) deep learning models have been proposed to compute FFR from CCTA, achieving 76% accuracy in detecting FFR variations[114]. AI-based algorithms also showed an excellent performance for prognostic prediction[90].
In relation to CV imaging, furthermore, researchers[27] have developed a ML algorithm that allowed thin-cap fibroatheroma (TCFA) to be recognized with high efficacy using IVUS, while Min et al. deployed a DL model to automatically identify TCFA from OCT images[115]. Cho et al. proposed a DL system to discriminate IVUS segments as plaque with attenuation or calcification, in order to help with the detection of high-risk lesions[116]. Other promising applications of AI algorithms to the broader family of invasive coronary diagnostics are the recently explored fully automatic processing and interpretation of pull-back measurement of the resting pressure-base index, known as the instantaneous wave-free Ratio (iFR). An international collaboration, coordinated by the group led by Justin E. Davies from the Hammersmith Hospital, demonstrated that the AI-based interpretation of iFR output was non-inferior to human interpretation by expert interventionalists, to discriminate both the clinical indication and the optimal physiology-based strategy for percutaneous coronary revascularization[117]. AI applications to CAD are designing a growing panorama of different tools to the benefit of cardiologists, involving both simple one-scope tools and support system capable of elaborating complex decision support resources. At the forefront of current research is the development of automated algorithms for the interpretation of coronary angiographies[118], promising to provide additional information to the traditionally extracted evidence of coronary stenoses and/or additional qualitative information for more focused and reliable measurements of multiple parameters, such as the hemodynamic impact of coronary lumen geometry, calcification or tortuosity, to the complex integration of multiple layers of information to guide the entire clinical process[119,120].
HEART FAILURE
With a prevalence of 1%-2% in the adult population, HF represents a significant global issue and one of the most common causes of hospitalization[92,121-125]. Although the prognosis of patients with HF has considerably improved over decades, it remains poor[126], with overall reduced QOL.
Multiple potential applications of AI were recently proposed to help solve the many challenges in the clinical management of HF[127]. Among the most promising areas is the improvement and simplification of both the identification of new risk factors for incident HF and its clinical monitoring. A ML approach was used to analyze data from more than 500000 individuals, recognizing the strongest features associated with incident HF, which included leg bioimpedance in addition to known risk factors for HF[128].
Beyond the aforementioned ECG-based algorithms able to identify patients with HF (40) and asymptomatic LV dysfunction[16], researchers reported several alternative AI strategies based on clinical features[129-131].
An AI model[132] used heart sound characteristics for the detection of HF. In a more recent study, a new AI model that combines supervised and unsupervised methods in different levels of learning for enhanced performance, called the time-growing neural network (TGNN), has been used for the analysis of the phonocardiogram and allows the detection of any obstruction on semilunar heart valve in children, even for the mild forms[133]. These findings open the scenario for the use of a smart stethoscope for decentralized diagnosis of heart disease. In addition, a ML-based approach was used to develop a novel risk prediction tool, WATCH-DM, which predicts the risk of incident HF and hospitalization among diabetic patients[134]. A race-specific ML-based model was also able to predict the 10-year risk of incident HF with superior performance compared to traditional HF risk calculators and non-race-specific ML models[135]. Another study validated a ML model able to provide real-time decision support to adults diagnosed with congestive HF, achieving higher exacerbation and triage classification performance than physicians[136].
Heart failure is a heterogeneous syndrome characterized by one or more contributing risk factors that ultimately lead to abnormal cardiac structure and function. The contemporary classification based on ejection fraction values may have limitations in the future when tailored medicine is expected. In this scenario, DL algorithms could help improve the classification of HF. The first detailed clinical, laboratory, ECG, and echocardiographic phenotyping of a HF with preserved ejection fraction (HFpEF) population was performed using a statistical learning algorithm[137]. The phenomapping analysis classified study participants into three distinct groups that differed markedly in clinical characteristics, cardiac structure/function, invasive hemodynamics, and outcomes. After this landmark work, an increasing number of studies have been published on the application of phenomapping to different cohorts of patients with HFpEF, with some focused only on clinical and laboratory parameters[138,139], and others including echocardiographic parameters[140-142].
Machine learning enables clustering of common clinical and/or laboratory characteristics, leading to the identification of less obvious or “predictable” HFpEF subgroups.
In a more recent study[143], researchers used a ML approach to separate patients according to different comorbidity profiles or phenotypic groups, regardless of LVEF. They described six phenotypes, characterized by different comorbidity profiles. This classification, independent of LVEF, resulted in greater separation of clusters and better association with clinical outcomes at 6 and 12 months than conventional classification of HF, suggesting that classifying HF patients based on clinical characteristics rather than LVEF categories provides relevant prognostic information.
Artificial intelligence can also be applied for prognostication purposes, as demonstrated by several studies where ML algorithms were able to predict both the risk of mortality in the acute setting, during hospitalization, and long-term outcomes[144-147].
Meeting the need for an increasingly tailored medicine, ML algorithms could allow the customization of drug therapy according to the characteristics of the patient. Despite an overall lack of efficacy from β blockers in individuals with HFrEF and AF, thanks to an ML approach, Karwath et al. were able to identify a subgroup of AF patients where β blockers did reduce death[148]. Finally, ML may also be used to optimize patient selection for device therapy in HF. Indeed, a ML-based approach was used to combine both standard clinical parameters and advanced echocardiographic data to identify patients most likely to respond to cardiac resynchronization therapy (CRT)[149].
CONGENITAL HEART DISEASE
The burgeoning demand for cardiac imaging in Adult Congenital Heart Disease (ACHD), due to the advances in therapeutic management and improved survival, is unfortunately not paralleled by a corresponding surge in the number of healthcare practitioners specialized in interpreting congenital cardiac images. The complexity and high variability of the anatomy, including previous surgical intervention, may, in fact, represent a challenge for non-specialized cardiologists. The advent of AI may help in overcoming some of these challenges, having a significant impact on patient care. Several studies have already tested the applicability and advantages of these tools in this field, using deep learning for image acquisition, post-processing, and diagnosis. AI-based algorithms have been used for LV detection in CMR images, with the latest technique using deep reinforcement learning[150-152].
The latter approach interacts with the environment and includes a sophisticated search method consisting of structured, tiered action phases. AI has also been applied to reduce CMR scan time, which is helpful not only in increasing patient compliance but also in reducing artifacts and improving overall image quality[153]. Deep learning algorithms have been demonstrated to be able to accelerate CMR scan time by reconstructing images from under-sampled data[154]. Despite more data certainly needed to standardize its use in clinical practice, preliminary data also show the potential usefulness of automated segmentation analysis tools in complex CHD patients[155-158]
By eliminating the laborious and time-consuming aspects of quantifying anatomical structures within these images, the risk of healthcare provider burnout is mitigated[159]. Consequently, clinicians can redirect their focus towards more elevated functions, nurturing the doctor-patient relationship, and delivering healthcare services infused with heightened empathy, which is crucial in the special ACHD population. Deep learning holds the promise of exceeding the capabilities of expert human image interpretation. Nonetheless, a substantial delivery gap persists, primarily attributable to data availability. Data preparation is also laborious: for ML, 80% of the time is spent on data preparation and only 20% is spent on algorithm development. This is particularly true for medical images portraying congenital heart pathologies, due to inconsistencies in DICOM (the standard for medical images) meta-data between different institutions and scanner manufacturers. Among the others, Diller et al. have delineated a pioneering DL methodology to estimate prognosis and guide therapy in ACHD using data from a single tertiary center including over 10,000 patients and 44,000 medical reports for congenital heart disease[160]. To this purpose, clinical and demographic data, ECG parameters, cardiopulmonary exercise testing, and laboratory values were accumulated and included in appropriate recurrent deep learning algorithms. In addition, models were developed to estimate the need for discussions within multidisciplinary teams, with an accuracy of 90%.
Another study[161] used the same methodology for the analysis of cardiac magnetic resonance imaging in individuals who have undergone surgical repair for tetralogy of Fallot. The researchers initially trained their model using images sourced from a singular medical center. Therefore, this model was applied to an independent cohort of patients with documented clinical outcomes, who had been reserved for validation purposes. From the derived volumetric data analysis, the authors constructed a prognostic model. This model, through its identification of heightened right atrial area and diminished right ventricular longitudinal function, was capable of discerning patients at an elevated risk of experiencing adverse outcomes. It is imperative to underscore that this research represents a pioneering milestone; however, it is critical to acknowledge, as the authors themselves do, that this paper serves as a proof of principle[162]. Overall, deep learning enhances the efficiency of image interpretation, aiding clinicians in the timely and accurate diagnosis of congenital heart defects, potential complications, and disease progression[163,164].
For instance, the Montreal group[165] proposed a novel recurrent neural networks (RNN) to model longitudinal medical data named Deep Heart-failure Trajectory Model, to predict the recurrence of future heart failure events in ACHD patients. Using a large dataset from the EHR documented Quebec Congenital Heart Disease database (84,498 patients, 28 years of follow-up), composed by demographics and several clinical variables, their model enhances the accuracy of recurrent events prediction compared to other AI-based models. The prognostic value of AI models may be particularly helpful in assessing preoperative risk of death. A machine learning prediction model applied in a single-center study, using clinical and CMR variables, was demonstrated to perform well in predicting MACE at 5 years in adults with repaired tetralogy of Fallot[166].
Leveraging advanced algorithms and analyzing comprehensive patient data such as clinical records, cardiovascular MRI, and demographic information, these models provide accurate and personalized assessments of mortality risk. This transformative approach empowers clinicians to make informed decisions, tailor surgical strategies, and ultimately enhance patient outcomes in ACHD surgery[167].
Despite the great potential of deep learning and imaging technologies in ACHD, its integration into clinical practice remains limited. Challenges such as the scarcity of large, standardized datasets and the complex nature of ACHD pathologies have hindered the widespread adoption of deep learning models.
PITFALLS, CURRENT BARRIERS AND POTENTIAL SOLUTIONS
AI is already a reality for cardiology and its increasing adoption in the future is inevitable. However, some critical issues still need to be addressed. One of the most critical aspects is the generalizability of AI algorithms, which is strictly related to the dataset used to train and test them.
A poor dataset, in fact, can easily lead to overfitting and limit the performance of the AI application. In other words, if some conditions are under-represented in the dataset, or if the dataset itself is affected by some bias, the predicted results will be influenced as well (the “garbage in - garbage out” rule represents very well the pitfall in this field)[168]. To overcome this limitation, two strategies, relying on different concepts of clinical data management, have been proposed. From a historical point of view, the first idea was to share the data among hospitals to create a large, public, heterogeneous, multi-institution, and multi-device dataset. This would allow more robust and representative training and a fairer testing/comparison of the proposed algorithms. However, this approach requires sharing clinical data that contain highly sensitive information about patients. As a result, it is mandatory to share data in a secure and privacy-respectful manner, while also adhering to the rules of each country. However, even assuming a successfully law-compliant workflow, this strategy represents a significant burden both for the administrative staff (required to authorize the sharing and define the modalities for this) and for the technical personnel (who have to carefully implement and execute the anonymization and the sharing routines). In addition, even if potentially able to deal with this aspect, not all Institutions are willing to share their own data.
In the past few years, a new approach, known as federated learning, has been introduced. The rationale behind this innovative strategy is to “share the AI model” rather than the dataset[169]. This is accomplished by involving each clinical partner in training its own AI model locally, exclusively by using its own data. Once the institution-specific AI models are trained, they are merged to generate a single global AI that is sent back to each center, guaranteeing a more secure and simple pipeline, since only the mathematical models are shared and not the patients’ data, see Figure 2 for schematic representation. In this way, each clinic will have information extracted by considering data that do not belong to that institution.
Figure 2. Schematic representation of federated learning. Federated learning is a decentralized technique for machine learning that produces an algorithm trained by utilizing collected data on decentralized devices or servers, without any exchange of the data itself. Local systems employ independent machine learning by utilizing an encrypted dataset to generate a local model. A central aggregator integrates all the local models and generates a global one. This collaborative system allows different actors to collaborate on a global machine-learning model without exchanging or uploading any data to a remote server.
Another issue of AI approaches, still strictly connected to the dataset, is the procedure to fairly test the developed algorithms. Ideally, this should be done by benchmarking the model on a large set of unseen data collected in a different institution and/or device. Unfortunately, due to the lack of open-source data, several authors have to deal with small and private datasets, which forces them to adopt a k-fold validation in their studies over external and heterogeneous data. However, even if this strategy is not conceptually wrong, it remains a suboptimal validation procedure. Like any newly introduced technology in healthcare, robust clinical validation is required to avoid potential mistakes that could have catastrophic consequences. A recent literature review[170] highlighted that most AI assessment studies have been assessed exclusively on internal datasets, with no external validation and a lack of recommended design features for accurate validation. Randomized clinical trials (RCTs) where AI models are compared to the gold standard are the optimal and most reliable way to assess AI performance and safety. On the other hand, RCTs have high financial costs and require previous studies and a long period of time. The European ITFoC consortium proposed an alternative method with the potential to accelerate the transfer of AI into clinical settings. They described a validation framework for the assessment of AI models based on real-world data, which includes seven principles: the intended use of AI, the target population, the timing for AI evaluation, the datasets selected for evaluation, the procedures used to ensure data safety, the metrics used to measure performance, and the procedures used to ensure that the AI is explainable[171]. The potential advantages of the use of a validation platform with external real-world datasets include cost and time reduction; transparency, reusability of the platform for further AI evaluations; and integration with new datasets.
Another key aspect to consider before a secure and large-scale usage of AI in medical applications concerns the interpretability/explainability of how the model produces its output. In fact, the risk is to have a box that generates very accurate predictions or classifications without the possibility of understanding the logic used to reach that conclusion. Of course, any clinician will treat a patient on the basis of a “digital greek-oracle” statement.
In this regard, AI algorithms can be divided into two classes, commonly named “white box” and “black box”[172].
The first group includes the mathematical models (typically the ML strategies) that can be interpreted by humans, while the second group includes models that are difficult or impossible to explain (such as the DL pipelines). It is important not to confuse the term “explainable” with the concept of “trivial”. In fact, the idea of explainability is mainly related to the ability of humans to assign a biological interpretation to the complex mathematical operations behind each strategy. Deep learning strategies are less explainable than classical ML algorithms just because they use many more parameters (up to some millions) to reach their decision, making the logical interpretation complex. However, significant technical efforts are already mitigating this issue, providing users with some insight into the decision taken. Gradcam algorithms, for example, inform the user about the features identified in the signal that drives the final decision[173].
The rapid widespread use of AI-based tools for clinical applications has induced regulatory agencies to establish an approval system requiring proof of safety and efficacy for computer-assisted software intended for clinical use before these can reach the market. Multiple steps are envisioned for an AI-based tool to comply with regulatory authorities and finally get approved as a computer-assisted device.
The legal and ethical implications are another issue of AI application, with the main points of concern represented by privacy and data protection, transparency, bias, discrimination, and responsibility. To reach their full potential, AI models need to have access to a wide range of patient information, both medical and non-medical. Potentially, these data could be hacked and used for malicious purposes. A key aspect to consider is, therefore, the creation of systems and regulations that guarantee patient safety and confidentiality. The majority of legal discussions on AI revolve around the issue of algorithmic transparency. Indeed, as explained above, obtaining information about the functionality of algorithms is often difficult, if not impossible. It is also known that AI models can incorporate human and social biases at scale[174]. For instance, data that include human decisions or data that display the secondary impacts of social or historical inequalities related to race and sex[175] can be used to train models. There are several cases of initially promising AI models with worse performance when applied to diverse populations[176,177]. For these reasons, a report of performance among diverse ethnic, racial, age, and sex groups should be ideally obtained for all new AI tools to ensure responsible use of this technology in healthcare. We should also consider that machines that can work according to unfixed rules and learn new models of behavior are said to threaten our ability to trace culpability back to the maker or operator. Nowadays, there is a lack of comprehensive regulations to address the potential legal and ethical consequences of using AI in healthcare settings. For these reasons, the European Commission is currently working on a general regulatory framework for AI. This will be based on the classification of potential risks associated with the use of AI and will define the fields of application. Once the use of AI is regulated, the above issues are solved, and a model with better performance than previous technology has been identified, we imagine that there will be a reasonable routine application of it in the healthcare setting. In conclusion, although a lot more work will be needed on the scientific, regulatory, and organizational levels, the future appears to be bright for AI in CV medicine.
DECLARATIONS
Authors’ contributions
Conceptualization: Strangio A, De Rosa S
Draft of the manuscript: Strangio A, Leo I, Sabatino J, Siracusa C, Carabetta N, Zaffino P, Laschera A, De Rosa S, Brida M, Spadea MF, Torella D, Critelli C, Sabouret P
All authors reviewed and approved the final version of the manuscript.
Availability of data and materials
Not applicable.
Financial support and sponsorship
This work was supported by grants from the Italian Ministry of University and Research (PNRR-National Center for Gene Therapy and Drugs based on RNA Technology - CN00000041), and from the Italian Ministry of Health (PSC SALUTE 2014-2020 -POS4 “Cal-Hub-Ria”-T4-AN-09 and PNRR-MAD-2022-12376814).
Conflicts of interest
All authors declared that there are no conflicts of interest.
Ethical approval and consent to participate
Not applicable.
Consent for publication
Not applicable.
Copyright
© The Author(s) 2024.
REFERENCES
1. Bergami M, Scarpone M, Bugiardini R, Cenko E, Manfrini O. Sex beyond cardiovascular risk factors and clinical biomarkers of cardiovascular disease. Rev Cardiovasc Med 2022;23:19.
2. Zhou SS, Jin JP, Wang JQ, et al. miRNAS in cardiovascular diseases: potential biomarkers, therapeutic targets and challenges. Acta Pharmacol Sin 2018;39:1073-84.
3. Westerlund AM, Hawe JS, Heinig M, Schunkert H. Risk prediction of cardiovascular events by exploration of molecular data with explainable artificial intelligence. Int J Mol Sci 2021;22:10291.
4. Bivard A, Churilov L, Parsons M. Artificial intelligence for decision support in acute stroke - current roles and potential. Nat Rev Neurol 2020;16:575-85.
5. Reel PS, Reel S, van Kralingen JC, et al. Machine learning for classification of hypertension subtypes using multi-omics: a multi-centre, retrospective, data-driven study. EBioMedicine 2022;84:104276.
6. Poss AM, Maschek JA, Cox JE, et al. Machine learning reveals serum sphingolipids as cholesterol-independent biomarkers of coronary artery disease. J Clin Invest 2020;130:1363-76.
7. de Gonzalo-Calvo D, Martínez-Camblor P, Bär C, et al. Improved cardiovascular risk prediction in patients with end-stage renal disease on hemodialysis using machine learning modeling and circulating microribonucleic acids. Theranostics 2020;10:8665-76.
8. Schlant RC, Adolph RJ, DiMarco JP, et al. Guidelines for electrocardiography. A report of the American college of cardiology/American heart association task force on assessment of diagnostic and therapeutic cardiovascular procedures (committee on electrocardiography). Circulation 1992;85:1221-8.
9. Hannun AY, Rajpurkar P, Haghpanahi M, et al. Cardiologist-level arrhythmia detection and classification in ambulatory electrocardiograms using a deep neural network. Nat Med 2019;25:65-9.
10. Shah AP, Rubin SA. Errors in the computerized electrocardiogram interpretation of cardiac rhythm. J Electrocardiol 2007;40:385-90.
11. Guglin ME, Thatai D. Common errors in computer electrocardiogram interpretation. Int J Cardiol 2006;106:232-7.
12. Poon K, Okin PM, Kligfield P. Diagnostic performance of a computer-based ECG rhythm algorithm. J Electrocardiol 2005;38:235-8.
14. Smith SW, Rapin J, Li J, et al. A deep neural network for 12-lead electrocardiogram interpretation outperforms a conventional algorithm, and its physician overread, in the diagnosis of atrial fibrillation. Int J Cardiol Heart Vasc 2019;25:100423.
15. Liu CW, Wu FH, Hu YL, et al. Left ventricular hypertrophy detection using electrocardiographic signal. Sci Rep 2023;13:2556.
16. Attia ZI, Kapa S, Lopez-Jimenez F, et al. Screening for cardiac contractile dysfunction using an artificial intelligence-enabled electrocardiogram. Nat Med 2019;25:70-4.
17. Attia ZI, Noseworthy PA, Lopez-Jimenez F, et al. An artificial intelligence-enabled ECG algorithm for the identification of patients with atrial fibrillation during sinus rhythm: a retrospective analysis of outcome prediction. Lancet 2019;394:861-7.
18. Raghunath S, Ulloa Cerna AE, Jing L, et al. Prediction of mortality from 12-lead electrocardiogram voltage data using a deep neural network. Nat Med 2020;26:886-91.
19. Poungponsri S, Yu XH. An adaptive filtering approach for electrocardiogram (ECG) signal noise reduction using neural networks. Neurocomputing 2013;117:206-13.
20. Jadhav SM, Nalbalwar SL, Ghatol A. Artificial neural network based cardiac arrhythmia classification using ECG signal data. In: 2010 international conference on electronics and information engineering; 1-3 Aug 2010; Kyoto, Japan.
21. Acharya UR, Oh SL, Hagiwara Y, et al. A deep convolutional neural network model to classify heartbeats. Comput Biol Med 2017;89:389-96.
22. Haseena HH, Mathew AT, Paul JK. Fuzzy clustered probabilistic and multi layered feed forward neural networks for electrocardiogram arrhythmia classification. J Med Syst 2011;35:179-88.
23. Sayantan G, Kien PT, Kadambari KV. Classification of ECG beats using deep belief network and active learning. Med Biol Eng Comput 2018;56:1887-98.
24. Tateno K, Glass L. Automatic detection of atrial fibrillation using the coefficient of variation and density histograms of RR and deltaRR intervals. Med Biol Eng Comput 2001;39:664-71.
25. Dash S, Chon KH, Lu S, Raeder EA. Automatic real time detection of atrial fibrillation. Ann Biomed Eng 2009;37:1701-9.
26. Huang C, Ye S, Chen H, Li D, He F, Tu Y. A novel method for detection of the transition between atrial fibrillation and sinus rhythm. IEEE Trans Biomed Eng 2011;58:1113-9.
27. Babaeizadeh S, Gregg RE, Helfenbein ED, Lindauer JM, Zhou SH. Improvements in atrial fibrillation detection for real-time monitoring. J Electrocardiol 2009;42:522-6.
28. Jiang K, Huang C, Ye SM, Chen H. High accuracy in automatic detection of atrial fibrillation for Holter monitoring. J Zhejiang Univ Sci B 2012;13:751-6.
29. Xia Y, Wulan N, Wang K, Zhang H. Detecting atrial fibrillation by deep convolutional neural networks. Comput Biol Med 2018;93:84-92.
30. Acharya UR, Fujita H, Oh SL, et al. Automated identification of shockable and non-shockable life-threatening ventricular arrhythmias using convolutional neural network. Future Gener Comput Syst 2018;79:952-9.
31. Sabut S, Pandey O, Mishra BSP, Mohanty M. Detection of ventricular arrhythmia using hybrid time-frequency-based features and deep neural network. Phys Eng Sci Med 2021;44:135-45.
32. Chang KC, Hsieh PH, Wu MY, et al. Usefulness of multi-labelling artificial intelligence in detecting rhythm disorders and acute ST-elevation myocardial infarction on 12-lead electrocardiogram. Eur Heart J Digit Health 2021;2:299-310.
33. Zheng J, Fu G, Struppa D, et al. A high precision machine learning-enabled system for predicting idiopathic ventricular arrhythmia origins. Front Cardiovasc Med 2022;9:809027.
34. Raghunath S, Pfeifer JM, Ulloa-Cerna AE, et al. Deep neural networks can predict new-onset atrial fibrillation from the 12-lead ECG and help identify those at risk of atrial fibrillation-related stroke. Circulation 2021;143:1287-98.
35. Hsu CY, Liu PY, Liu SH, Kwon Y, Lavie CJ, Lin GM. Machine learning for electrocardiographic features to identify left atrial enlargement in young adults: CHIEF heart study. Front Cardiovasc Med 2022;9:840585.
36. Zhao X, Huang G, Wu L, et al. Deep learning assessment of left ventricular hypertrophy based on electrocardiogram. Front Cardiovasc Med 2022;9:952089.
37. Grogan M, Lopez-Jimenez F, Cohen-Shelly M, et al. Artificial intelligence-enhanced electrocardiogram for the early detection of cardiac amyloidosis. Mayo Clin Proc 2021;96:2768-78.
38. Kwon JM, Kim KH, Jeon KH, et al. Development and validation of deep-learning algorithm for electrocardiography-based heart failure identification. Korean Circ J 2019;49:629-39.
39. McDonagh TA, McDonald K, Maisel AS. Screening for asymptomatic left ventricular dysfunction using B-type natriuretic peptide. Congest Heart Fail 2008;14:5-8.
40. Dey M, Omar N, Ullah MA. Temporal feature-based classification into myocardial infarction and other CVDs merging CNN and Bi-LSTM from ECG signal. IEEE Sensors J 2021;21:21688-95.
41. Jahmunah V, Ng EYK, San TR, Acharya UR. Automated detection of coronary artery disease, myocardial infarction and congestive heart failure using GaborCNN model with ECG signals. Comput Biol Med 2021;134:104457.
42. Yadav SS, More SB, Jadhav SM, Sutar SR. Convolutional neural networks based diagnosis of myocardial infarction in electrocardiograms. In: 2021 international conference on computing, communication, and intelligent systems (ICCCIS); 19-20 Feb 2021; Greater Noida, India. pp. 581-6.
43. Hasbullah S, Mohd Zahid MS, Mandala S. Detection of myocardial infarction using hybrid models of convolutional neural network and recurrent neural network. BioMedInformatics 2023;3:478-92.
44. Xiong P, Xue Y, Zhang J, et al. Localization of myocardial infarction with multi-lead ECG based on DenseNet. Comput Meth Prog Bio 2021;203:106024.
45. Ibrahim L, Mesinovic M, Yang KW, Eid MA. Explainable prediction of acute myocardial infarction using machine learning and shapley values. IEEE Access 2020;8:210410-7.
46. Gustafsson S, Gedon D, Lampa E, et al. Development and validation of deep learning ECG-based prediction of myocardial infarction in emergency department patients. Sci Rep 2022;12:19615.
47. Fiorina L, Maupain C, Gardella C, et al. Evaluation of an ambulatory ECG analysis platform using deep neural networks in routine clinical practice. J Am Heart Assoc 2022;11:e026196.
48. Guo SL, Han LN, Liu HW, Si QJ, Kong DF, Guo FS. The future of remote ECG monitoring systems. J Geriatr Cardiol 2016;13:528-30.
49. Abdou A, Krishnan S. Horizons in single-lead ECG analysis from devices to data. Front Signal Process 2022;2:866047.
50. Mannhart D, Lischer M, Knecht S, et al. Clinical validation of 5 direct-to-consumer wearable smart devices to detect atrial fibrillation: BASEL wearable study. JACC Clin Electrocardiol 2023;9:232-42.
51. Kim M, Kang Y, You SC, et al. Artificial intelligence predicts clinically relevant atrial high-rate episodes in patients with cardiac implantable electronic devices. Sci Rep 2022;12:37.
52. Shakibfar S, Krause O, Lund-Andersen C, et al. Predicting electrical storms by remote monitoring of implantable cardioverter-defibrillator patients using machine learning. Europace 2019;21:268-74.
53. Cheng Y, Ousdigian KT, Sarkar S, Koehler J, Cho YK, Kloosterman EM. B-PO04-036 innovative artificial intelligence application reduces false pause alerts while maintaining perfect true pause alert sensitivity for insertable cardiac monitors. Heart Rhythm 2021;18:S293-4.
54. Radtke AP, Ousdigian KT, Haddad TD, Koehler JL, Colombowala IK. B-AB24-04 artificial intelligence enables dramatic reduction of false atrial fibrillation alerts from insertable cardiac monitors. Heart Rhythm 2021;18:S47.
55. McSharry PE, Clifford GD, Tarassenko L, Smith LA. A dynamical model for generating synthetic electrocardiogram signals. IEEE Trans Biomed Eng 2003;50:289-94.
56. Li Z, Ma M. ECG modeling with DFG. In: 2005 IEEE engineering in medicine and biology 27th annual conference 2005; 17-18 Jan 2006; Shanghai, China.
57. Sameni R, Clifford GD, Jutten C, Shamsollahi MB. Multichannel ECG and noise modeling: application to maternal and fetal ECG signals. EURASIP J Adv Signal Process 2007;2007:043407.
58. Clifford GD, Nemati S, Sameni R. An artificial vector model for generating abnormal electrocardiographic rhythms. Physiol Meas 2010;31:595-609.
59. Roonizi EK, Sameni R. Morphological modeling of cardiac signals based on signal decomposition. Comput Biol Med 2013;43:1453-61.
60. Wulan N, Wang W, Sun P, Wang K, Xia Y, Zhang H. Generating electrocardiogram signals by deep learning. Neurocomputing 2020;404:122-36.
61. Smith-Bindman R, Miglioretti DL, Larson EB. Rising use of diagnostic medical imaging in a large integrated health system. Health Aff 2008;27:1491-502.
62. Lim LJ, Tison GH, Delling FN. Artificial intelligence in cardiovascular imaging. Methodist Debakey Cardiovasc J 2020;16:138-45.
63. Kusunose K, Abe T, Haga A, et al. A deep learning approach for assessment of regional wall motion abnormality from echocardiographic images. JACC Cardiovasc Imag 2020;13:374-81.
64. Madani A, Arnaout R, Mofrad M, Arnaout R. Fast and accurate view classification of echocardiograms using deep learning. NPJ Digit Med 2018;1:6.
65. Zhang J, Gajjala S, Agrawal P, et al. Fully automated echocardiogram interpretation in clinical practice. Circulation 2018;138:1623-35.
66. Tabassian M, Alessandrini M, Herbots L, et al. Machine learning of the spatio-temporal characteristics of echocardiographic deformation curves for infarct classification. Int J Cardiovasc Imag 2017;33:1159-67.
67. Sengupta PP, Huang YM, Bansal M, et al. Cognitive machine-learning algorithm for cardiac imaging: a pilot study for differentiating constrictive pericarditis from restrictive cardiomyopathy. Circ Cardiovasc Imag 2016;9:e004330.
68. Heitzinger G, Spinka G, Koschatko S, et al. A streamlined, machine learning-derived approach to risk-stratification in heart failure patients with secondary tricuspid regurgitation. Eur Heart J Cardiovasc Imag 2023;24:588-97.
69. Lei J, Wang YG, Bhatta L, et al. Ventricular geometry-regularized QRSd predicts cardiac resynchronization therapy response: machine learning from crosstalk between electrocardiography and echocardiography. Int J Cardiovasc Imag 2019;35:1221-9.
70. Fotaki A, Puyol-Antón E, Chiribiri A, Botnar R, Pushparajah K, Prieto C. Artificial intelligence in cardiac MRI: is clinical adoption forthcoming? Front Cardiovasc Med 2021;8:818765.
71. Chen C, Bai W, Davies RH, et al. Improving the generalizability of convolutional neural network-based segmentation on CMR images. Front Cardiovasc Med 2020;7:105.
72. He Y, Qin W, Wu Y, et al. Automatic left ventricle segmentation from cardiac magnetic resonance images using a capsule network. J Xray Sci Technol 2020;28:541-53.
73. Bhuva AN, Bai W, Lau C, et al. A multicenter, scan-rescan, human and machine learning CMR study to test generalizability and precision in imaging biomarker analysis. Circ Cardiovasc Imag 2019;12:e009214.
74. Augusto JB, Davies RH, Bhuva AN, et al. Diagnosis and risk stratification in hypertrophic cardiomyopathy using machine learning wall thickness measurement: a comparison with human test-retest performance. Lancet Digit Health 2021;3:e20-8.
75. Eichhorn C, Greulich S, Bucciarelli-Ducci C, Sznitman R, Kwong RY, Gräni C. Multiparametric cardiovascular magnetic resonance approach in diagnosing, monitoring, and prognostication of myocarditis. JACC Cardiovasc Imag 2022;15:1325-38.
76. Fahmy AS, Rausch J, Neisius U, et al. Automated cardiac MR scar quantification in hypertrophic cardiomyopathy using deep convolutional neural networks. JACC Cardiovasc Imag 2018;11:1917-8.
77. Fahmy AS, El-Rewaidy H, Nezafat M, Nakamori S, Nezafat R. Automated analysis of cardiovascular magnetic resonance myocardial native T1 mapping images using fully convolutional neural networks. J Cardiovasc Magn Reson 2019;21:7.
78. Neisius U, El-Rewaidy H, Nakamori S, Rodriguez J, Manning WJ, Nezafat R. Radiomic analysis of myocardial native T1 imaging discriminates between hypertensive heart disease and hypertrophic cardiomyopathy. JACC Cardiovasc Imag 2019;12:1946-54.
79. Wang J, Yang F, Liu W, et al. Radiomic analysis of native T1 mapping images discriminates between MYH7 and MYBPC3-related hypertrophic cardiomyopathy. J Magn Reson Imag 2020;52:1714-21.
80. Xue H, Artico J, Davies RH, et al. Automated in-line artificial intelligence measured global longitudinal shortening and mitral annular plane systolic excursion: reproducibility and prognostic significance. J Am Heart Assoc 2022;11:e023849.
81. Coenen A, Kim YH, Kruk M, et al. Diagnostic accuracy of a machine-learning approach to coronary computed tomographic angiography-based fractional flow reserve: result from the MACHINE consortium. Circ Cardiovasc Imag 2018;11:e007217.
82. Kang D, Dey D, Slomka PJ, et al. Structured learning algorithm for detection of nonobstructive and obstructive coronary plaque lesions from computed tomography angiography. J Med Imag 2015;2:014003.
83. Liao S, Mo Z, Zeng M, et al. Fast and low-dose medical imaging generation empowered by hybrid deep-learning and iterative reconstruction. Cell Rep Med 2023;4:101119.
84. Motwani M, Dey D, Berman DS, et al. Machine learning for prediction of all-cause mortality in patients with suspected coronary artery disease: a 5-year multicentre prospective registry analysis. Eur Heart J 2017;38:500-7.
85. van Rosendael AR, Maliakal G, Kolli KK, et al. Maximization of the usage of coronary CTA derived plaque information using a machine learning based algorithm to improve risk stratification; insights from the CONFIRM registry. J Cardiovasc Comput Tomogr 2018;12:204-9.
86. Li T, Zhang M, Qi W, Asma E, Qi J. Motion correction of respiratory-gated PET images using deep learning based image registration framework. Phys Med Biol 2020;65:155003.
87. Nakajima K, Kudo T, Nakata T, et al. Diagnostic accuracy of an artificial neural network compared with statistical quantitation of myocardial perfusion images: a Japanese multicenter study. Eur J Nucl Med Mol Imag 2017;44:2280-9.
88. Arsanjani R, Xu Y, Dey D, et al. Improved accuracy of myocardial perfusion SPECT for detection of coronary artery disease by machine learning in a large population. J Nucl Cardiol 2013;20:553-62.
89. Betancur J, Commandeur F, Motlagh M, et al. Deep learning for prediction of obstructive disease from fast myocardial perfusion SPECT: a multicenter study. JACC Cardiovasc Imag 2018;11:1654-63.
90. Betancur J, Otaki Y, Motwani M, et al. Prognostic value of combined clinical and myocardial perfusion imaging data using machine learning. JACC Cardiovasc Imag 2018;11:1000-9.
91. Arsanjani R, Dey D, Khachatryan T, et al. Prediction of revascularization after myocardial perfusion SPECT by machine learning in a large population. J Nucl Cardiol 2015;22:877-84.
92. Virani SS, Alonso A, Benjamin EJ, et al. Heart disease and stroke statistics-2020 update: a report from the american heart association. Circulation 2020;141:e139-596.
93. Maragna R, Giacari CM, Guglielmo M, et al. Artificial intelligence based multimodality imaging: a new frontier in coronary artery disease management. Front Cardiovasc Med 2021;8:736223.
94. Acharya UR, Fujita H, Adam M, et al. Automated characterization and classification of coronary artery disease and myocardial infarction by decomposition of ECG signals: a comparative study. Inf Sci 2017;377:17-29.
95. Han C, Shi L. ML-ResNet: a novel network to detect and locate myocardial infarction using 12 leads ECG. Comput Meth Prog Bio 2020;185:105138.
96. Lih OS, Jahmunah V, San TR, et al. Comprehensive electrocardiographic diagnosis based on deep learning. Artif Intell Med 2020;103:101789.
97. McCarthy CP, Neumann JT, Michelhaugh SA, et al. Derivation and external validation of a high-sensitivity cardiac troponin-based proteomic model to predict the presence of obstructive coronary artery disease. J Am Heart Assoc 2020;9:e017221.
98. Sabatino J, De Rosa S, Leo I, et al. Non-invasive myocardial work is reduced during transient acute coronary occlusion. PLoS One 2020;15:e0244397.
99. Sabatino J, De Rosa S, Leo I, et al. Prediction of significant coronary artery disease through advanced echocardiography: role of non-invasive myocardial work. Front Cardiovasc Med 2021;8:719603.
100. Raghavendra U, En Wei JK, Gudigar A, et al. Automated diagnosis and assessment of cardiac structural alteration in hypertension ultrasound images. Contrast Media Mol Imag 2022;2022:5616939.
101. Vidya KS, Ng EYK, Acharya UR, Chou SM, Tan RS, Ghista DN. Computer-aided diagnosis of myocardial infarction using ultrasound images with DWT, GLCM and HOS methods: a comparative study. Comput Biol Med 2015;62:86-93.
102. Glessgen CG, Boulougouri M, Vallée JP, et al. Artificial intelligence-based opportunistic detection of coronary artery stenosis on aortic computed tomography angiography in emergency department patients with acute chest pain. Eur Heart J Open 2023;3:oead088.
103. Knott KD, Seraphim A, Augusto JB, et al. The prognostic significance of quantitative myocardial perfusion: an artificial intelligence-based approach using perfusion mapping. Circulation 2020;141:1282-91.
104. Farooq V, van Klaveren D, Steyerberg EW, et al. Anatomical and clinical characteristics to guide decision making between coronary artery bypass surgery and percutaneous coronary intervention for individual patients: development and validation of SYNTAX score II. Lancet 2013;381:639-50.
105. De Rosa S, Polimeni A, De Velli G, et al. Reliability of instantaneous wave-free ratio (iFR) for the evaluation of left main coronary artery lesions. J Clin Med 2019;8:1143.
106. De Rosa S, Polimeni A, Petraco R, Davies JE, Indolfi C. Diagnostic performance of the instantaneous wave-free ratio: comparison with fractional flow reserve. Circ Cardiovasc Interv 2018;11:e004613.
107. SCOT-HEART Investigators. Coronary CT angiography and 5-year risk of myocardial infarction. N Engl J Med 2018;379:924-33.
108. Douglas PS, De Bruyne B, Pontone G, et al. 1-year outcomes of FFRCT-guided care in patients with suspected coronary disease: the PLATFORM study. J Am Coll Cardiol 2016;68:435-45.
109. Tesche C, Gray HN. Machine learning and deep neural networks applications in coronary flow assessment: the case of computed tomography fractional flow reserve. J Thorac Imag 2020;35:S66-71.
110. Celeng C, Leiner T, Maurovich-Horvat P, et al. Anatomical and functional computed tomography for diagnosing hemodynamically significant coronary artery disease: a meta-analysis. JACC Cardiovasc Imag 2019;12:1316-25.
111. Qiao HY, Tang CX, Schoepf UJ, et al. Impact of machine learning-based coronary computed tomography angiography fractional flow reserve on treatment decisions and clinical outcomes in patients with suspected coronary artery disease. Eur Radiol 2020;30:5841-51.
112. Curzen N, Nicholas Z, Stuart B, et al. Fractional flow reserve derived from computed tomography coronary angiography in the assessment and management of stable chest pain: the FORECAST randomized trial. Eur Heart J 2021;42:3844-52.
113. Yang J, Shan D, Wang X, et al. On-site computed tomography-derived fractional flow reserve to guide management of patients with stable coronary artery disease: the TARGET randomized trial. Circulation 2023;147:1369-81.
114. Kumamaru KK, Fujimoto S, Otsuka Y, et al. Diagnostic accuracy of 3D deep-learning-based fully automated estimation of patient-level minimum fractional flow reserve from coronary computed tomography angiography. Eur Heart J Cardiovasc Imag 2020;21:437-45.
115. Min HS, Yoo JH, Kang SJ, et al. Detection of optical coherence tomography-defined thin-cap fibroatheroma in the coronary artery using deep learning. EuroIntervention 2020;16:404-12.
116. Cho H, Kang SJ, Min HS, et al. Intravascular ultrasound-based deep learning for plaque characterization in coronary artery disease. Atherosclerosis 2021;324:69-75.
117. Cook CM, Warisawa T, Howard JP, et al. Algorithmic versus expert human interpretation of instantaneous wave-free ratio coronary pressure-wire pull back data. JACC Cardiovasc Interv 2019;12:1315-24.
118. Miyoshi T, Higaki A, Kawakami H, Yamaguchi O. Automated interpretation of the coronary angioscopy with deep convolutional neural networks. Open Heart 2020;7:e001177.
119. Serruys PW, Chichareon P, Modolo R, et al. The SYNTAX score on its way out or … towards artificial intelligence: part I. EuroIntervention 2020;16:44-59.
120. Serruys PW, Chichareon P, Modolo R, et al. The SYNTAX score on its way out or … towards artificial intelligence: part II. EuroIntervention 2020;16:60-75.
121. Conrad N, Judge A, Tran J, et al. Temporal trends and patterns in heart failure incidence: a population-based study of 4 million individuals. Lancet 2018;391:572-80.
122. 2017 Disease and Injury Incidence and Prevalence Collaborators. Global, regional, and national incidence, prevalence, and years lived with disability for 354 diseases and injuries for 195 countries and territories, 1990-2017: a systematic analysis for the global burden of disease study 2017. Lancet 2018;392:1789-858.
125. Smeets M, Vaes B, Mamouris P, et al. Burden of heart failure in Flemish general practices: a registry-based study in the Intego database. BMJ Open 2019;9:e022972.
126. Gerber Y, Weston SA, Redfield MM, et al. A contemporary appraisal of the heart failure epidemic in Olmsted County, Minnesota, 2000 to 2010. JAMA Intern Med 2015;175:996-1004.
127. Boccuto F, De Rosa S, Torella D, Veltri P, Guzzi PH. Will artificial intelligence provide answers to current gaps and needs in chronic heart failure? Appl Sci 2023;13:7663.
128. Lindholm D, Fukaya E, Leeper NJ, Ingelsson E. Bioimpedance and new-onset heart failure: a longitudinal study of >500,000 individuals from the general population. J Am Heart Assoc 2018;7:e008970.
129. Yang G, Ren Y, Pan Q, et al. A heart failure diagnosis model based on support vector machine. In 2010 3rd international conference on biomedical engineering and informatics; 16-18 Oct 2010; Yantai, China.
130. Gharehchopogh SF, Khalifehlou ZA. Neural network application in diagnosis of patient: a case study. In International Conference on Computer Networks and Information Technology; 11-13 Jul 2011; Abbottabad, Pakistan.
131. Aljaaf AJ, Al-Jumeily D, Hussain AJ, Dawson T, Fergus P, Al-Jumaily M. Predicting the likelihood of heart failure with a multi level risk assessment using decision tree. In: 2015 third international conference on technological advances in electrical, electronics and computer engineering (TAEECE); 29 April 2015-01 May 2015; Beirut, Lebanon.
132. Zheng Y, Guo X, Qin J, Xiao S. Computer-assisted diagnosis for chronic heart failure by the analysis of their cardiac reserve and heart sound characteristics. Comput Meth Prog Bio 2015;122:372-83.
133. Gharehbaghi A, Lindén M, Babic A. An artificial intelligent-based model for detecting systolic pathological patterns of phonocardiogram based on time-growing neural network. Appl Soft Comput 2019;83:105615.
134. Segar MW, Vaduganathan M, Patel KV, et al. Machine learning to predict the risk of incident heart failure hospitalization among patients with diabetes: the WATCH-DM risk score. Diabetes Care 2019;42:2298-306.
135. Segar MW, Jaeger BC, Patel KV, et al. Development and validation of machine learning-based race-specific models to predict 10-year risk of heart failure: a multicohort analysis. Circulation 2021;143:2370-83.
136. Morrill J, Qirko K, Kelly J, et al. A machine learning methodology for identification and triage of heart failure exacerbations. J Cardiovasc Transl Res 2022;15:103-15.
137. Shah SJ, Katz DH, Selvaraj S, et al. Phenomapping for novel classification of heart failure with preserved ejection fraction. Circulation 2015;131:269-79.
138. Kao DP, Lewsey JD, Anand IS, et al. Characterization of subgroups of heart failure patients with preserved ejection fraction with possible implications for prognosis and treatment response. Eur J Heart Fail 2015;17:925-35.
139. Gu J, Pan JA, Lin H, Zhang JF, Wang CQ. Characteristics, prognosis and treatment response in distinct phenogroups of heart failure with preserved ejection fraction. Int J Cardiol 2021;323:148-54.
140. Segar MW, Patel KV, Ayers C, et al. Phenomapping of patients with heart failure with preserved ejection fraction using machine learning-based unsupervised cluster analysis. Eur J Heart Fail 2020;22:148-58.
141. Hedman ÅK, Hage C, Sharma A, et al. Identification of novel pheno-groups in heart failure with preserved ejection fraction using machine learning. Heart 2020;106:342-9.
142. Schrub F, Oger E, Bidaut A, et al. Heart failure with preserved ejection fraction: a clustering approach to a heterogenous syndrome. Arch Cardiovasc Dis 2020;113:381-90.
143. Gevaert AB, Tibebu S, Mamas MA, et al. Clinical phenogroups are more effective than left ventricular ejection fraction categories in stratifying heart failure outcomes. ESC Heart Fail 2021;8:2741-54.
144. Kwon JM, Kim KH, Jeon KH, et al. Artificial intelligence algorithm for predicting mortality of patients with acute heart failure. PLoS One 2019;14:e0219302.
145. Peng S, Huang J, Liu X, et al. Interpretable machine learning for 28-day all-cause in-hospital mortality prediction in critically ill patients with heart failure combined with hypertension: a retrospective cohort study based on medical information mart for intensive care database-IV and eICU databases. Front Cardiovasc Med 2022;9:994359.
146. Li J, Liu S, Hu Y, Zhu L, Mao Y, Liu J. Predicting mortality in intensive care unit patients with heart failure using an interpretable machine learning model: retrospective cohort study. J Med Internet Res 2022;24:e38082.
147. Yang B, Zhu Y, Lu X, Shen C. A Novel composite indicator of predicting mortality risk for heart failure patients with diabetes admitted to intensive care unit based on machine learning. Front Endocrinol 2022;13:917838.
148. Karwath A, Bunting KV, Gill SK, et al. Redefining β-blocker response in heart failure patients with sinus rhythm and atrial fibrillation: a machine learning cluster analysis. Lancet 2021;398:1427-35.
149. Cikes M, Sanchez-Martinez S, Claggett B, et al. Machine learning-based phenogrouping in heart failure to identify responders to cardiac resynchronization therapy. Eur J Heart Fail 2019;21:74-85.
150. Zheng Y, Lu X, Georgescu B, et al. Robust object detection using marginal space learning and ranking-based multi-detector aggregation: application to left ventricle detection in 2D MRI images. In: 2009 IEEE conference on computer vision and pattern recognition; 20-25 Jun 2009; Miami, FL, USA.
151. Lu X, Jolly MP, Georgescu B, et al. Automatic view planning for cardiac MRI acquisition. In: Fichtinger G, Martel A, Peters T, editors. Medical image computing and computer-assisted intervention - MICCAI 2011. Berlin, Heidelberg: Springer; 2011. pp. 479-86.
152. Avendi MR, Kheradvar A, Jafarkhani H. A combined deep-learning and deformable-model approach to fully automatic segmentation of the left ventricle in cardiac MRI. Med Image Anal 2016;30:108-19.
153. Orwat S, Arvanitaki A, Diller GP. A new approach to modelling in adult congenital heart disease: artificial intelligence. Rev Esp Cardiol 2021;74:573-5.
154. Hyun CM, Kim HP, Lee SM, et al. Deep learning for undersampled MRI reconstruction. Phys Med Biol 2018;63:135007.
155. Pace DF, Dalca AV, Geva T, Powell AJ, Moghari MH, Golland P. Interactive whole-heart segmentation in congenital heart disease. In: Navab N, Hornegger J, Wells WM, Frangi AF, editors. Medical Image computing and computer-assisted intervention - MICCAI 2015. Cham: Springer International Publishing; 2015. pp. 80-8.
156. Arafati A, Hu P, Finn JP, et al. Artificial intelligence in pediatric and adult congenital cardiac MRI: an unmet clinical need. Cardiovasc Diagn Ther 2019;9:S310-25.
157. Diller GP, Vahle J, Radke R, et al. Utility of deep learning networks for the generation of artificial cardiac magnetic resonance images in congenital heart disease. BMC Med Imag 2020;20:113.
158. Pace DF, Dalca AV, Brosch T, et al. Iterative segmentation from limited training data: applications to congenital heart disease. In: Stoyanov D, Taylor Z, Carneiro G, et al. editors. Deep learning in medical image analysis and multimodal learning for clinical decision support. Cham: Springer International Publishing; 2018. pp. 334-42.
159. Wesołowski S, Lemmon G, Hernandez EJ, et al. An explainable artificial intelligence approach for predicting cardiovascular outcomes using electronic health records. PLOS Digit Health 2022;1:e0000004.
160. Diller GP, Kempny A, Babu-Narayan SV, et al. Machine learning algorithms estimating prognosis and guiding therapy in adult congenital heart disease: data from a single tertiary centre including 10019 patients. Eur Heart J 2019;40:1069-77.
161. Diller GP, Orwat S, Vahle J, et al. Prediction of prognosis in patients with tetralogy of Fallot based on deep learning imaging analysis. Heart 2020;106:1007-14.
162. Davies R, Babu-Narayan SV. Deep learning in congenital heart disease imaging: hope but not haste. Heart 2020;106:960-1.
163. Diller GP, Lammers AE, Babu-Narayan S, et al. Denoising and artefact removal for transthoracic echocardiographic imaging in congenital heart disease: utility of diagnosis specific deep learning algorithms. Int J Cardiovasc Imag 2019;35:2189-96.
164. Chessa M, Van De Bruaene A, Farooqi K, et al. Three-dimensional printing, holograms, computational modelling, and artificial intelligence for adult congenital heart disease care: an exciting future. Eur Heart J 2022;43:2672-84.
165. Lu XH, Liu A, Fuh SC, et al. Recurrent disease progression networks for modelling risk trajectory of heart failure. PLoS One 2021;16:e0245177.
166. Ishikita A, McIntosh C, Hanneman K, et al. Machine learning for prediction of adverse cardiovascular events in adults with repaired tetralogy of fallot using clinical and cardiovascular magnetic resonance imaging variables. Circ Cardiovasc Imag 2023;16:e015205.
167. Chang Junior J, Binuesa F, Caneo LF, et al. Improving preoperative risk-of-death prediction in surgery congenital heart defects using artificial intelligence model: a pilot study. PLoS One 2020;15:e0238199.
168. Bogani R, Theodorou A, Arnaboldi L, Wortham RH. Garbage in, toxic data out: a proposal for ethical artificial intelligence sustainability impact statements. AI Ethics 2022:1-8.
169. Liyanage H, Liaw ST, Jonnagaddala J, et al. Artificial intelligence in primary health care: perceptions, issues, and challenges. Yearb Med Inform 2019;28:41-6.
170. Kim DW, Jang HY, Kim KW, Shin Y, Park SH. Design characteristics of studies reporting the performance of artificial intelligence algorithms for diagnostic analysis of medical images: results from recently published papers. Korean J Radiol 2019;20:405-10.
171. Tsopra R, Fernandez X, Luchinat C, et al. A framework for validating AI in precision medicine: considerations from the European ITFoC consortium. BMC Med Inform Decis Mak 2021;21:274.
172. Ghasemi M, Samadi M, Soleimanian E, Chau KW. A comparative study of black-box and white-box data-driven methods to predict landfill leachate permeability. Environ Monit Assess 2023;195:862.
173. Mey O, Neufeld D. Explainable AI algorithms for vibration data-based fault detection: use case-adadpted methods and critical evaluation. Sensors 2022;22:9037.
174. Noseworthy PA, Attia ZI, Brewer LC, et al. Assessing and mitigating bias in medical artificial intelligence: the effects of race and ethnicity on a deep learning model for ECG analysis. Circ Arrhythm Electrocardiol 2020;13:e007988.
175. De Rosa S, Brida M, Grapsa J, Dos Subira L, Bäck M, Chieffo A. Women in cardiology. Eur Heart J Open 2023;3:oead122.
176. Abrams C. Google’s effort to prevent blindness shows AI challenges. Wall Street J 2019;1:26. Available from: https://www.wsj.com/articles/googles-effort-to-prevent-blindness-hits-roadblock-11548504004 [Last accessed on 25 Apr 2024].
177. Hardesty L. Study finds gender and skin-type bias in commercial artificial-intelligence systems. 2018. Available from: https://news.mit.edu/2018/study-finds-gender-skin-type-bias-artificial-intelligence-systems-0212 [Last accessed on 5 Mar 2024].
Cite This Article
Export citation file: BibTeX | EndNote | RIS
OAE Style
Strangio A, Leo I, Sabatino J, Brida M, Siracusa C, Carabetta N, Zaffino P, Critelli C, Laschera A, Spadea MF, Torella D, Sabouret P, De Rosa S. Is artificial intelligence the new kid on the block? Sustainable applications in cardiology. Vessel Plus 2024;8:12. http://dx.doi.org/10.20517/2574-1209.2023.123
AMA Style
Strangio A, Leo I, Sabatino J, Brida M, Siracusa C, Carabetta N, Zaffino P, Critelli C, Laschera A, Spadea MF, Torella D, Sabouret P, De Rosa S. Is artificial intelligence the new kid on the block? Sustainable applications in cardiology. Vessel Plus. 2024; 8: 12. http://dx.doi.org/10.20517/2574-1209.2023.123
Chicago/Turabian Style
Antonio Strangio, Isabella Leo, Jolanda Sabatino, Margarita Brida, Chiara Siracusa, Nicole Carabetta, Paolo Zaffino, Claudia Critelli, Alessandro Laschera, Maria Francesca Spadea, Daniele Torella, Pierre Sabouret, Salvatore De Rosa. 2024. "Is artificial intelligence the new kid on the block? Sustainable applications in cardiology" Vessel Plus. 8: 12. http://dx.doi.org/10.20517/2574-1209.2023.123
ACS Style
Strangio, A.; Leo I.; Sabatino J.; Brida M.; Siracusa C.; Carabetta N.; Zaffino P.; Critelli C.; Laschera A.; Spadea MF.; Torella D.; Sabouret P.; De Rosa S. Is artificial intelligence the new kid on the block? Sustainable applications in cardiology. Vessel Plus. 2024, 8, 12. http://dx.doi.org/10.20517/2574-1209.2023.123
About This Article
Special Issue
Copyright
Data & Comments
Data
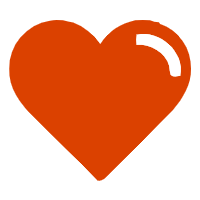
Comments
Comments must be written in English. Spam, offensive content, impersonation, and private information will not be permitted. If any comment is reported and identified as inappropriate content by OAE staff, the comment will be removed without notice. If you have any queries or need any help, please contact us at support@oaepublish.com.